PF-Net: Point Fractal Network for 3D Point Cloud Completion
CVPR(2020)
摘要
In this paper, we propose a Point Fractal Network (PF-Net), a novel learning-based approach for precise and high-fidelity point cloud completion. Unlike existing point cloud completion networks, which generate the overall shape of the point cloud from the incomplete point cloud and always change existing points and encounter noise and geometrical loss, PF-Net preserves the spatial arrangements of the incomplete point cloud and can figure out the detailed geometrical structure of the missing region(s) in the prediction. To succeed at this task, PF-Net estimates the missing point cloud hierarchically by utilizing a feature-points-based multi-scale generating network. Further, we add up multi-stage completion loss and adversarial loss to generate more realistic missing region(s). The adversarial loss can better tackle multiple modes in the prediction. Our experiments demonstrate the effectiveness of our method for several challenging point cloud completion tasks.
更多查看译文
关键词
point fractal network,point cloud completion tasks,adversarial loss,multistage completion loss,feature-points-based multiscale generating network,missing point cloud,PF-Net estimates,incomplete point cloud,point cloud completion networks,high-fidelity point cloud completion,learning-based approach
AI 理解论文
溯源树
样例
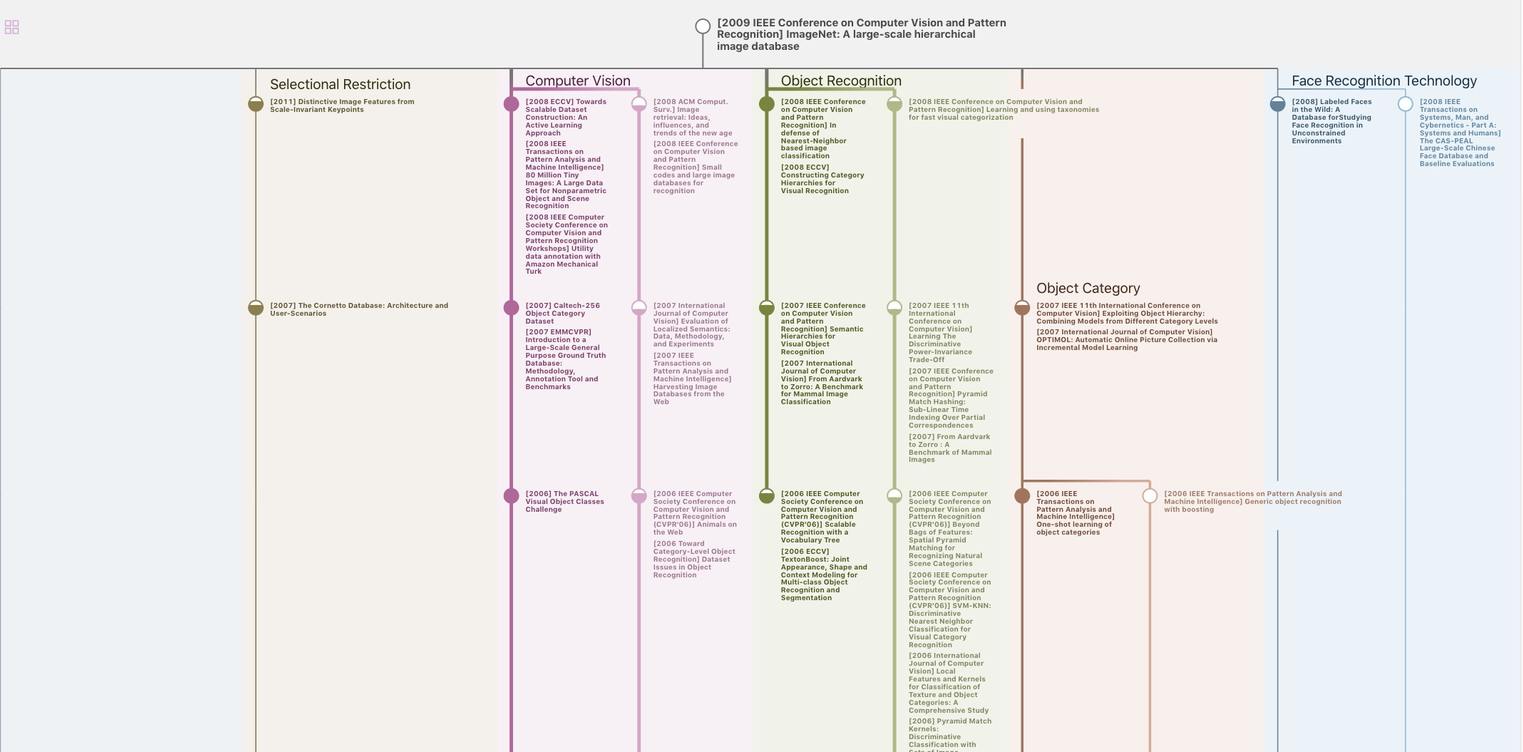
生成溯源树,研究论文发展脉络
Chat Paper
正在生成论文摘要