Uprightnet: Geometry-Aware Camera Orientation Estimation From Single Images
2019 IEEE/CVF INTERNATIONAL CONFERENCE ON COMPUTER VISION (ICCV 2019)(2019)
摘要
We introduce UprightNet, a learning-based approach for estimating 2DoF camera orientation from a single RGB image of an indoor scene. Unlike recent methods that leverage deep learning to perform black-box regression from image to orientation parameters, we propose an end-to-end framework that incorporates explicit geometric reasoning. In particular, we design a network that predicts two representations of scene geometry, in both the local camera and global reference coordinate systems, and solves for the camera orientation as the rotation that best aligns these two predictions via a differentiable least squares module. This network can be trained end-to-end, and can be supervised with both ground truth camera poses and intermediate representations of surface geometry. We evaluate UprightNet on the single-image camera orientation task on synthetic and real datasets, and show significant improvements over prior state-of-the-art approaches.
更多查看译文
关键词
scene geometry,local camera,aligns these two predictions,ground truth camera,surface geometry,UprightNet,single-image camera orientation task,geometry-aware camera orientation estimation,learning-based approach,2DoF camera orientation,single RGB image,indoor scene,black-box regression,orientation parameters,end-to-end framework,explicit geometric reasoning
AI 理解论文
溯源树
样例
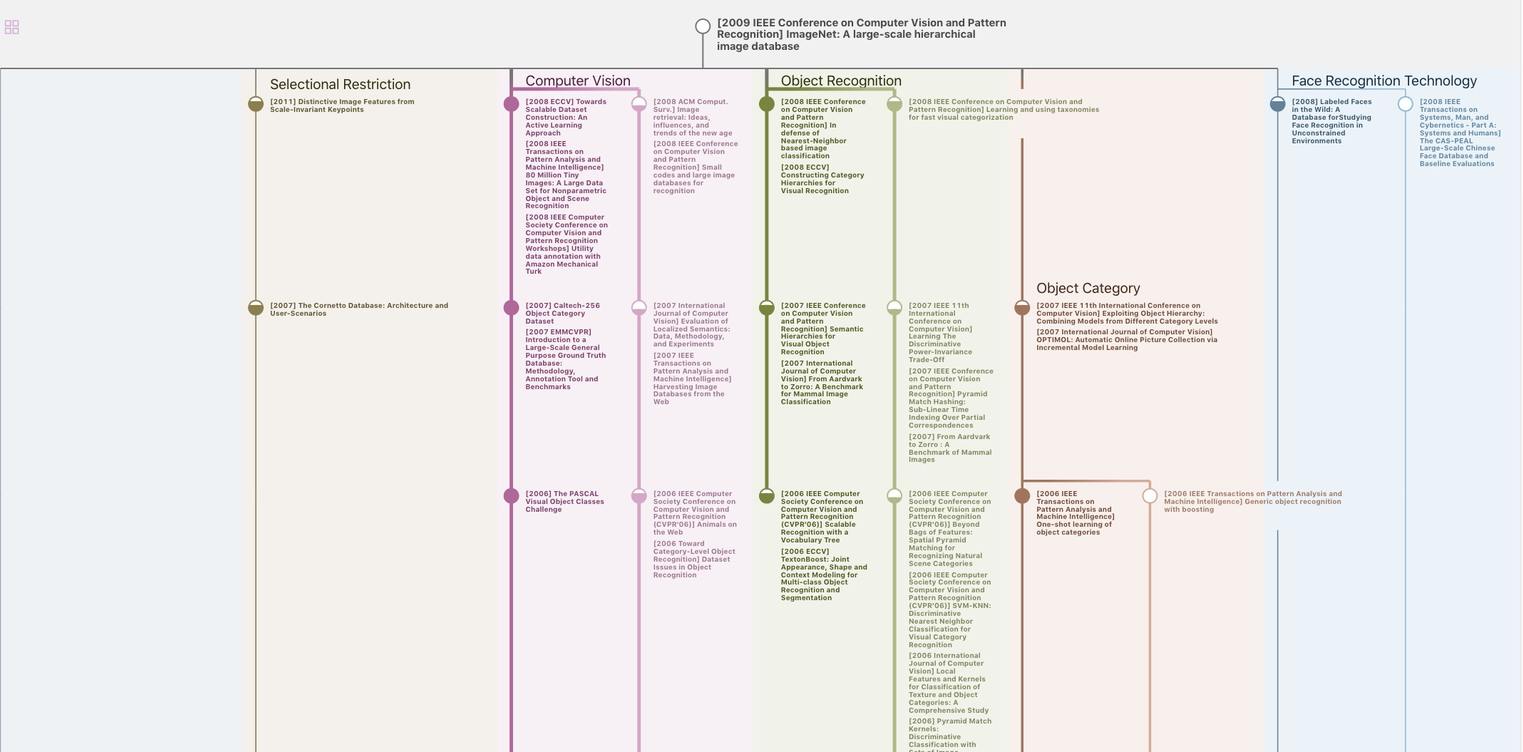
生成溯源树,研究论文发展脉络
Chat Paper
正在生成论文摘要