Fast Algorithms For Joint Multicast Beamforming And Antenna Selection In Massive Mimo
IEEE TRANSACTIONS ON SIGNAL PROCESSING(2020)
摘要
Massive MIMO is currently a leading physical layer technology candidate that can dramatically enhance throughput in 5G systems, for both unicast and multicast transmission modalities. As antenna elements are becoming smaller and cheaper in the mmW range compared to radio frequency (RF) chains, it is crucial to perform antenna selection at the transmitter, such that the available RF chains are switched to an appropriate subset of antennas. This paper considers the joint problem of multicast beamforming and antenna selection for a single multicast group in massive MIMO systems. The prior state-of-art for this problem relies on semi-definite relaxation (SDR), which cannot scale up to the massive MIMO regime. A successive convex approximation (SCA) based approach is proposed to tackle max-min fair joint multicast beamforming and antenna selection. The key idea of SCA is to successively approximate the non-convex problem by a class of non-smooth, convex optimization problems. Two fast and memory efficient first-order methods are proposed to solve each SCA subproblem. Simulations demonstrate that the proposed algorithms outperform the existing state-of-art approach in terms of solution quality and run time, in both traditional and especially in massive MIMO settings.
更多查看译文
关键词
MIMO communication, Array signal processing, Signal processing algorithms, Transmitting antennas, Approximation algorithms, Multicast communication, Transmit beamforming, antenna selection, multicasting, multi-user massive multiple-input multiple-output (MIMO), group sparsity, non-convex optimization, first-order methods, convergence
AI 理解论文
溯源树
样例
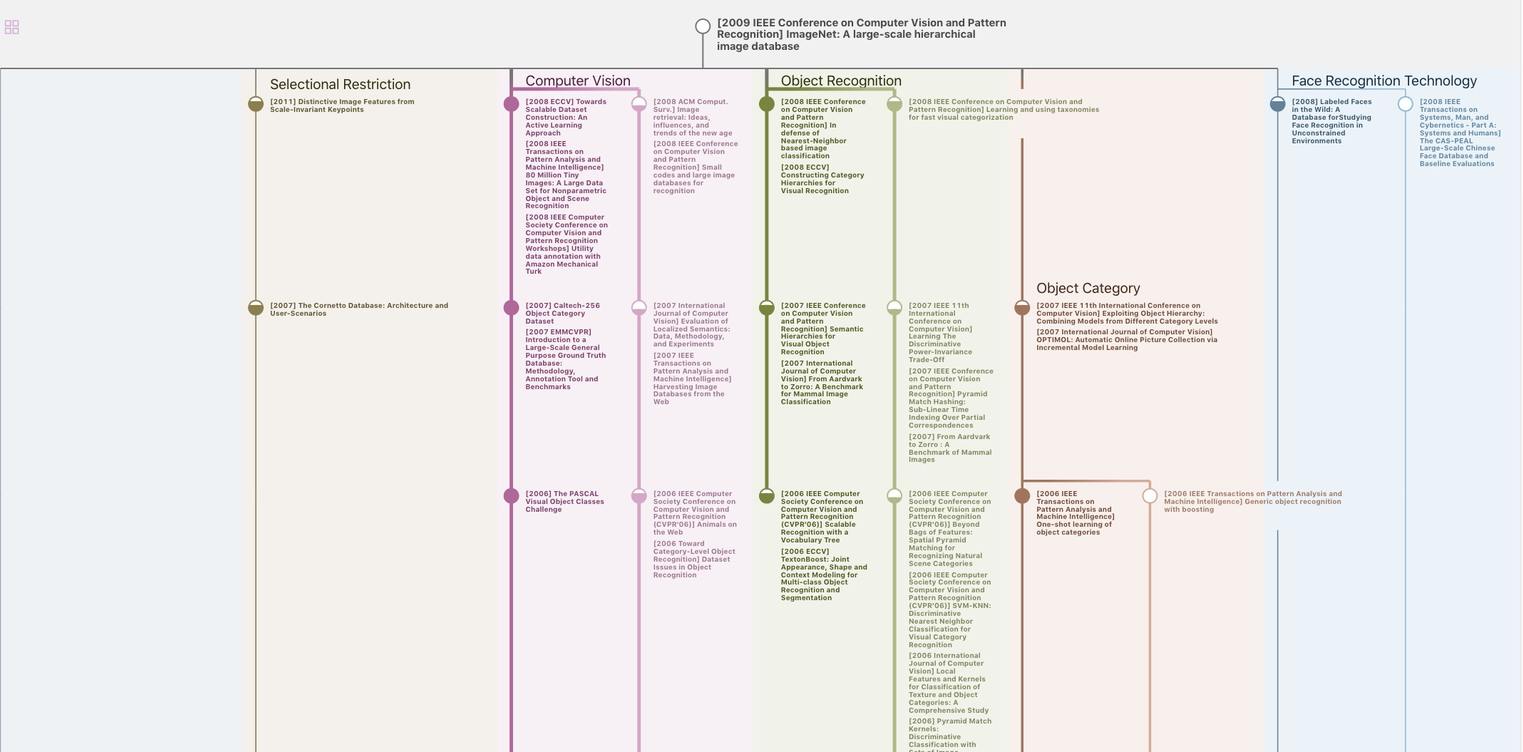
生成溯源树,研究论文发展脉络
Chat Paper
正在生成论文摘要