Leveraging Neural Caption Translation With Visually Grounded Paraphrase Augmentation
IEICE TRANSACTIONS ON INFORMATION AND SYSTEMS(2020)
摘要
Since a concept can be represented by different vocabularies, styles, and levels of detail, a translation task resembles a many-to-many mapping task from a distribution of sentences in the source language into a distribution of sentences in the target language. This viewpoint, however, is not fully implemented in current neural machine translation (NMT), which is one-to-one sentence mapping. In this study, we represent the distribution itself as multiple paraphrase sentences, which will enrich the model context understanding and trigger it to produce numerous hypotheses. We use a visually grounded paraphrase (VGP), which uses images as a constraint of the concept in paraphrasing, to guarantee that the created paraphrases are within the intended distribution. In this way, our method can also be considered as incorporating image information into NMT without using the image itself. We implement this idea by crowdsourcing a paraphrasing corpus that realizes VGP and construct neural paraphrasing that behaves as expert models in a NMT. Our experimental results reveal that our proposed VGP augmentation strategies showed improvement against a vanilla NMT baseline.
更多查看译文
关键词
visually grounded paraphrase, data augmentation, neural machine translation
AI 理解论文
溯源树
样例
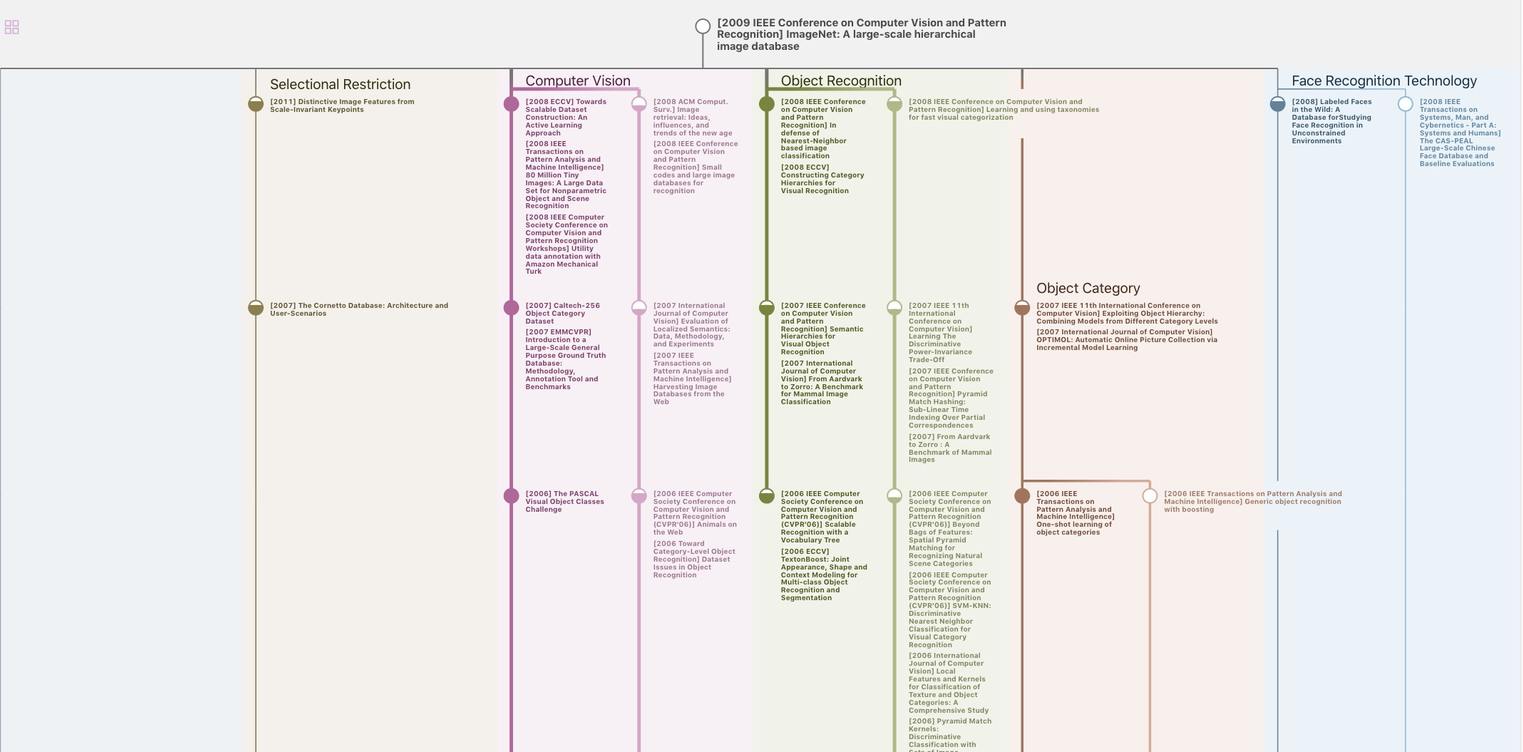
生成溯源树,研究论文发展脉络
Chat Paper
正在生成论文摘要