A comparative study of LSTM and ARIMA for energy load prediction with enhanced data preprocessing
2020 IEEE Sensors Applications Symposium (SAS)(2020)
摘要
Energy load prediction plays a central role in the decision-making process of energy production and consumption for smart homes with systems based on energy harvesting. However, forecasting energy load turned out to be a difficult problem since time series data used for the prediction involve both linear and non-linear properties. In this paper, we proposed a system which can predict a daily future energy load in a smart home based on LSTM and ARIMA models. To improve the energy load forecasting accuracy, we propose a new data preprocessing algorithm called STDAN (Same Time a Day Ago or Next) to fill the missing values. This technique is compared with well-known techniques using previous or mean values. A comparison between LSTM and ARIMA is provided for short and medium-term load forecasting. Results show that LSTM outperforms ARIMA in all cases. Finally, we also evaluated our training model based on LSTM with a new data set and the model provides an around 80% accuracy.
更多查看译文
关键词
Machine Learning,Deep Learning,Electric load,Forecasting,LSTM,ARIMA,Data Preprocessing,
AI 理解论文
溯源树
样例
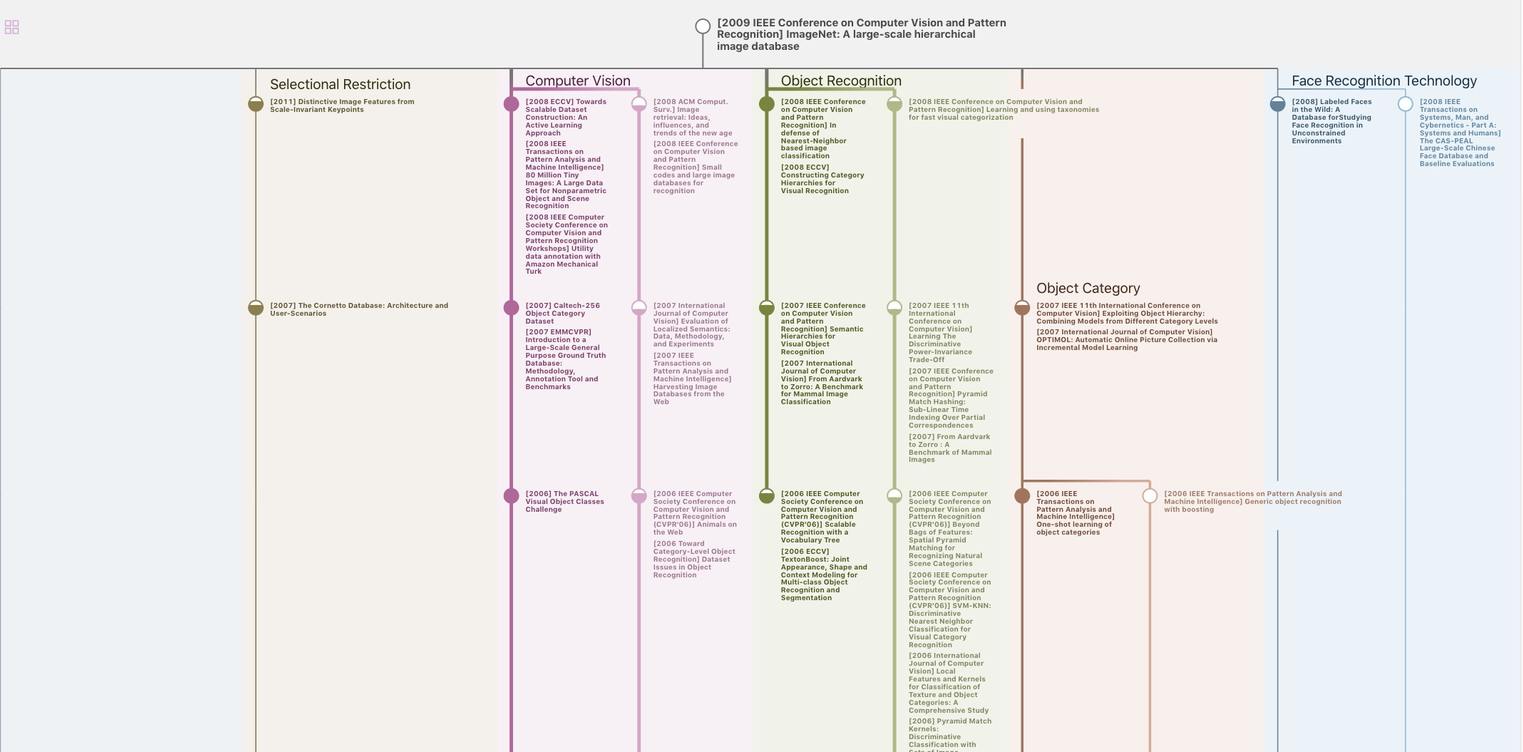
生成溯源树,研究论文发展脉络
Chat Paper
正在生成论文摘要