Image Denoising Using Eigenvectors of Graph Laplacian with Low-Rank Approximation
2019 International Conference on Artificial Intelligence and Advanced Manufacturing (AIAM)(2019)
摘要
Recently, a method based on eigenvectors of graph Laplacian (EGL) is proposed for image denoising. However, the EGL employs a complex measure based on the Euclidean distance to describe the similarity between patch pair, which has limitation to some degree in high-level noise. In this paper, we proposed an image denoising algorithm using eigenvectors of graph Laplacian with distance estimation via low-rank approximation (LR-EGL) to make up for this defect. In detail, with probability distribution of distance as prior knowledge, low-rank approximation is employed to estimate the distance between similar patches in underlying clean image. Then, the estimated distance is fused into EGL algorithm to construct graph Laplacian matrix and generate reliable eigenvectors to denoising. Experiments demonstrate that our algorithm outperforms EGL and is comparable with well-developed methods, especially with high-level noise.
更多查看译文
关键词
image denoising, distance estimation, low-rank approximation, graph Laplacian
AI 理解论文
溯源树
样例
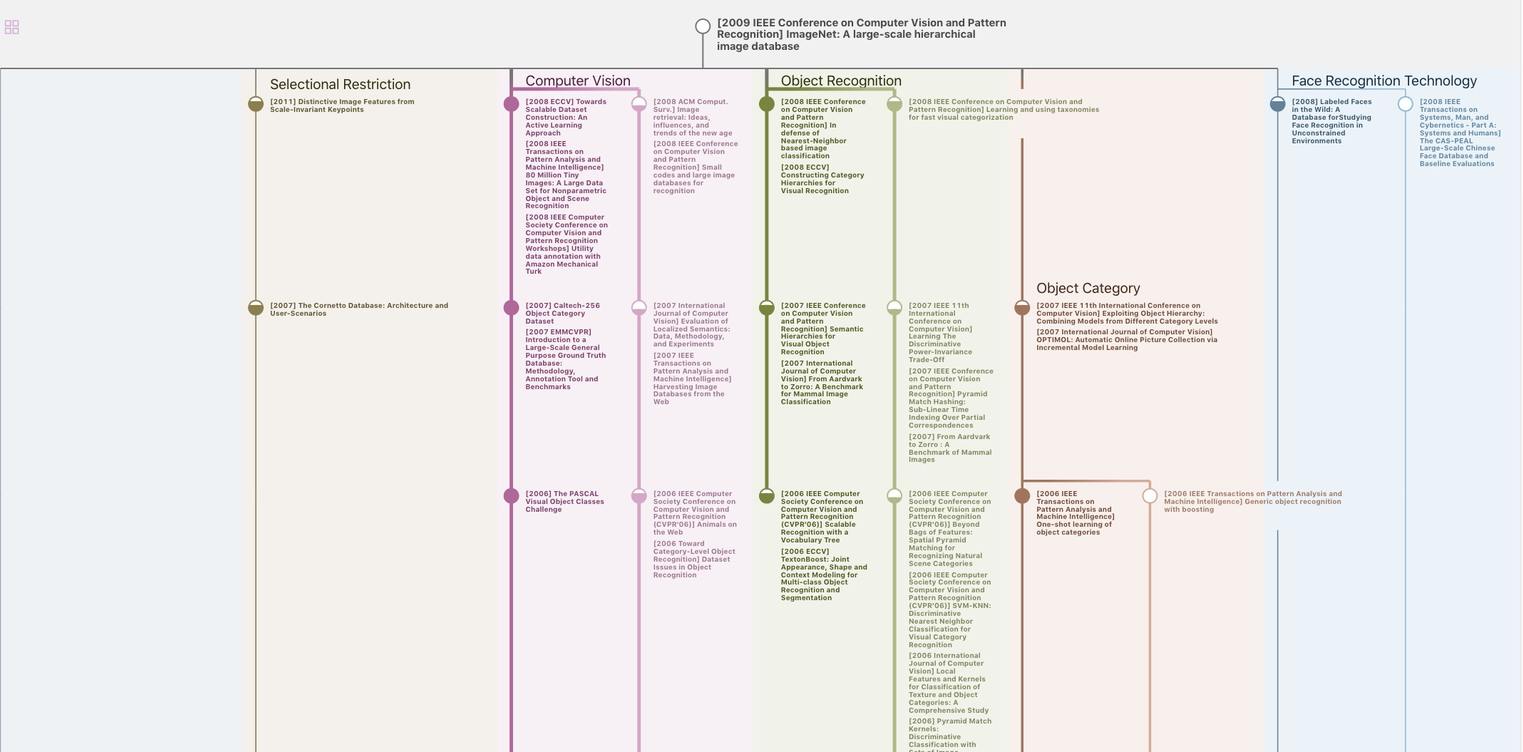
生成溯源树,研究论文发展脉络
Chat Paper
正在生成论文摘要