Atrial Fibrillation Detection Using MEMS Accelerometer Based Bedsensor
2019 Computing in Cardiology (CinC)(2019)
摘要
Atrial fibrillation (AFib) is the most common cardiac arrhythmia, affecting eventually up to a quarter of the population. The purpose of this small scale clinical study was to validate the usability of MEMS accelerometer based bedsensor for detection of AFib. A Murata accelerometer based ballistocardiogram bedsensor was attached under the hospital bed magnetically and measurement data was recorded from 20 AFib patients and 15 healthy volunteers, mainly females. The recording time was up 30 minutes. The sensor built-in algorithms automatically extracted features such as heart rate (HR), heart rate variability (HRV), relative stroke volume (SVOL), signal strength (SS) and whether the patient is in bed or not. We calculated median values for each feature HR, HRV, SVOL and SS, and investigated whether it is possible to separate AFib from healthy with these features or their combinations. Areas under the curve (AUC) were 0.98 for full length signals and 0.85 for 3 min signal segments using random forest (RF) classifier corresponding to sensitivity and specificity of 100% and 93.3% for full length signals and 90% and 80% for 3 min signals. We conclude, that based on our pilot results, the Murata bedsensor is able to detect AFib, and seems to be a promising technology for long-term monitoring of AFib at home settings as it requires only one-time installation and operational time can be up to years and even tens of years.
更多查看译文
关键词
random forest classifier,relative stroke volume,heart rate variability,automatically extracted features,signal segments,signal strength,HRV,recording time,healthy volunteers,AFib patients,hospital bed,Murata accelerometer based ballistocardiogram bedsensor,scale clinical study,cardiac arrhythmia,MEMS accelerometer based bedsensor,atrial fibrillation detection,time 3.0 min
AI 理解论文
溯源树
样例
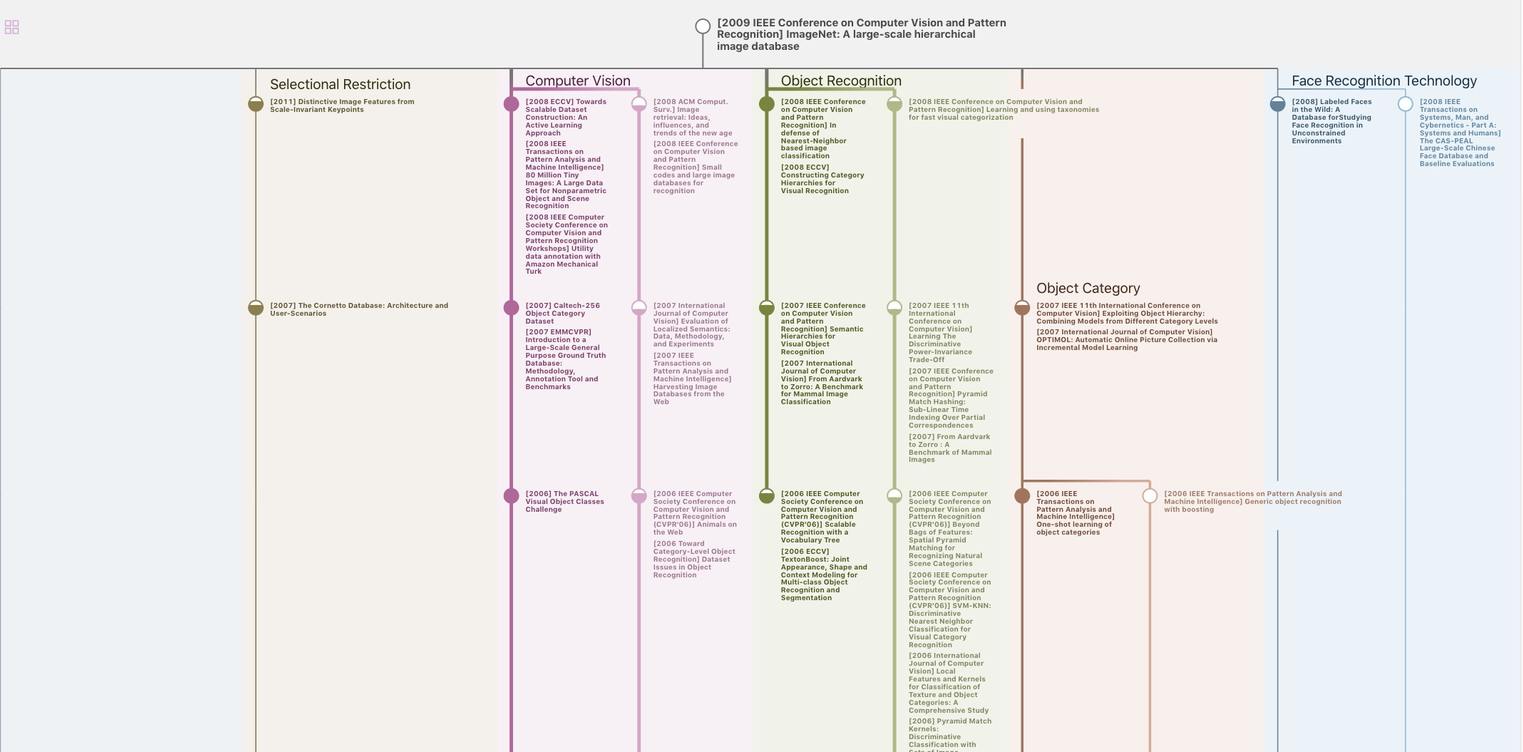
生成溯源树,研究论文发展脉络
Chat Paper
正在生成论文摘要