Markov chain Monte Carlo with neural network surrogates: application to contaminant source identification
STOCHASTIC ENVIRONMENTAL RESEARCH AND RISK ASSESSMENT(2020)
摘要
Subsurface remediation often involves reconstruction of contaminant release history from sparse observations of solute concentration. Markov Chain Monte Carlo (MCMC), the most accurate and general method for this task, is rarely used in practice because of its high computational cost associated with multiple solves of contaminant transport equations. We propose an adaptive MCMC method, in which a transport model is replaced with a fast and accurate surrogate model in the form of a deep convolutional neural network (CNN). The CNN-based surrogate is trained on a small number of the transport model runs based on the prior knowledge of the unknown release history. Thus reduced computational cost allows one to diminish the sampling error associated with construction of the approximate likelihood function. As all MCMC strategies for source identification, our method has an added advantage of quantifying predictive uncertainty and accounting for measurement errors. Our numerical experiments demonstrate the accuracy comparable to that of MCMC with the forward transport model, which is obtained at a fraction of the computational cost of the latter.
更多查看译文
关键词
MCMC,CNN,Surrogate model,Source identification
AI 理解论文
溯源树
样例
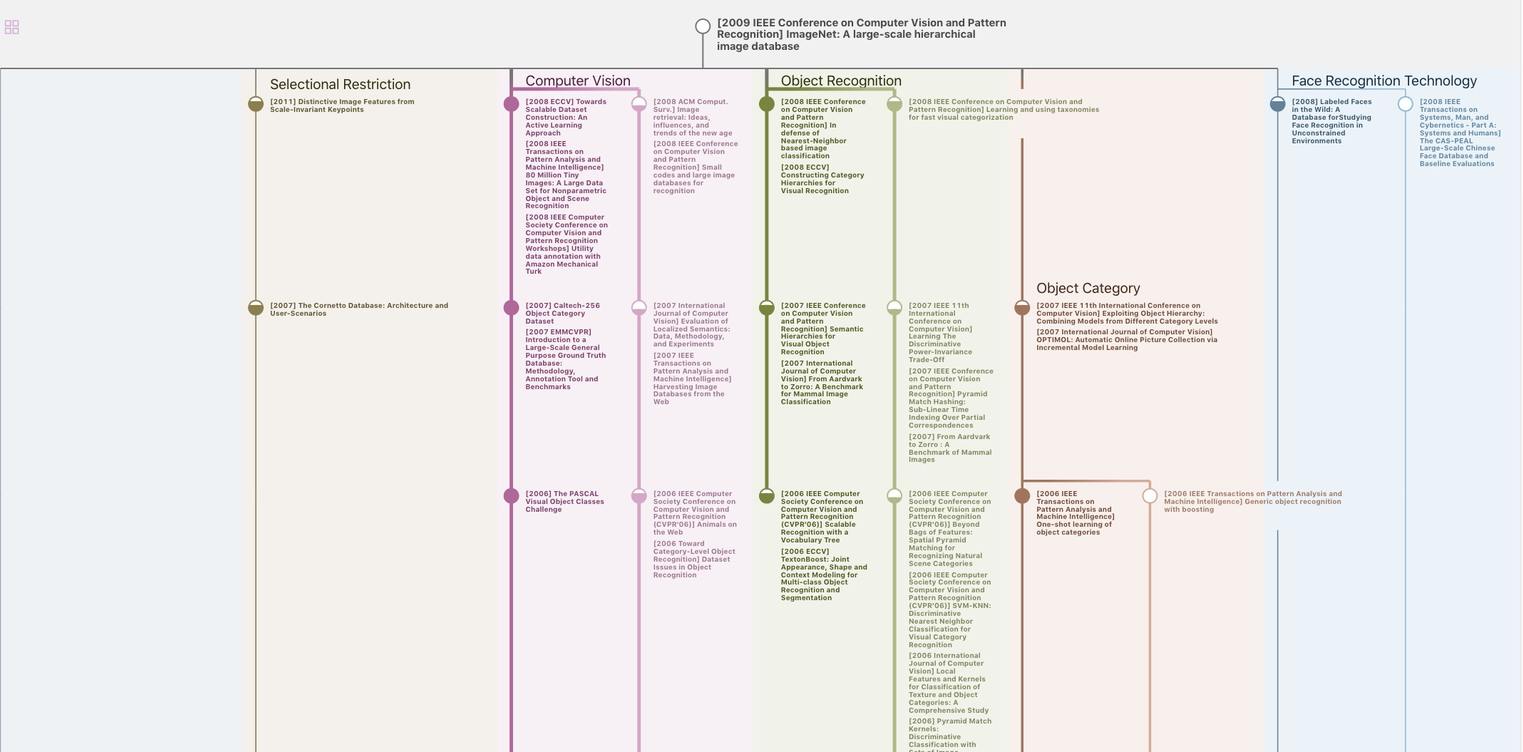
生成溯源树,研究论文发展脉络
Chat Paper
正在生成论文摘要