Multi-Label Learning via Feature and Label Space Dimension Reduction
IEEE Access(2020)
摘要
In multi-label learning, each object belongs to multiple class labels simultaneously. In the data explosion age, the size of data is often huge, i.e., large number of instances, features and class labels. The high dimension of both the feature and label spaces has posed great challenges to multi-label learning problems, e.g., high time and memory costs. In this paper, we propose a new framework for multi-label learning with a large number of class labels and features, i.e.,
M
ulti-
L
abel
L
earning via
F
eature and
L
abel
S
pace
D
imension
R
eduction, namely MLL-FLSDR. Specifically, both the feature space and label space are reduced to low dimensional spaces respectively, in which the local structure of data points is utilized to constrain the geometrical structure on both the learned low dimensional spaces and guarantee the qualities of them. Then, an effective multi-label classifier is constructed from the low dimensional feature space to the latent label space. Last, the final prediction for new test data examples can be obtained by recovering from their prediction results in the latent label space with an encoding matrix learned in the previous stage. Extensive comparison experiments with the state-of-the-art approaches manifest the effectiveness of the proposed method MLL-FLSDR.
更多查看译文
关键词
Multi-label learning,label space dimension reduction,feature space dimension reduction
AI 理解论文
溯源树
样例
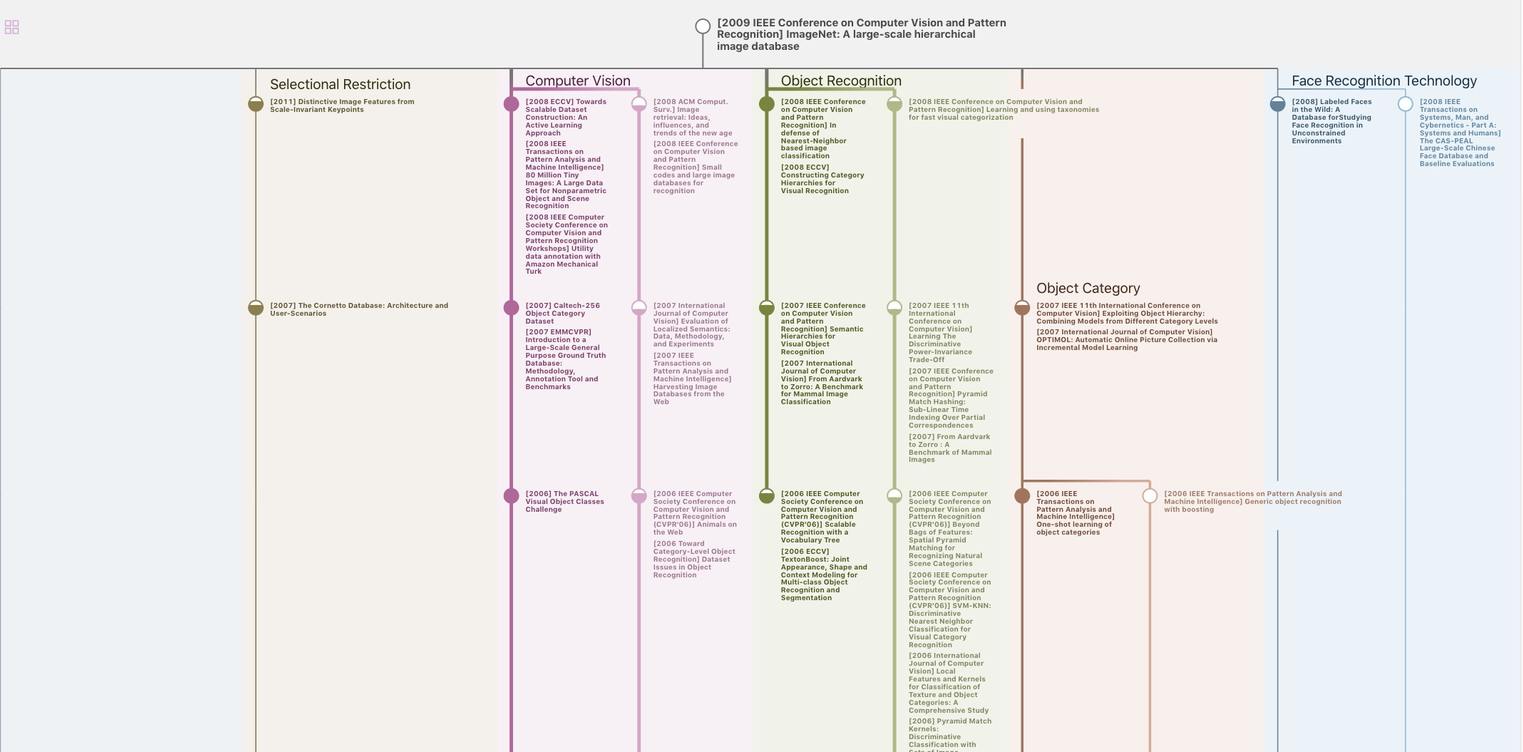
生成溯源树,研究论文发展脉络
Chat Paper
正在生成论文摘要