Cost-Sensitive Joint Feature and Dictionary Learning for Face Recognition
Neurocomputing(2020)
摘要
Dictionary learning (DL) for classification aims to learn a codebook from training samples to enhance the discriminative capability of their coding vectors. But how to determine appropriate features that can best work with the learned dictionary remains an open question. Recently, several joint feature and dictionary learning method have been proposed and achieved impressive performance. The purpose of these methods is to achieve low classification errors by implicitly assuming the costs for all misclassifications, regardless of the original labels, are the same. However, in real applications, this assumption may not hold as different kinds of mistake could produce different losses. Motivated by this concern, we propose a cost-sensitive joint feature and dictionary learning (CS-JFDL) method, in which the features are concurrently learned with the dictionaries. Our method considers the separate misclassification cost objectives during the feature and dictionary learning stages to achieve a minimum overall recognition loss. Thus, the derived feature and dictionary attain cost-sensitive constraints throughout the learning process. Extensive experimental results on both image based face recognition and image set based face recognition demonstrate that the proposed algorithm is better than many state-of-the-art methods.
更多查看译文
关键词
Face recognition,Cost-sensitive learning,Feature learning,Dictionary learning,Joint learning
AI 理解论文
溯源树
样例
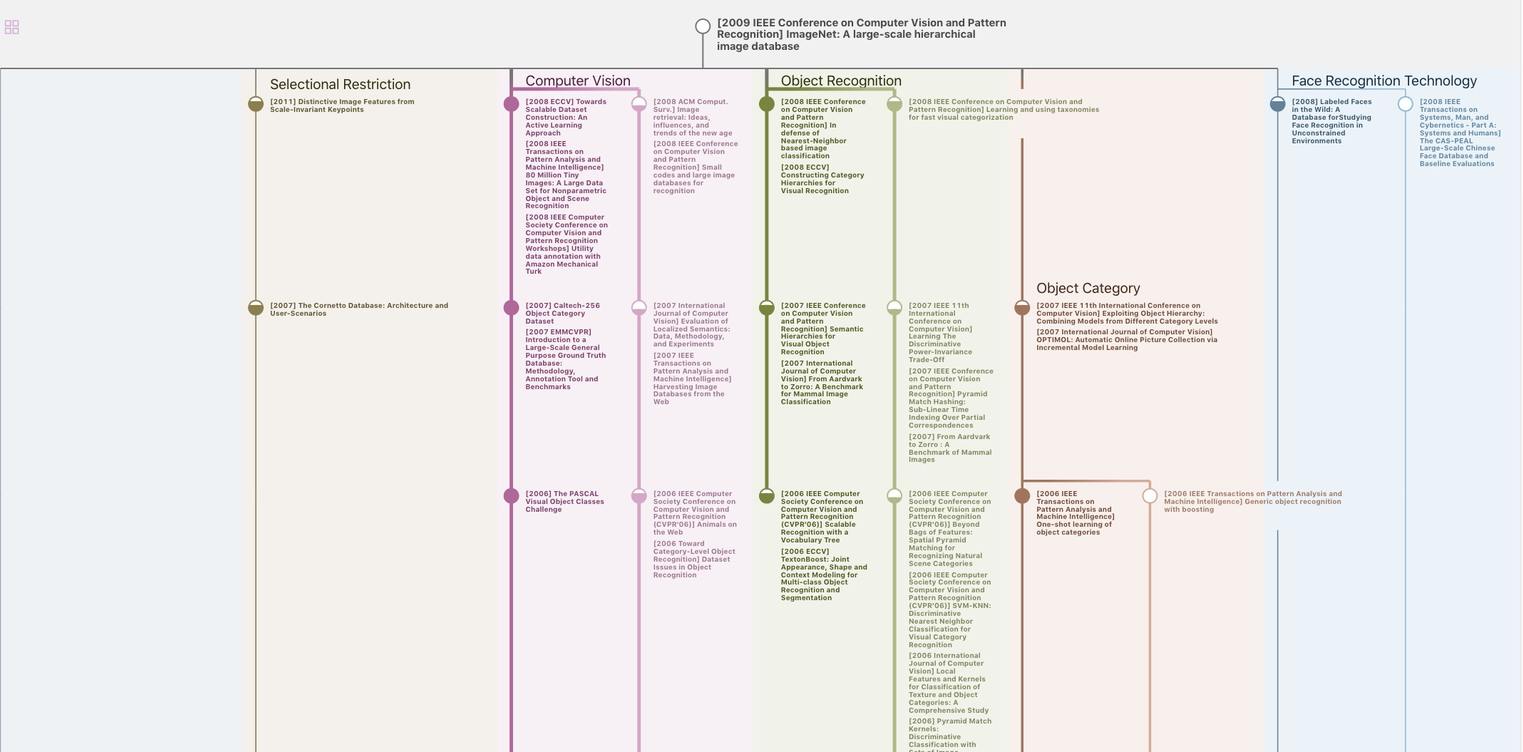
生成溯源树,研究论文发展脉络
Chat Paper
正在生成论文摘要