Exploiting Spatial-Temporal Relationships For 3d Pose Estimation Via Graph Convolutional Networks
2019 IEEE/CVF INTERNATIONAL CONFERENCE ON COMPUTER VISION (ICCV 2019)(2019)
摘要
Despite great progress in 3D pose estimation from single-view images or videos, it remains a challenging task due to the substantial depth ambiguity and severe self-occlusions. Motivated by the effectiveness of incorporating spatial dependencies and temporal consistencies to alleviate these issues, we propose a novel graph-based method to tackle the problem of 3D human body and 3D hand pose estimation from a short sequence of 2D joint detections. Particularly, domain knowledge about the human hand (body) configurations is explicitly incorporated into the graph convolutional operations to meet the specific demand of the 3D pose estimation. Furthermore, we introduce a local-to-global network architecture, which is capable of learning multi-scale features for the graph-based representations. We evaluate the proposed method on challenging benchmark datasets for both 3D hand pose estimation and 3D body pose estimation. Experimental results show that our method achieves state-of-the-art performance on both tasks.
更多查看译文
关键词
3d pose estimation,graph convolutional networks,spatial-temporal
AI 理解论文
溯源树
样例
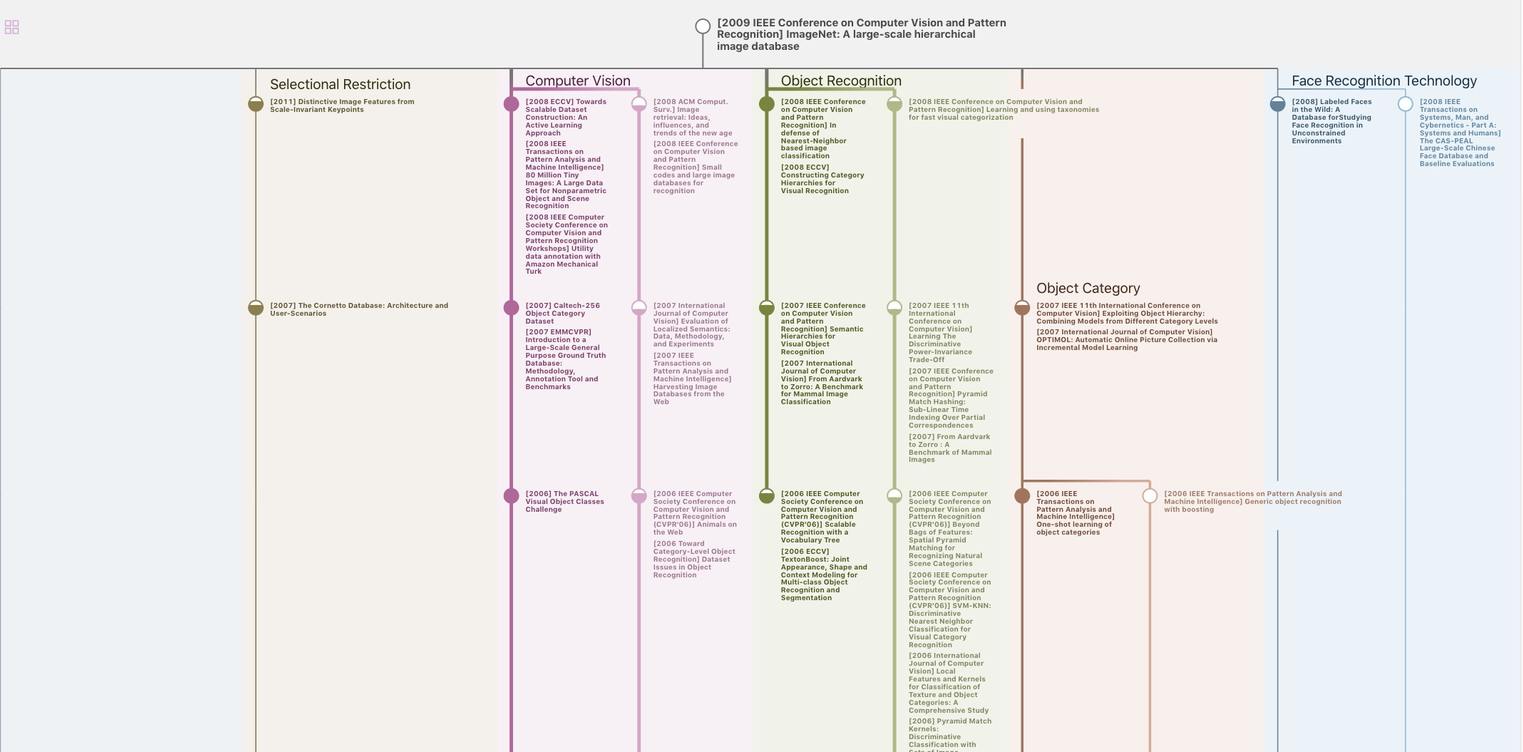
生成溯源树,研究论文发展脉络
Chat Paper
正在生成论文摘要