Rolling Bearing Fault Diagnosis Based on Wavelet Packet Transform and Convolutional Neural Network
APPLIED SCIENCES-BASEL(2020)
摘要
Timely sensing the abnormal condition of the bearings plays a crucial role in ensuring the normal and safe operation of the rotating machine. Most traditional bearing fault diagnosis methods are developed from machine learning, which might rely on the manual design features and prior knowledge of the faults. In this paper, based on the advantages of CNN model, a two-step fault diagnosis method developed from wavelet packet transform (WPT) and convolutional neural network (CNN) is proposed for fault diagnosis of bearings without any manual work. In the first step, the WPT is designed to obtain the wavelet packet coefficients from raw signals, which then are converted into the gray scale images by a designed data-to-image conversion method. In the second step, a CNN model is built to automatically extract the representative features from gray images and implement the fault classification. The performance of the proposed method is evaluated by a real rolling-bearing dataset. From the experimental study, it can be seen the proposed method presents a more superior fault diagnosis capability than other machine-learning-based methods.
更多查看译文
关键词
fault diagnosis,convolution neural networks,wavelet packet transform,data-to-image conversion,bearing
AI 理解论文
溯源树
样例
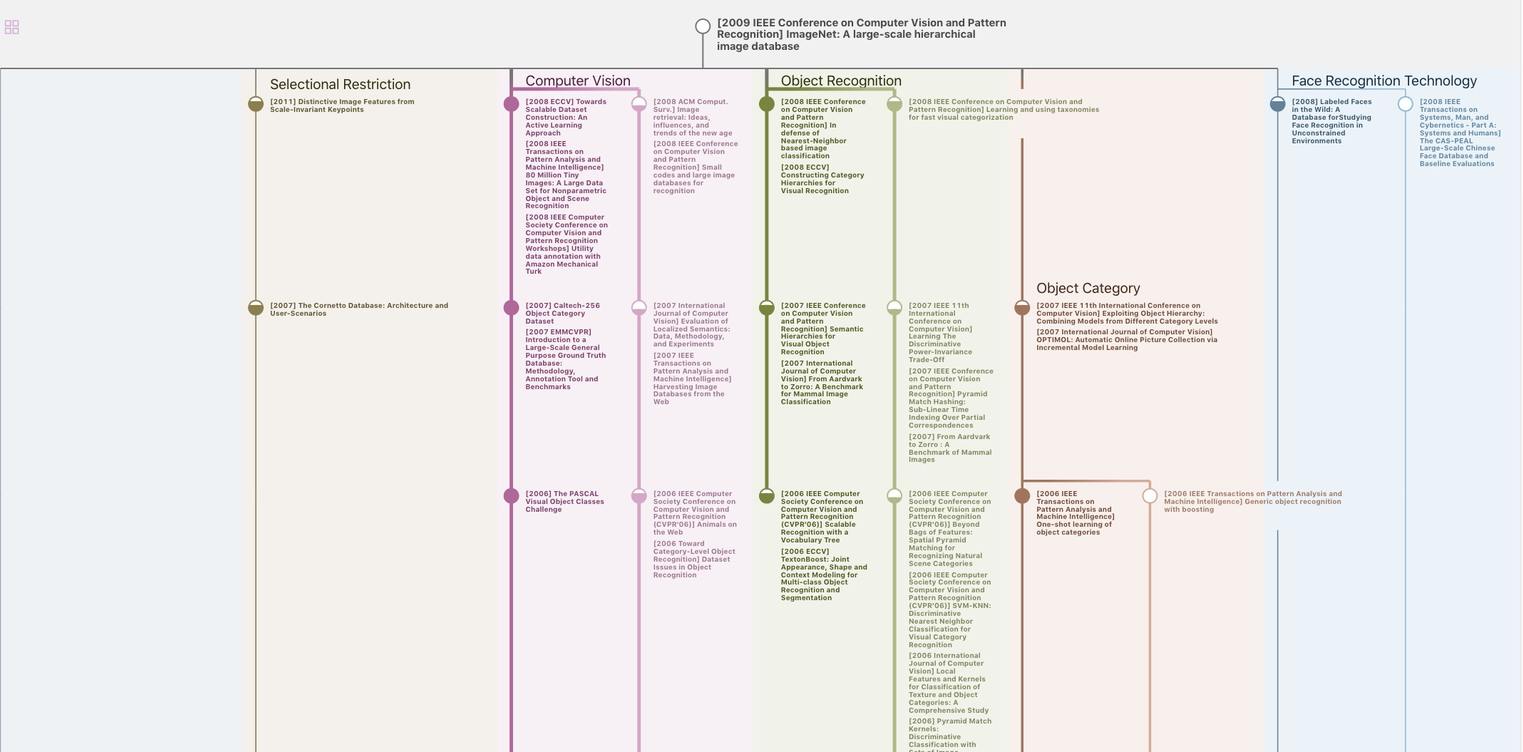
生成溯源树,研究论文发展脉络
Chat Paper
正在生成论文摘要