Load prediction in short-term implementing the multivariate quantile regression
Energy(2020)
摘要
Probability-based interim demand prediction plays and important role in managing the grid and optimizing the transmitted power through lines. Improved prediction techniques able to offer precise forecasting are supposed to be compatible with their own implementational situations in interim operation and must be highly efficient and fast. A lot of prediction techniques based on data are excessively verbose and not very suitable. The mentioned challenge emerges when the numerous demands are supposed to be forecasted at the same time, for example assessing and optimizing the energy delivery network. Here, a novel hybrid prediction framework is suggested, which improves the probability-based prediction of each load in real-time. The improvement approach uses the multi-variable quantile regression that is implemented on each prediction in real-time when a new observational data is inputted to the system. The proposed procedure is assessed using the demand data released by the Independent System Operator-new England for eight areas, which is composed of six states of the U.S. The performance of the probability-based prediction are compared to that of three other benchmarks with respect to reliability and accuracy. The suggested approach shows better accuracy compared to the highest-ranked benchmark.
更多查看译文
关键词
Hybrid prediction method,Multi-variable regression,Interim probability-based prediction,Quantile regression forest
AI 理解论文
溯源树
样例
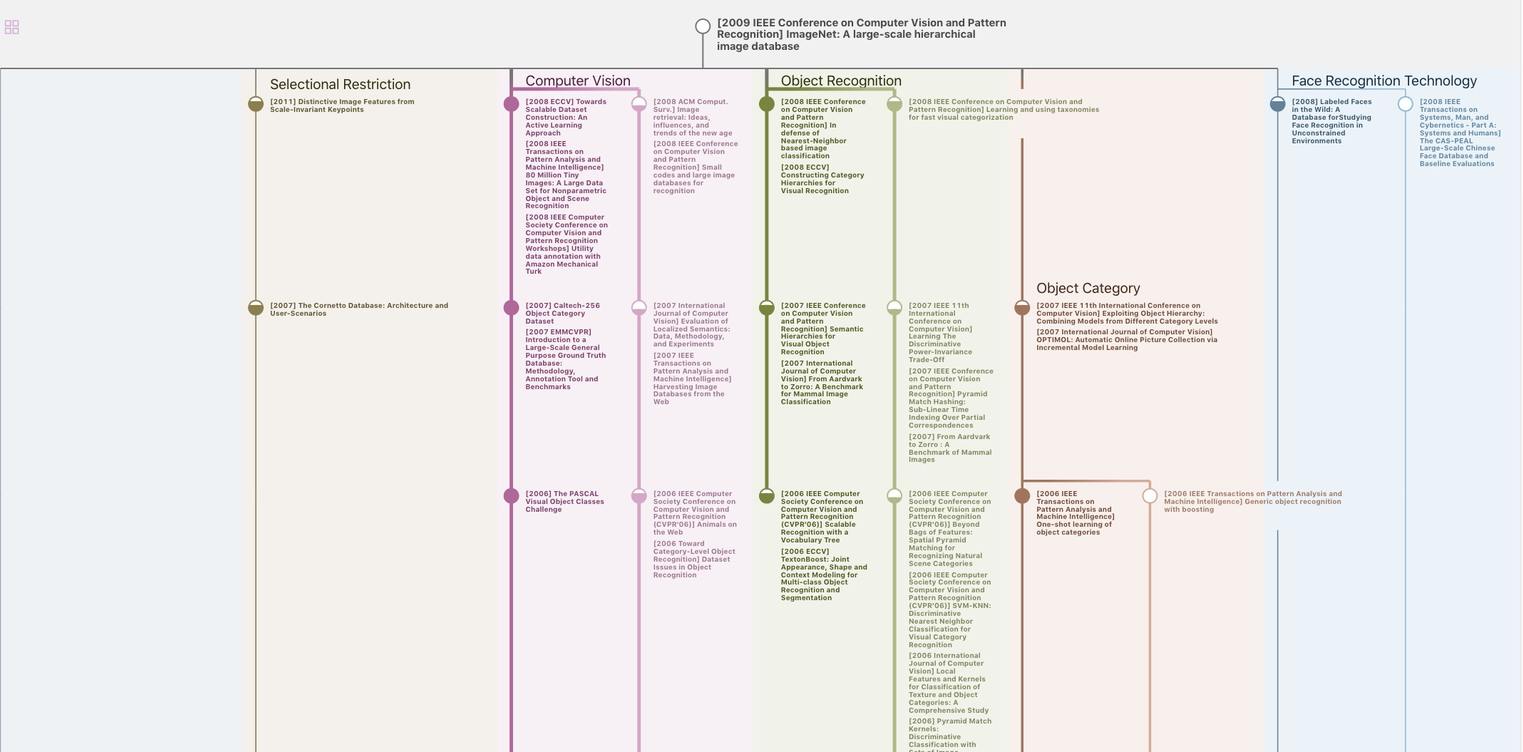
生成溯源树,研究论文发展脉络
Chat Paper
正在生成论文摘要