A Long Short-Term Memory Enabled Framework for DDoS Detection
IEEE Global Communications Conference(2019)
摘要
The proliferation of attack-for-hire services, coupled with the advent of Internet of Things (IoT)-enabled botnets, is driving the increase of the frequency and intensity of Distributed Denial of Services (DDoS) attacks, at an alarming rate. Inspired by the success of machine learning in a variety of fields and domain applications, numerous intelligent schemes have been proposed to effectively defend against and mitigate the impact of these attacks. Traditional machine learning methods, however, are limited by the use of an expensive and error-prone feature engineering process. Feature engineering is fundamental to the application of machine learning, and is both difficult and expensive. Furthermore, the ability of these schemes to successfully detect previously unknown attacks is limited. To address these limitations, a novel DDoS detection scheme, based on Long Short-Term Memory (LSTM), is proposed. The basic tenet of the LSTM scheme is its ability to distinguish between attack and legitimate flows by only examining a relatively small number of a network flow packets. The performance evaluation results show that the LSTM-based scheme successfully learns the complex flow-level feature representations embedded in raw input traffic. Furthermore, the results show that the scheme performs better than other approaches that use sophisticated flow-level statistical features. Lastly, the results show the ability of the proposed scheme to accurately capture the dynamic behaviors of unknown network traffic exceeds that of traditional machine learning methods.
更多查看译文
关键词
DDoS Detection,Deep Learning,LSTM
AI 理解论文
溯源树
样例
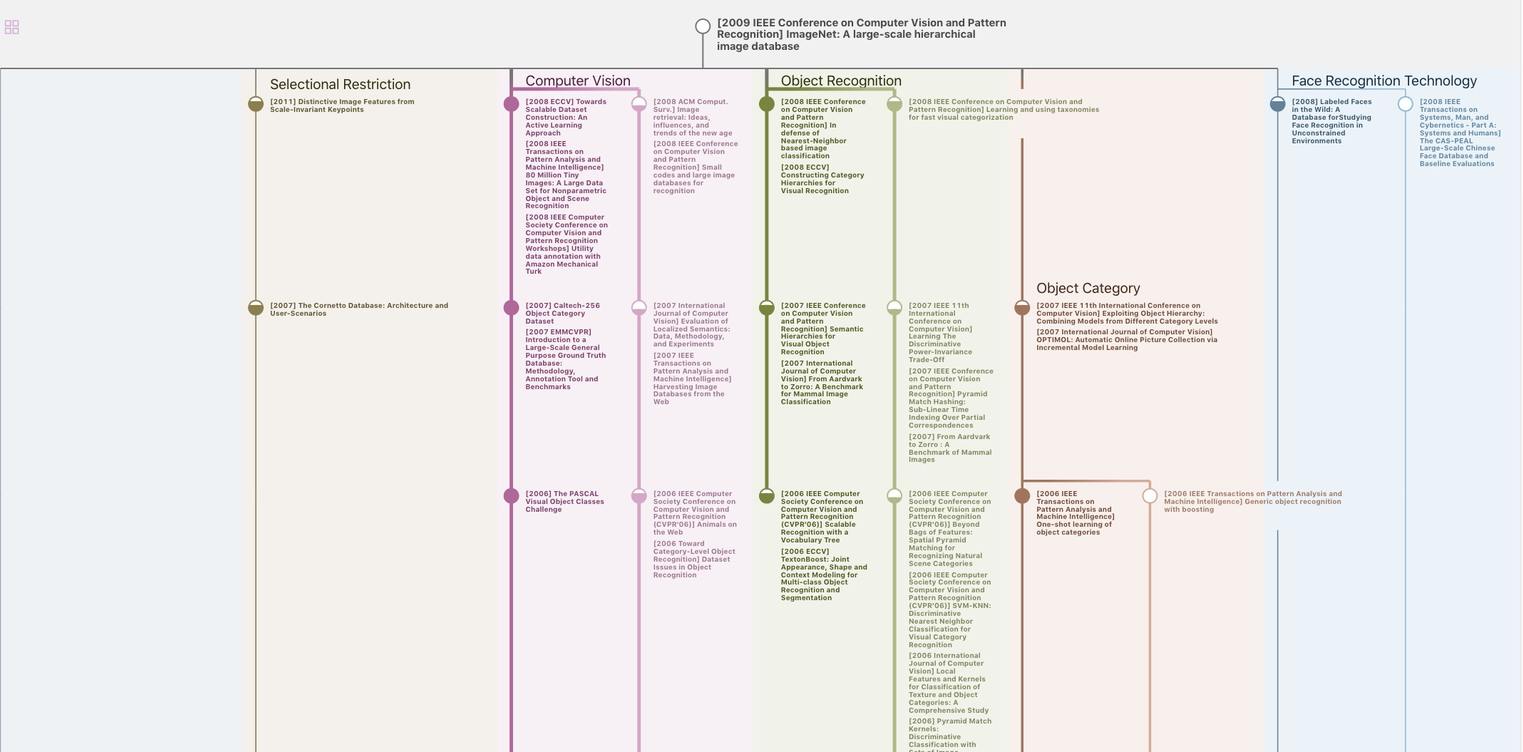
生成溯源树,研究论文发展脉络
Chat Paper
正在生成论文摘要