Gsanet: Semantic Segmentation With Global And Selective Attention
2020 IEEE INTERNATIONAL CONFERENCE ON IMAGE PROCESSING (ICIP)(2020)
摘要
This paper proposes a novel deep learning architecture for semantic segmentation. The proposed Global and Selective Attention Network (GSANet) features Atrous Spatial Pyramid Pooling (ASPP) with a novel sparsemax global attention and a novel selective attention that deploys a condensation and diffusion mechanism to aggregate the multi-scale contextual information from the extracted deep features. A selective attention decoder is also proposed to process the GSA-ASPP outputs for optimizing the softmax volume. We are the first to benchmark the performance of semantic segmentation networks with the low-complexity feature extraction network (FXN) MobileNetEdge, that is optimized for low latency on edge devices. We show that GSANet can result in more accurate segmentation with MobileNetEdge, as well as with strong FXNs, such as Xception. GSANet improves the state-of-art semantic segmentation accuracy on both the ADE20k and the Cityscapes datasets.
更多查看译文
关键词
GSANet, Selective Attention ASPP, Global Attention Feature, sparsemax
AI 理解论文
溯源树
样例
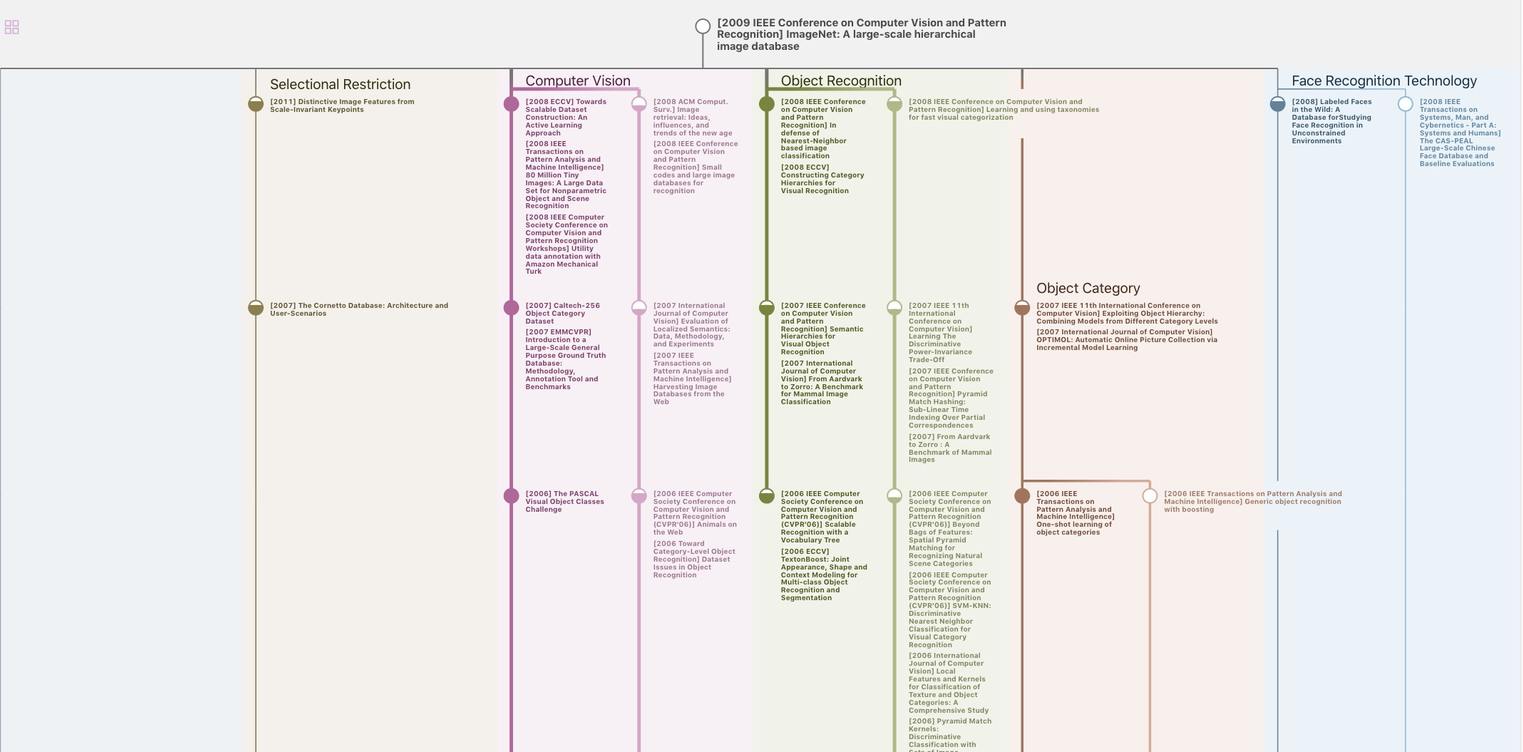
生成溯源树,研究论文发展脉络
Chat Paper
正在生成论文摘要