Fast discrete factorization machine for personalized item recommendation
Knowledge-Based Systems(2020)
摘要
Personalized item recommendation has become an essential target of Web applications, but it suffers from the efficiency problem due to a large volume of data. In particular, feature-based factorization machine models are generally limited by the vast number of feature dimensions, leading to catastrophic computation time. In this paper, we propose a Fast Discrete Factorization Machine (FDFM) method to resolve these issues by applying the hash coding technologies to factorization machine models. Specifically, it discretizes the real-valued feature vectors in the parameter model during the process of learning personalized item rankings, whereby the overall computational time can be greatly reduced. Besides, we propose convergence update rules to optimize the quantization loss of the binarization problem, which can be used in personalized ranking scenarios efficiently. Based on the evaluation in two real-world datasets, our proposed approach consistently shows better performance than other baselines, especially when using shorter binary codes.
更多查看译文
关键词
Discretization,Factorization machine,Item recommendation
AI 理解论文
溯源树
样例
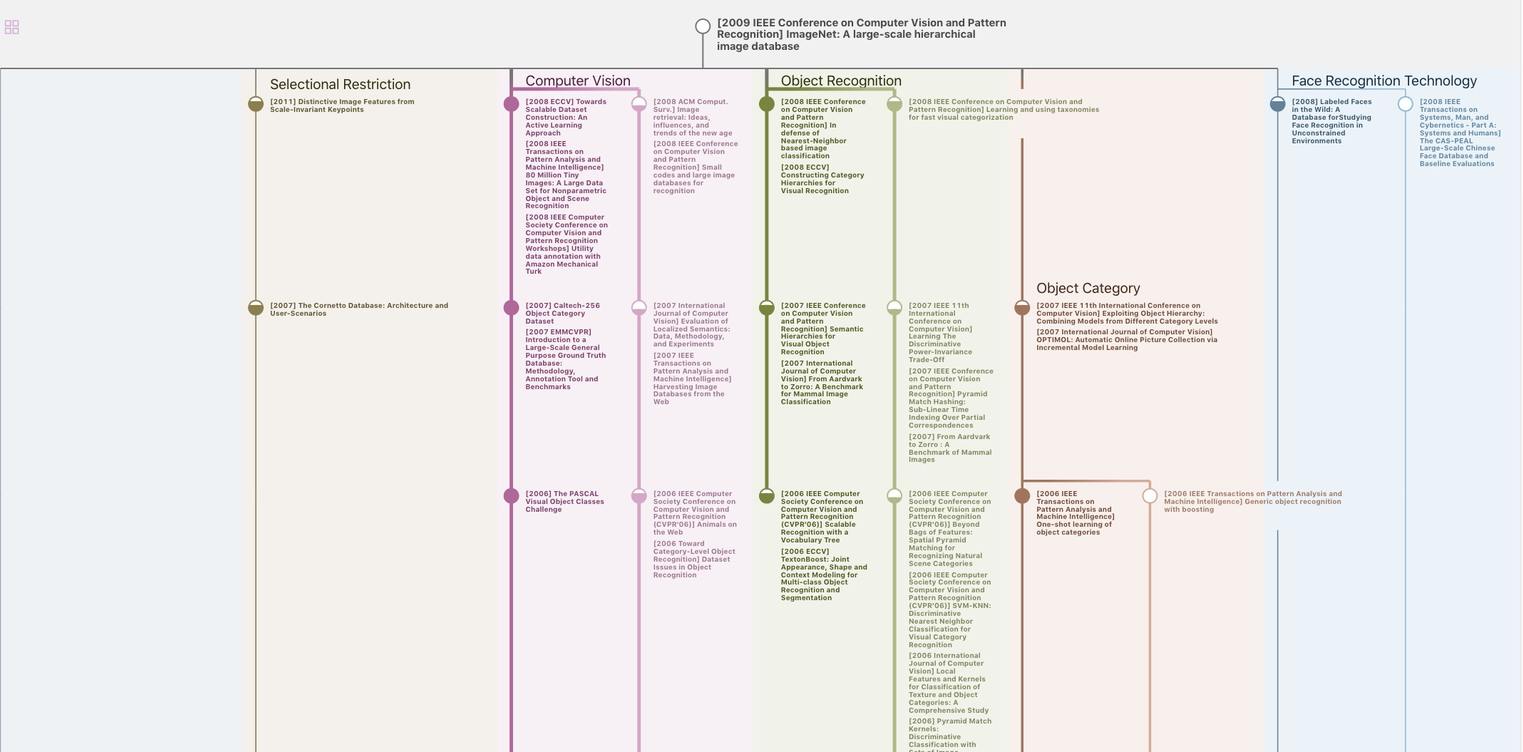
生成溯源树,研究论文发展脉络
Chat Paper
正在生成论文摘要