Multi-channel residual network model for accurate estimation of spatially-varying and depth-dependent defocus kernels.
OPTICS EXPRESS(2020)
摘要
Digital projectors have been increasingly utilized in various commercial and scientific applications. However, they are prone to the out-of-focus blurring problem since their depth-of-fields are typically limited. In this paper, we explore the feasibility of utilizing a deep learning-based approach to analyze the spatially-varying and depth-dependent defocus properties of digital projectors. A multimodal displaying/imaging system is built for capturing images projected at various depths. Based on the constructed dataset containing well-aligned in-focus, out-of-focus, and depth images, we propose a novel multi-channel residual deep network model to learn the end-to-end mapping function between the in-focus and out-of-focus image patches captured at different spatial locations and depths. To the best of our knowledge, it is the first research work revealing that the complex spatially-varying and depth-dependent blurring effects can be accurately learned from a number of real-captured image pairs instead of being hand-crafted as before. Experimental results demonstrate that our proposed deep learning-based method significantly outperforms the state-of-the-art defocus kernel estimation techniques and thus leads to better out-of-focus compensation for extending the dynamic ranges of digital projectors. (C) 2020 Optical Society of America under the terms of the OSA Open Access Publishing Agreement
更多查看译文
关键词
accurate estimation,kernels,multi-channel,spatially-varying,depth-dependent
AI 理解论文
溯源树
样例
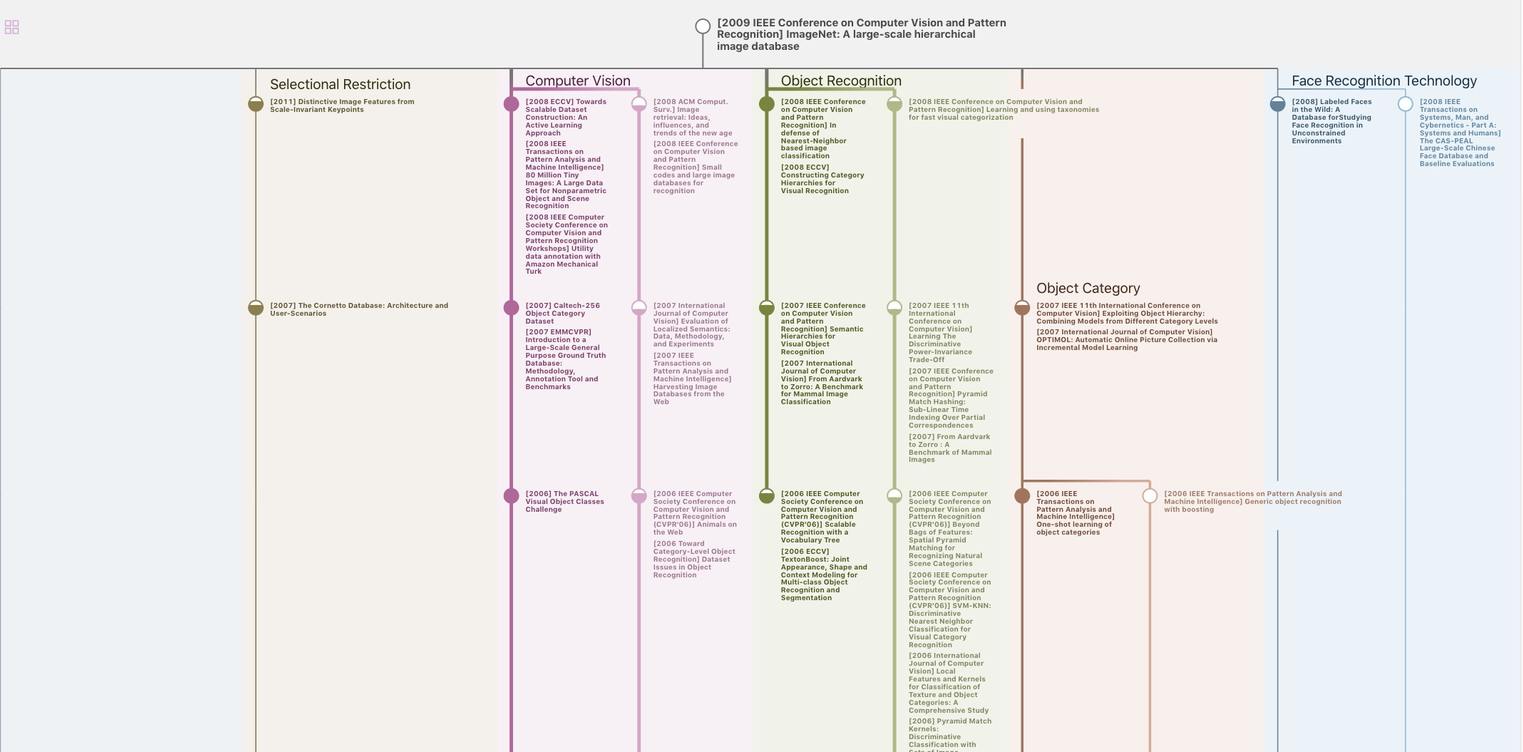
生成溯源树,研究论文发展脉络
Chat Paper
正在生成论文摘要