A Probabilistic Model-based Online Learning Optimal Control Algorithm for Soft Pneumatic Actuators
international conference on robotics and automation(2020)
摘要
Soft robots are increasingly being employed in different fields and various designs are created to satisfy relevant requirements. The wide ranges of design bring challenges to soft robotic control in that a unified control framework is difficult to derive. Traditional model-driven approaches for soft robots are usually design-specific which highly depend on specific design structures. Our approach to such challenges involves a probabilistic model that learns a mapping from the soft actuator states and controls to the next states. Then an optimal control policy is derived by minimizing a cost function based on the probabilistic model. We demonstrate the efficiency of our approach through simulations with parameter analysis and real-robot experiments involving three different designs of soft pneumatic actuators. Comparisons with previous model-based controllers are also provided to show advantages of the proposed method. Overall, this work provides a promising design-independent control approach for the soft robotics community.
更多查看译文
关键词
Modeling, control and learning for soft robots, model learning for control, optimization and optimal control
AI 理解论文
溯源树
样例
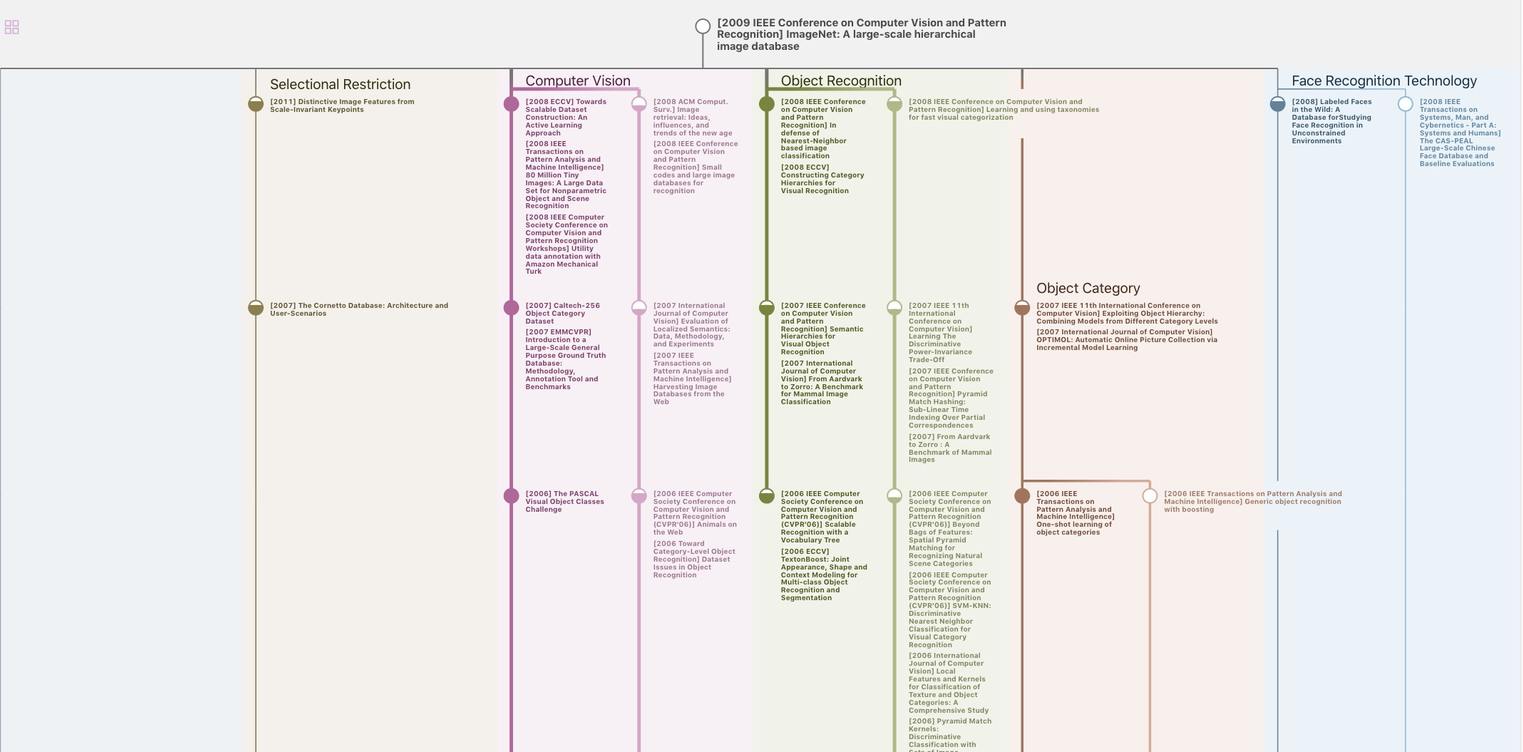
生成溯源树,研究论文发展脉络
Chat Paper
正在生成论文摘要