Gaussian Affinity For Max-Margin Class Imbalanced Learning
2019 IEEE/CVF INTERNATIONAL CONFERENCE ON COMPUTER VISION (ICCV 2019)(2019)
摘要
Real-world object classes appear in imbalanced ratios. This poses a significant challenge for classifiers which get biased towards frequent classes. We hypothesize that improving the generalization capability of a classifier should improve learning on imbalanced datasets. Here, we introduce the first hybrid loss function that jointly performs classification and clustering in a single formulation. Our approach is based on an 'affinity measure' in Euclidean space that leads to the following benefits: (1) direct enforcement of maximum margin constraints on classification boundaries, (2) a tractable way to ensure uniformly spaced and equidistant cluster centers, (3) flexibility to learn multiple class prototypes to support diversity and discriminability in feature space. Our extensive experiments demonstrate the significant performance improvements on multiple imbalanced datasets belonging to visual classification and verification tasks. The proposed loss can easily be plugged in any deep architecture as a differentiable block and demonstrates robustness against different levels of data imbalance and corrupted labels.
更多查看译文
关键词
max-margin class,real-world object classes,imbalanced ratios,classifier,hybrid loss function,clustering,affinity measure,Euclidean space,maximum margin constraints,classification boundaries,equidistant cluster centers,discriminability,feature space,visual classification,verification tasks,imbalanced datasets,Gaussian affinity,imbalanced learning
AI 理解论文
溯源树
样例
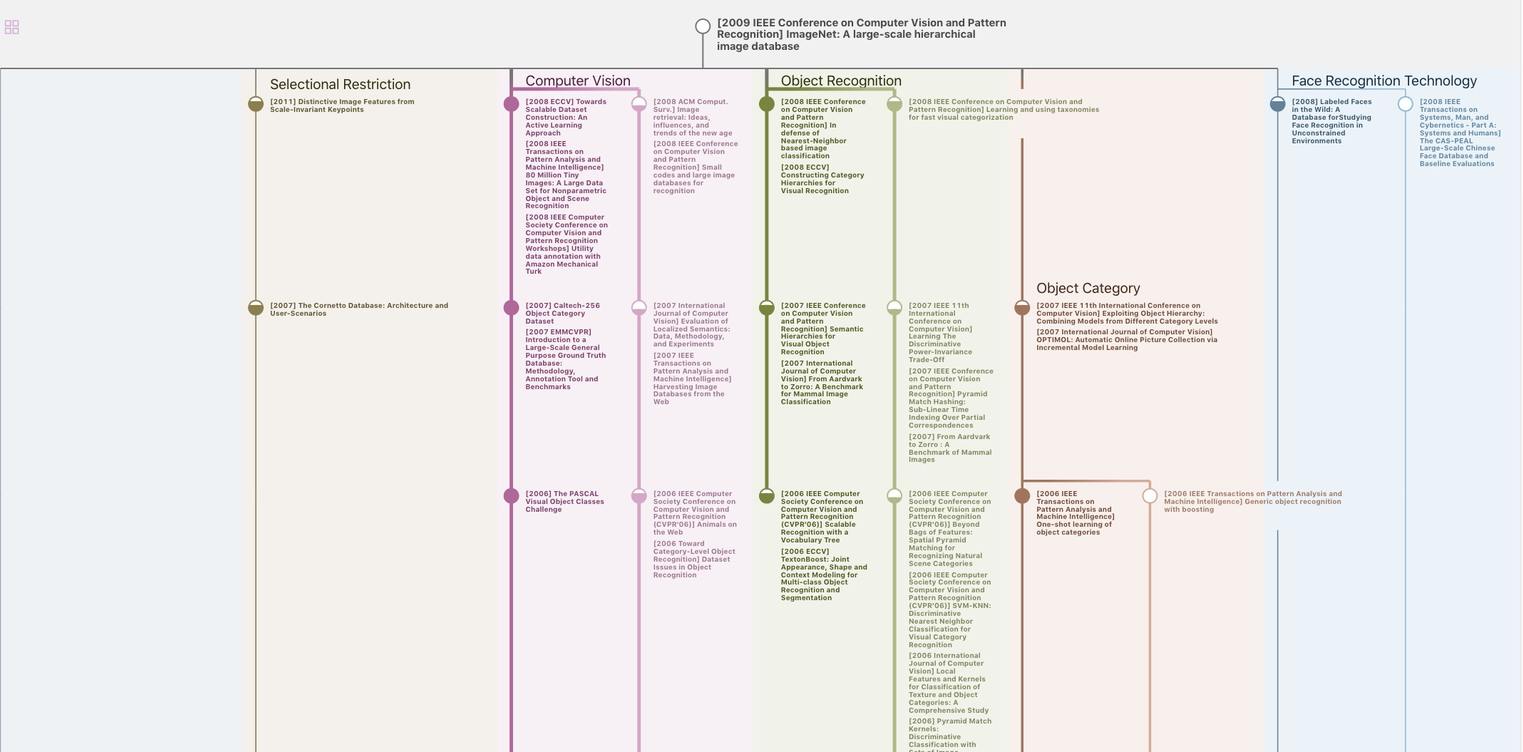
生成溯源树,研究论文发展脉络
Chat Paper
正在生成论文摘要