A Novel Image Classification Approach via Dense-MobileNet Models.
MOBILE INFORMATION SYSTEMS(2020)
摘要
As a lightweight deep neural network, MobileNet has fewer parameters and higher classification accuracy. In order to further reduce the number of network parameters and improve the classification accuracy, dense blocks that are proposed in DenseNets are introduced into MobileNet. In Dense-MobileNet models, convolution layers with the same size of input feature maps in MobileNet models are taken as dense blocks, and dense connections are carried out within the dense blocks. The new network structure can make full use of the output feature maps generated by the previous convolution layers in dense blocks, so as to generate a large number of feature maps with fewer convolution cores and repeatedly use the features. By setting a small growth rate, the network further reduces the parameters and the computation cost. Two Dense-MobileNet models, Dense1-MobileNet and Dense2-MobileNet, are designed. Experiments show that Dense2-MobileNet can achieve higher recognition accuracy than MobileNet, while only with fewer parameters and computation cost.
更多查看译文
关键词
novel image classification approach,models,dense-mobilenet
AI 理解论文
溯源树
样例
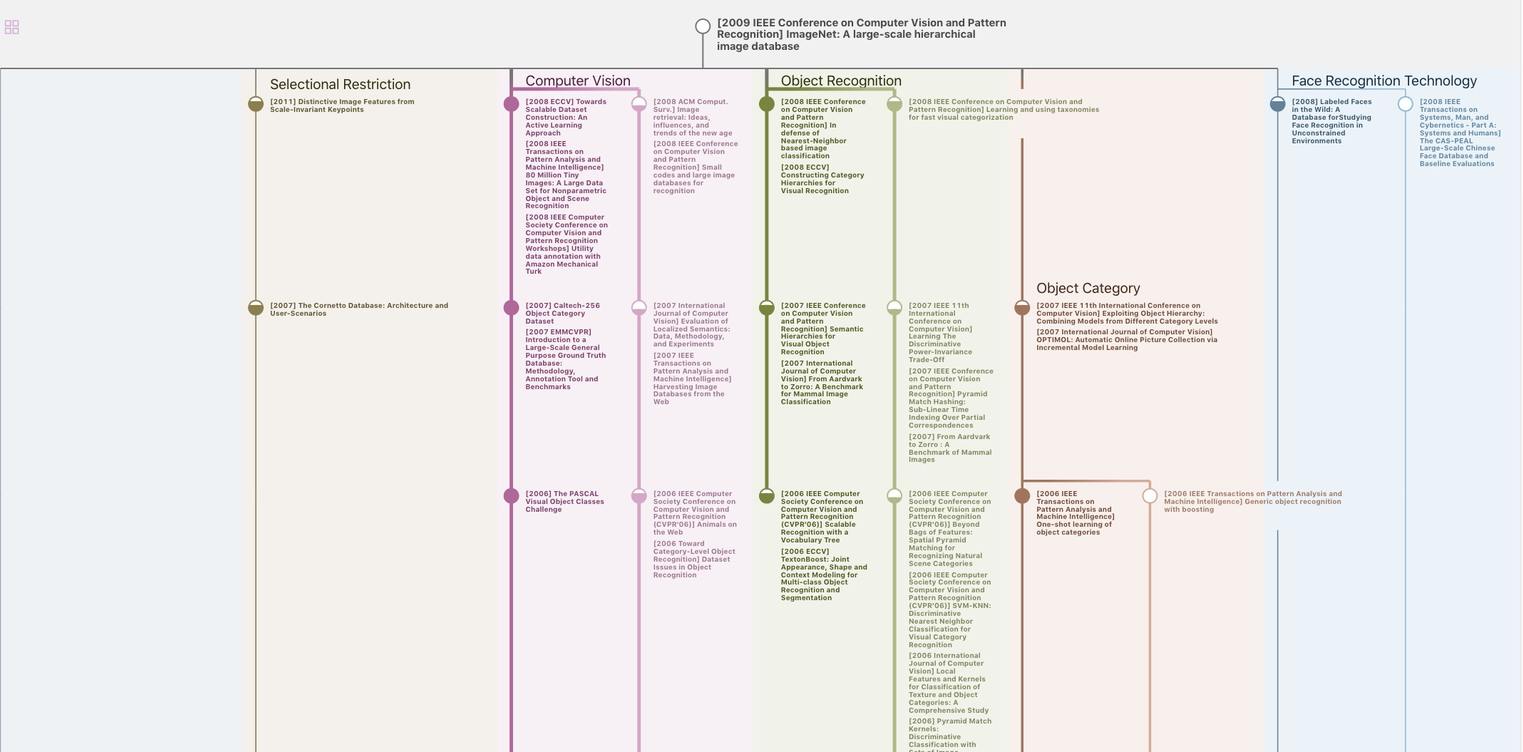
生成溯源树,研究论文发展脉络
Chat Paper
正在生成论文摘要