Classification of epileptic electroencephalogram signals using tunable-Q wavelet transform based filter-bank
Journal of Ambient Intelligence and Humanized Computing(2024)
摘要
The epilepsy is a neurological disorder and the seizure events frequently appear in epileptic patients. This disorder can be analysed through electroencephalogram (EEG) signals. In this paper, we propose a novel approach for automated identification of seizure EEG signals. The proposed method in this paper decomposes EEG signal into set of sub-band signals by applying tunable- Q wavelet transform (TQWT) based filter-bank. The sub-bands in TQWT based filter-bank have different value of quality ( Q )-factor and have nearly constant bandwidth ( BW ). The features are computed by applying cross-information potential (CIP) on N_s number of sub-band signals, for N_s values varying from two to maximum number of sub-band signals obtained from TQWT based filter-bank. The features are computed for various values of N_s and fed as input to random forest (RF) classifier. We have observed that, with the increase in the N_s , the number of computed features increases and hence the classification accuracy (ACC) depends on N_s . In this work, we have obtained ACC of 99 % in the classification of normal, seizure-free, and seizure EEG signals using our proposed method. The developed algorithm is ready to be tested using huge database and can be employed to aid the epileptologists to screen the seizure-free and seizure patients accurately.
更多查看译文
关键词
Epilepsy,Electroencephalogram signal,Tunable-Q wavelet transform,Cross-information potential,Random forest classifier,Epileptic seizure classification
AI 理解论文
溯源树
样例
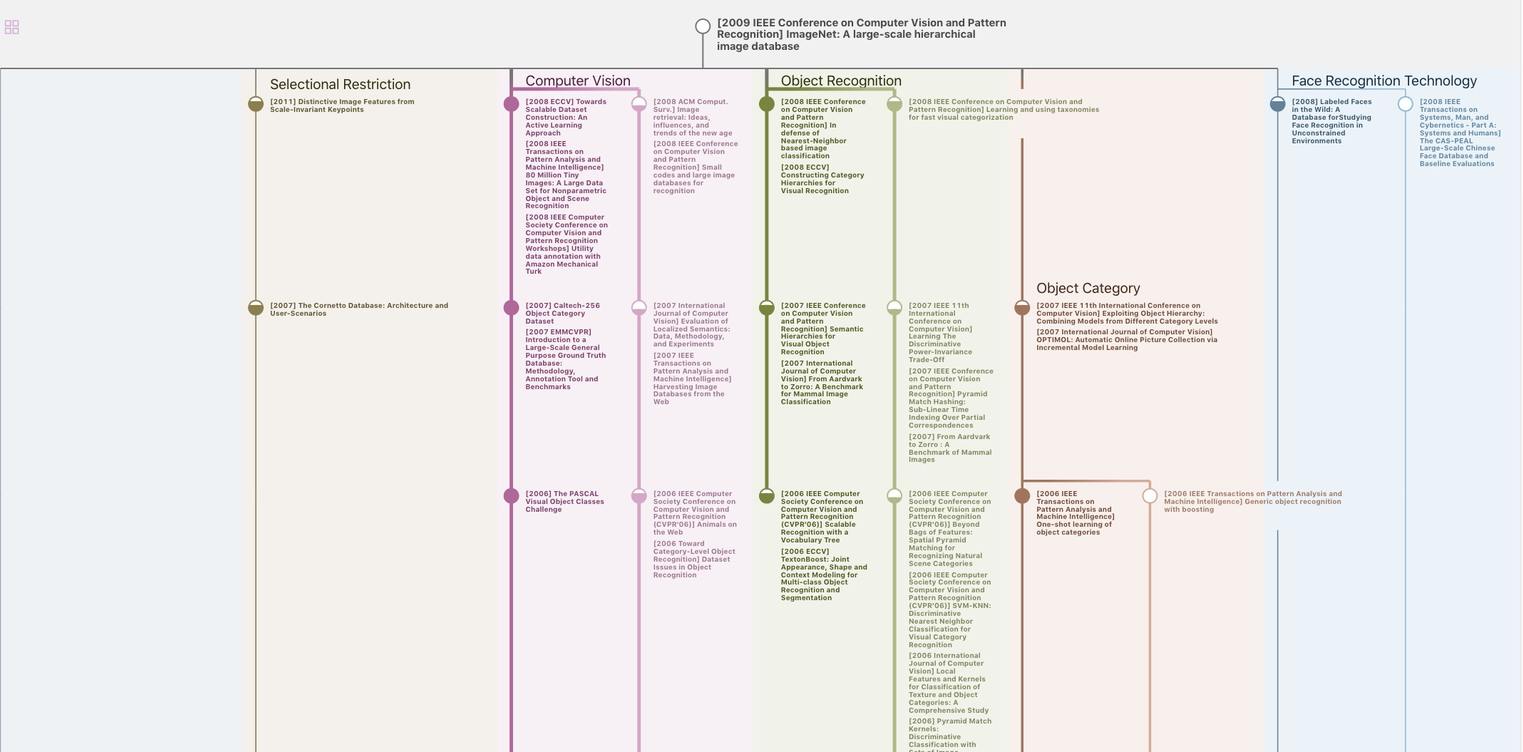
生成溯源树,研究论文发展脉络
Chat Paper
正在生成论文摘要