Can Primal Methods Outperform Primal-Dual Methods In Decentralized Dynamic Optimization?
IEEE TRANSACTIONS ON SIGNAL PROCESSING(2020)
摘要
In this paper, we consider the decentralized dynamic optimization problem defined over a multi-agent network. Each agent possesses a time-varying local objective function, and all agents aim to collaboratively track the drifting global optimal solution that minimizes the summation of all local objective functions. The decentralized dynamic optimization problem can be solved by primal or primal-dual methods, and when the problem degenerates to be static, it has been proved in literature that primal-dual methods are superior to primal ones. This motivates us to ask: are primal-dual methods necessarily better than primal ones in decentralized dynamic optimization? To answer this question, we investigate, and compare convergence properties of the primal method, diffusion, and the primal-dual approach, decentralized gradient tracking (DGT). Theoretical analysis reveals that diffusion can outperform DGT in certain dynamic settings. We find that DGT, and diffusion are significantly affected by the drifts, and the magnitudes of optimal gradients, respectively. In addition, we show that DGT is more sensitive to the network topology, and a badly-connected network can greatly deteriorate its convergence performance. These conclusions provide guidelines on how to choose proper dynamic algorithms in various application scenarios. Numerical experiments are constructed to validate the theoretical analysis.
更多查看译文
关键词
Optimization, Heuristic algorithms, Network topology, Steady-state, Convergence, Linear programming, Signal processing algorithms, Decentralized dynamic optimization, diffusion, decentralized gradient tracking (DGT)
AI 理解论文
溯源树
样例
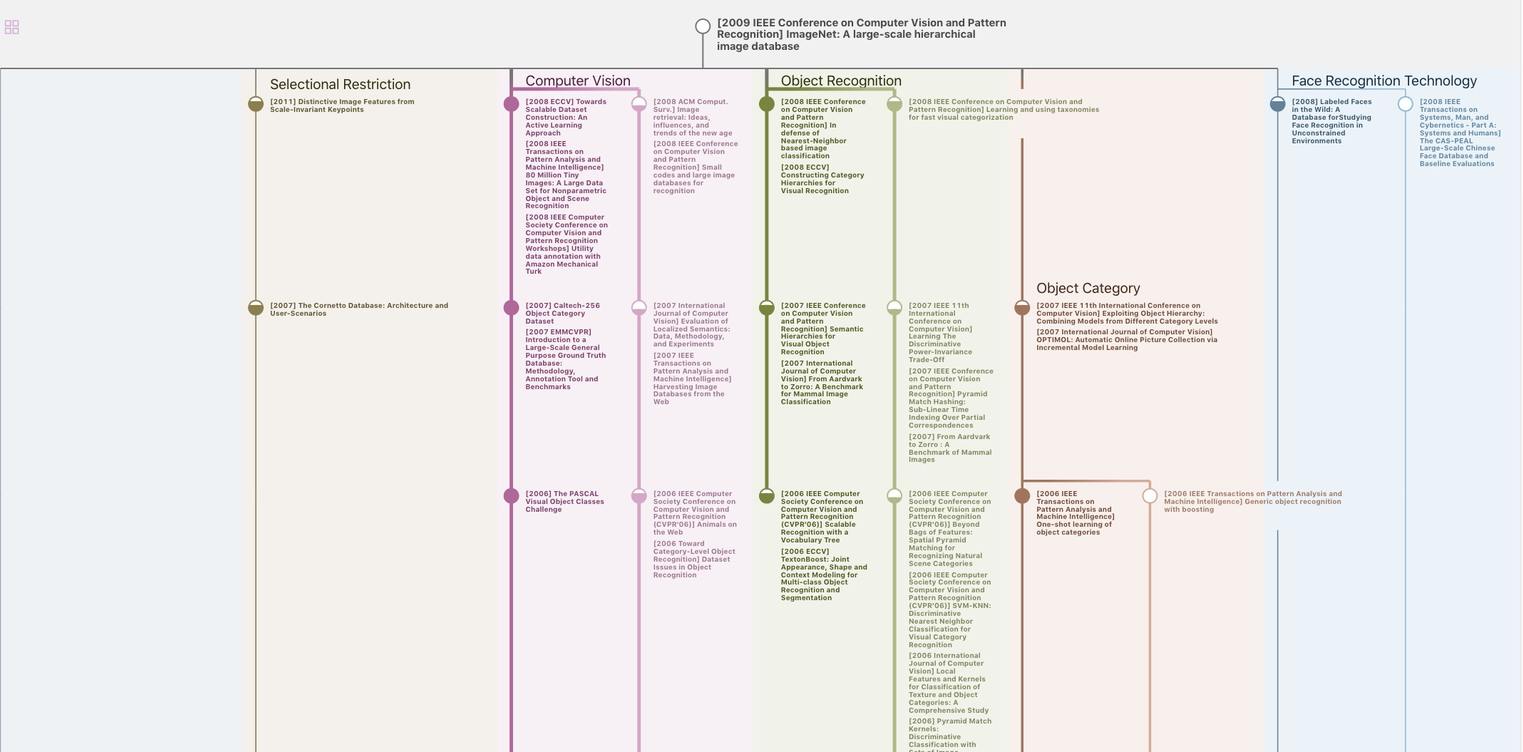
生成溯源树,研究论文发展脉络
Chat Paper
正在生成论文摘要