A global optimization strategy based on the Kriging surrogate model and parallel computing
Structural and Multidisciplinary Optimization(2020)
摘要
The computational efficiency and accuracy of the global solution are the main performance indicators of an optimization algorithm to solve the structural and multidisciplinary optimization problems. The Kriging-based optimization algorithm can satisfy certain engineering requirements by applying the single-point sequence sampling method. However, this conventional algorithm does not efficiently apply the parallel performance of high-performance multi-core computers. This study aims to propose a global optimization strategy based on the Kriging surrogate model and parallel computing depending on the multi-peak characteristics of the expect improvement (EI) function. The proposed method searches out the locations of multiple peaks of the EI function by introducing a so-called P -EI function; it then simultaneously includes multiple sampling points located nearby these peaks. Furthermore, an efficient design domain reduction technique is applied to improve the accuracy of the global solution. When compared with the traditional Kriging-based methods, the proposed method can effectively obtain multiple peaks of the EI function without solving complex expressions of the high-dimensional EI function exhibiting a joint probability density distribution. The parallel computation ability, global performance, and solution accuracy of our method are validated via typical test functions and structural optimization problems.
更多查看译文
关键词
Global optimization,Parallel computing,Multi-point sampling,Surrogate model
AI 理解论文
溯源树
样例
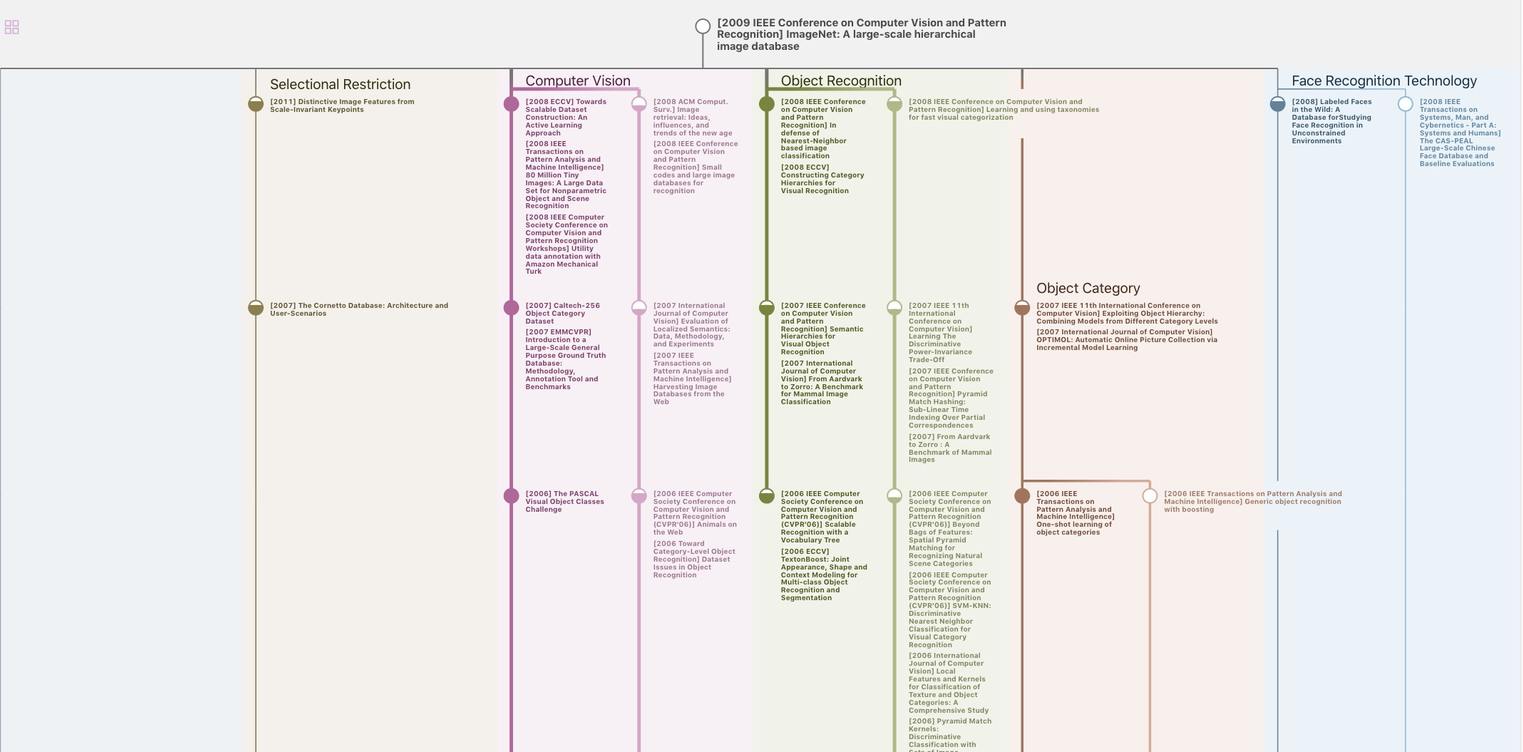
生成溯源树,研究论文发展脉络
Chat Paper
正在生成论文摘要