Merging Similar Neurons for Deep Networks Compression
Cognitive Computation(2020)
摘要
Deep neural networks have achieved outstanding progress in many fields, such as computer vision, speech recognition and natural language processing. However, large deep neural networks often need huge storage space and long training time, making them difficult to apply to resource restricted devices. In this paper, we propose a method for compressing the structure of deep neural networks. Specifically, we apply clustering analysis to find similar neurons in each layer of the original network, and merge them and the corresponding connections. After the compression of the network, the number of parameters in the deep neural network is significantly reduced, and the required storage space and computational time is greatly reduced as well. We test our method on deep belief network (DBN) and two convolutional neural networks. The experimental results demonstrate that our proposed method can greatly reduce the number of parameters of the deep networks, while keeping their classification accuracy. Especially, on the CIFAR-10 dataset, we have compressed VGGNet with compression ratio 92.96%, and the final model after fine-tuning obtains even higher accuracy than the original model.
更多查看译文
关键词
Machine learning, Deep neural networks, Structure compression, Neurons, Clustering
AI 理解论文
溯源树
样例
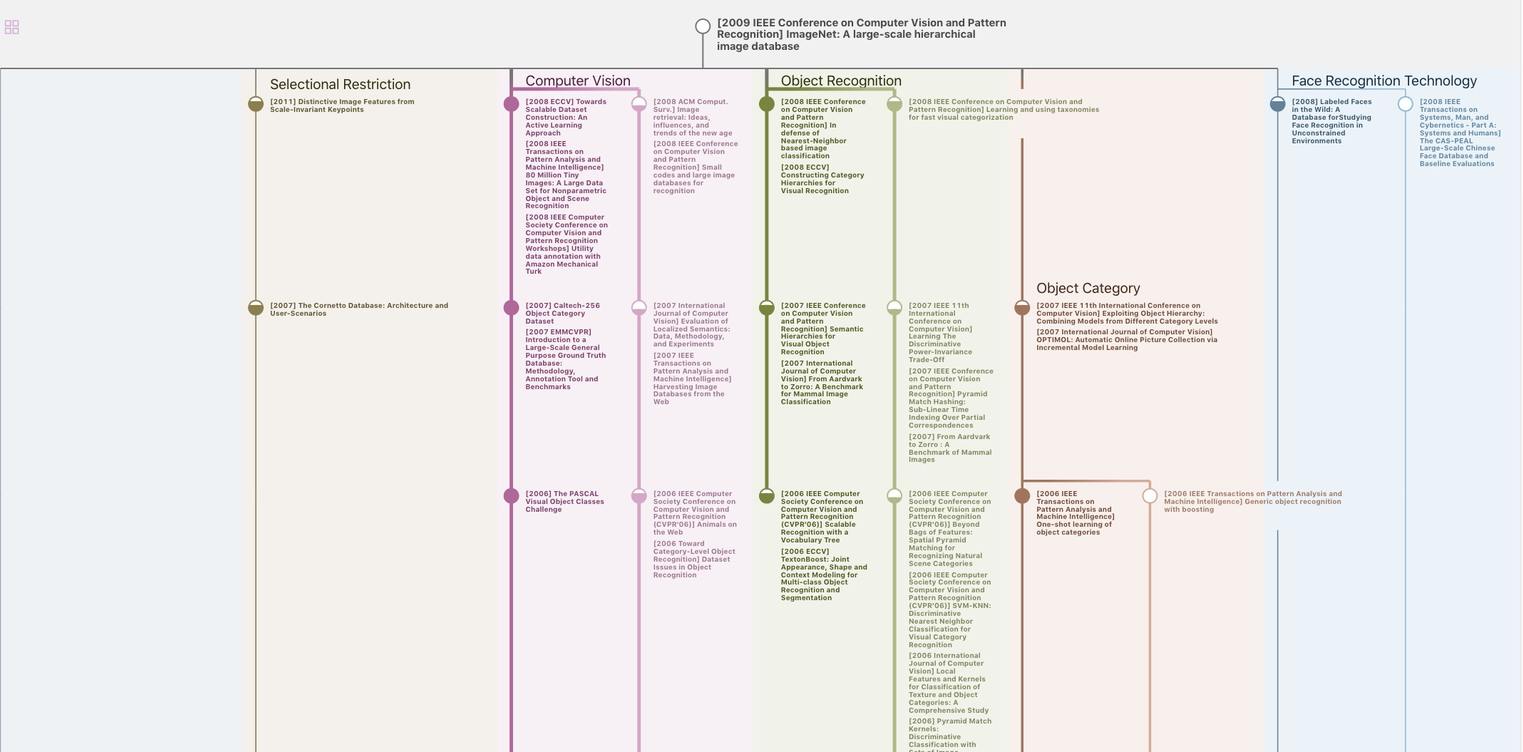
生成溯源树,研究论文发展脉络
Chat Paper
正在生成论文摘要