DAGMapper: Learning to Map by Discovering Lane Topology
2019 IEEE/CVF INTERNATIONAL CONFERENCE ON COMPUTER VISION (ICCV 2019)(2019)
摘要
One of the fundamental challenges to scale self-driving is being able to create accurate high definition maps (HD maps) with low cost. Current attempts to automate this process typically focus on simple scenarios, estimate independent maps per frame or do not have the level of precision required by modern self driving vehicles. In contrast, in this paper we focus on drawing the lane boundaries of complex highways with many lanes that contain topology changes due to forks and merges. Towards this goal, we formulate the problem as inference in a directed acyclic graphical model (DAG), where the nodes of the graph encode geometric and topological properties of the local regions of the lane boundaries. Since we do not know a priori the topology of the lanes, we also infer the DAG topology (i.e., nodes and edges) for each region. We demonstrate the effectiveness of our approach on two major North American Highways in two different states and show high precision and recall as well as 89% correct topology.
更多查看译文
关键词
DAGMapper,geometric properties,North American Highways,DAG topology,local regions,topological properties,directed acyclic graphical model,merges,forks,topology changes,complex highways,lane boundaries,self driving vehicles,HD maps,accurate high definition maps,lane topology
AI 理解论文
溯源树
样例
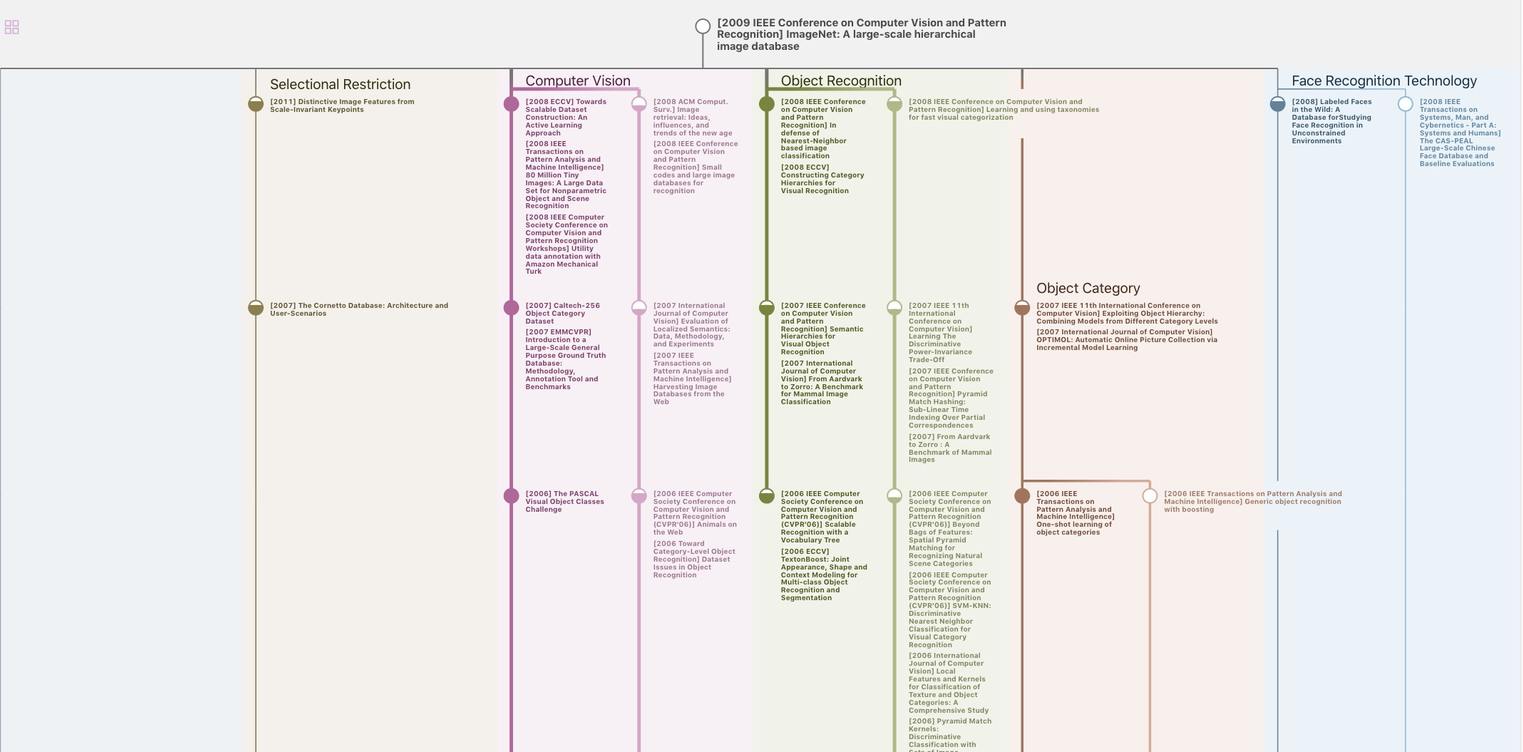
生成溯源树,研究论文发展脉络
Chat Paper
正在生成论文摘要