Fingerprinting-Based Indoor Localization with Commercial mmWave WiFi - Part II: Spatial Beam SNRs
IEEE Global Communications Conference(2019)
摘要
Existing fingerprint-based indoor localization uses either fine-grained channel state information (CSI) from the physical layer or coarse-grained received signal strength indicator (RSSI) measurements from the MAC layer. In this paper, we propose to use an intermediate channel measurement - spatial beam signal-to-noise ratios (SNRs) that are inherently available during the beam training phase as defined in the IEEE 802.11ad standard - to construct the feature space for location-and-orientation-dependent fingerprinting database. We build a 60GHz experimental platform consisting of three access points and one client using commercial-off-the-shelf routers and collect realworld beam SNR measurements in an office environment during regular office hours. Both position/orientation classification and coordinate estimation are considered using classic machine learning approaches. Comprehensive performance evaluation using real-world beam SNRs demonstrates that the classification accuracy is 99.8% if the location is only interested, while the accuracy is 98.6% for simultaneous position-and-orientations classification. Direct coordinate estimation gives an average root-mean-square error of 17.52 cm and 95% of all coordinate estimates are less than 26.90 cm away from corresponding true locations. This concept directly applies to other mmWave band (e.g., 5G) devices where beam training is also required.
更多查看译文
关键词
location-and-orientation-dependent fingerprinting database,intermediate channel measurement,RSSI measurements,received signal strength indicator measurements,CSI,mmWave WiFi band,spatial beam SNR measurements,performance evaluation,machine learning approaches,office environment,routers,IEEE 802.11ad standard,beam training phase,beam signal-to-noise ratios,MAC layer,fine-grained channel state information,fingerprint-based indoor localization,frequency 60.0 GHz
AI 理解论文
溯源树
样例
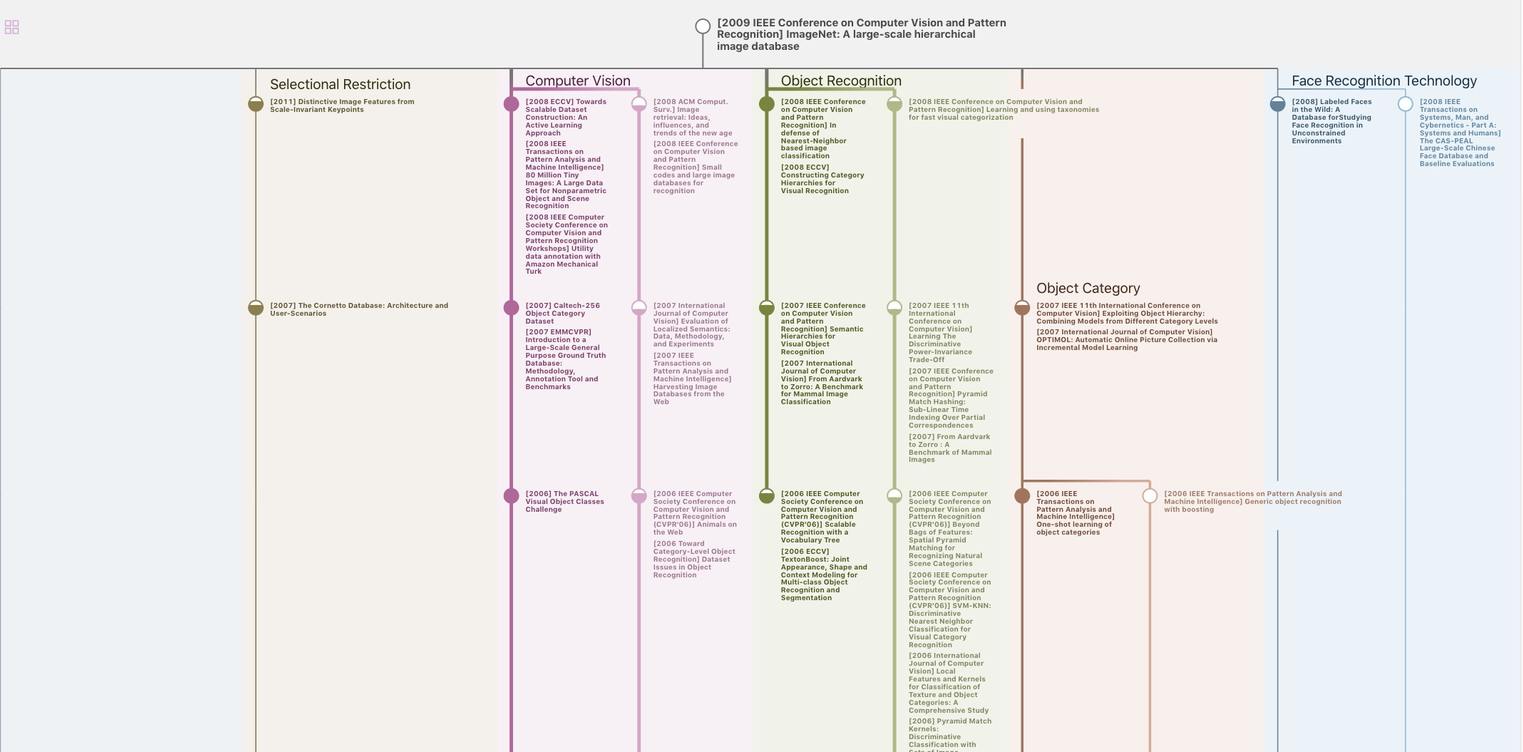
生成溯源树,研究论文发展脉络
Chat Paper
正在生成论文摘要