Automatic Hyper-Parameter Optimization Based on Mapping Discovery from Data to Hyper-Parameters
arxiv(2020)
摘要
Machine learning algorithms have made remarkable achievements in the field of artificial intelligence. However, most machine learning algorithms are sensitive to the hyper-parameters. Manually optimizing the hyper-parameters is a common method of hyper-parameter tuning. However, it is costly and empirically dependent. Automatic hyper-parameter optimization (autoHPO) is favored due to its effectiveness. However, current autoHPO methods are usually only effective for a certain type of problems, and the time cost is high. In this paper, we propose an efficient automatic parameter optimization approach, which is based on the mapping from data to the corresponding hyper-parameters. To describe such mapping, we propose a sophisticated network structure. To obtain such mapping, we develop effective network constrution algorithms. We also design strategy to optimize the result futher during the application of the mapping. Extensive experimental results demonstrate that the proposed approaches outperform the state-of-the-art apporaches significantly.
更多查看译文
关键词
mapping discovery,hyper-parameters
AI 理解论文
溯源树
样例
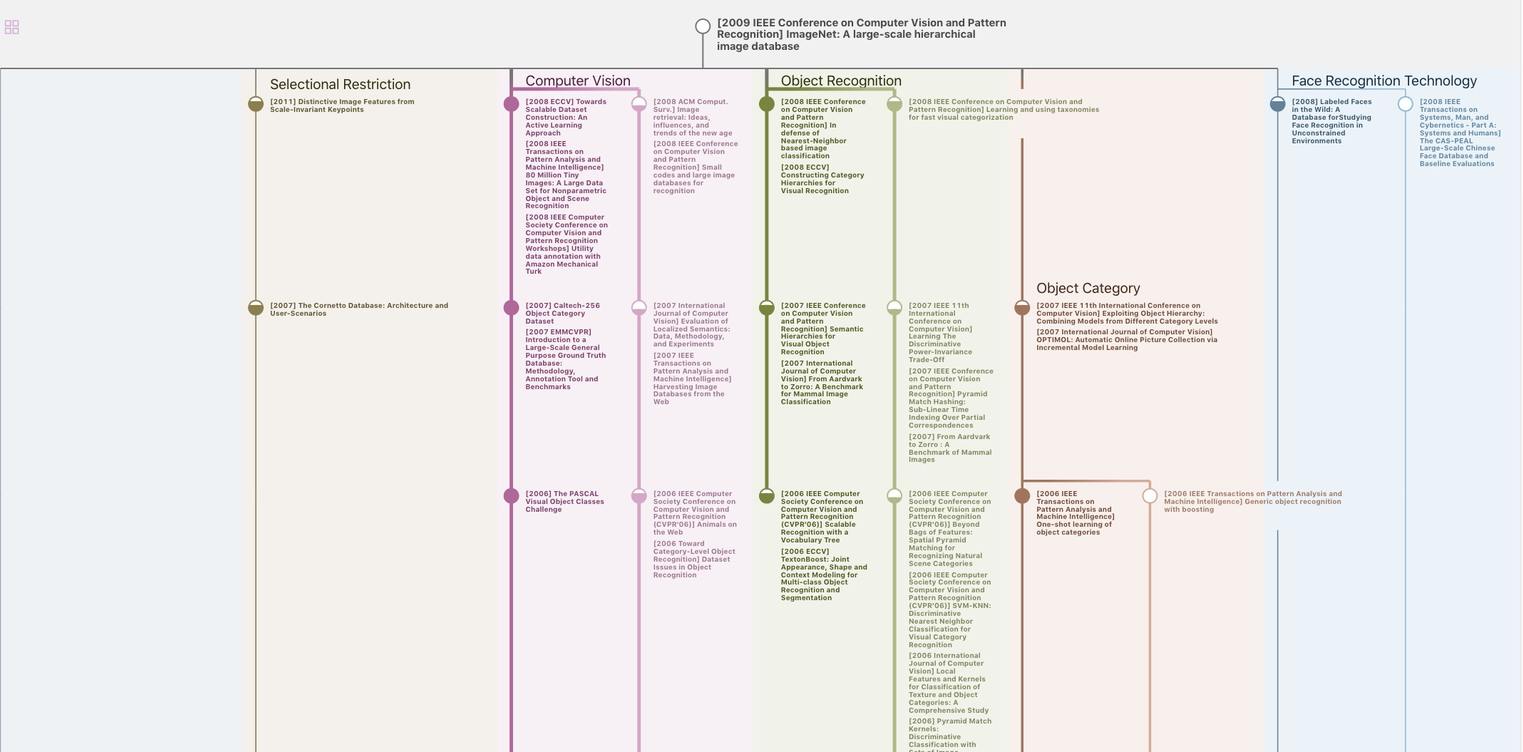
生成溯源树,研究论文发展脉络
Chat Paper
正在生成论文摘要