Parity-based robust data-driven fault detection for nonlinear systems using just-in-time learning approach:
TRANSACTIONS OF THE INSTITUTE OF MEASUREMENT AND CONTROL(2020)
摘要
This paper discusses a framework for fault detection in nonlinear processes. A novel subspace aided parity-based data-driven technique is proposed using the so-called just-in-time learning (JITL) approach. The idea of the proposed technique is to choose a set of the most similar data samples from the database for each test data sample using the JITL approach to address the nonlinearity problem. The parity vector is constructed for each test sample using the selected data samples to generate the residual signal. The salient features of the proposed technique include easy and simple implementation together with effectiveness in fault detection in the presence of disturbances and measurement noise. The fault detection scheme is so designed that it exhibits robustness against sensor noise and disturbances and sensitivity to faults. A case study for the fault detection of a nuclear research reactor (NRR) is presented to demonstrate the efficacy of the technique. The NRR is a highly nonlinear and complex process. Two faults of NRR, namely external reactivity insertion and control rod withdrawal, are successfully detected using the proposed approach.
更多查看译文
关键词
Fault detection,nonlinear system,industrial process,data-driven technique,just-in-time learning
AI 理解论文
溯源树
样例
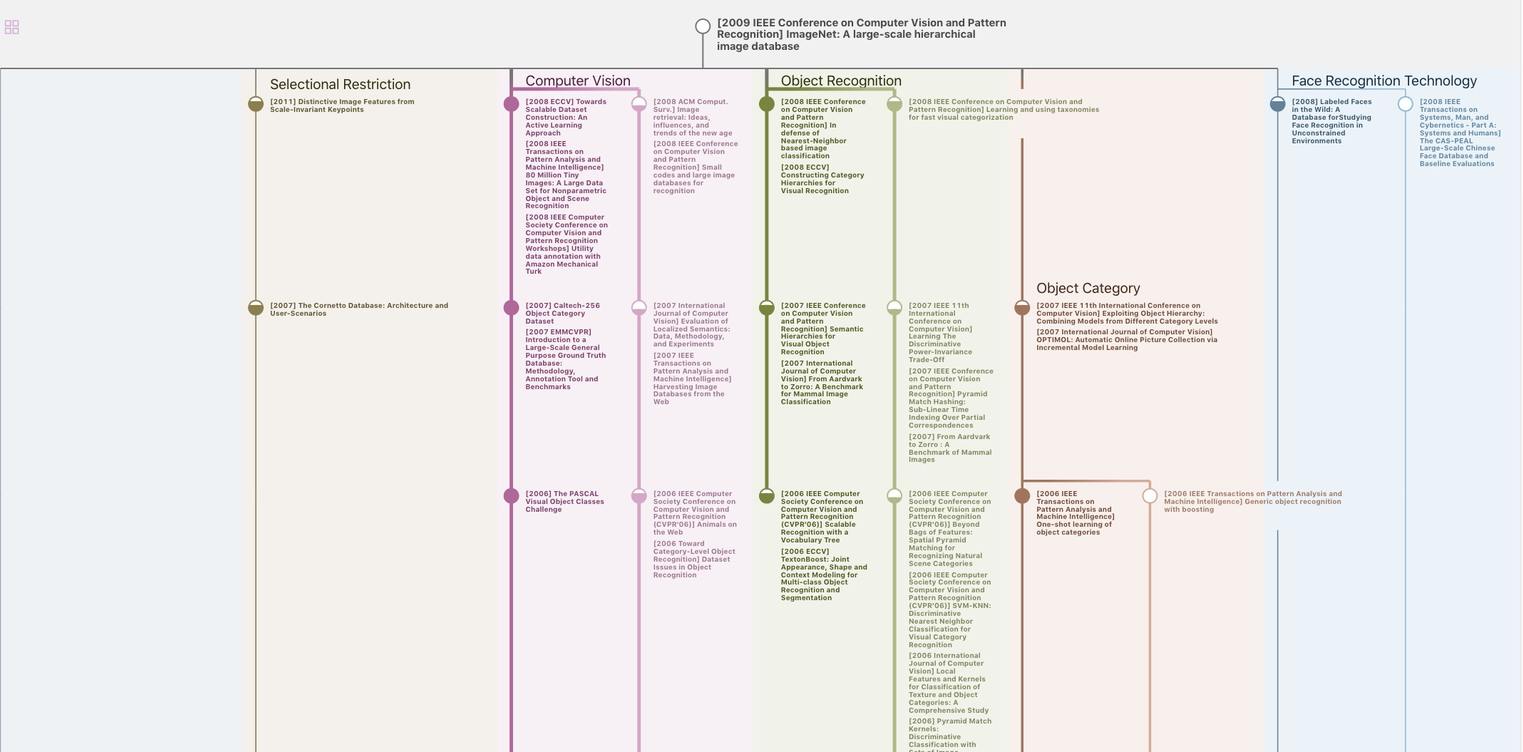
生成溯源树,研究论文发展脉络
Chat Paper
正在生成论文摘要