Identification of primary and collateral tracks in stuttered speech
LREC(2020)
摘要
Disfluent speech has been previously addressed from two main perspectives: the clinical perspective focusing on diagnostic, and the Natural Language Processing (NLP) perspective aiming at modeling these events and detect them for downstream tasks. In addition, previous works often used different metrics depending on whether the input features are text or speech, making it difficult to compare the different contributions. Here, we introduce a new evaluation framework for disfluency detection inspired by the clinical and NLP perspective together with the theory of performance from \cite{clark1996using} which distinguishes between primary and collateral tracks. We introduce a novel forced-aligned disfluency dataset from a corpus of semi-directed interviews, and present baseline results directly comparing the performance of text-based features (word and span information) and speech-based (acoustic-prosodic information). Finally, we introduce new audio features inspired by the word-based span features. We show experimentally that using these features outperformed the baselines for speech-based predictions on the present dataset.
更多查看译文
关键词
evaluation metrics, disfluency, stuttering, speech processing, audio features
AI 理解论文
溯源树
样例
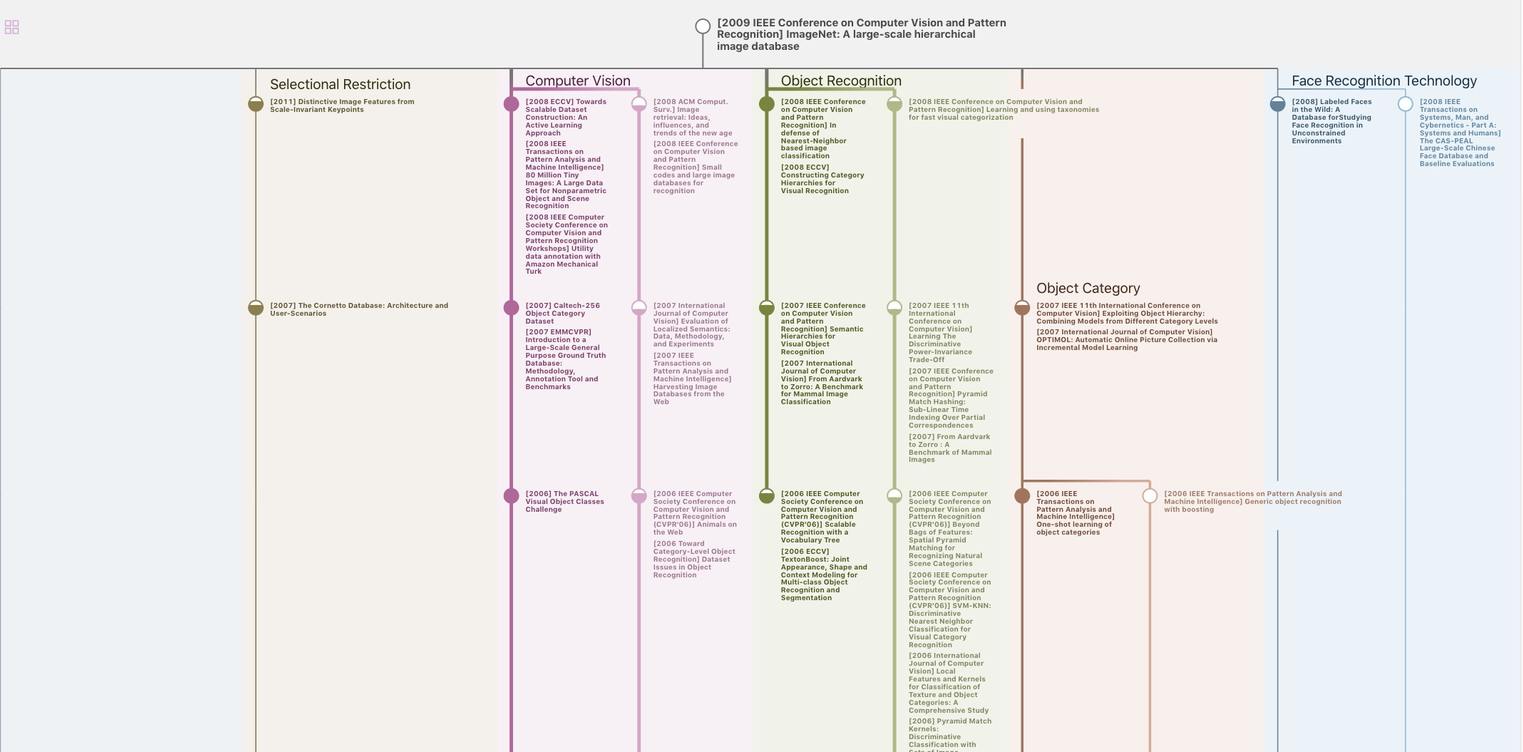
生成溯源树,研究论文发展脉络
Chat Paper
正在生成论文摘要