Hyperspectral anomaly detection by local joint subspace process and support vector machine
INTERNATIONAL JOURNAL OF REMOTE SENSING(2020)
摘要
Hyperspectral anomaly detection is a challenging task due to the inaccurate evaluation of background statistics and the contamination of anomaly pixels. In this paper, we propose an effective hyperspectral anomaly detection algorithm based on local joint subspace process and support vector machine (SVM). The method mainly consists of three steps. At first, in the local joint subspace process, we combine Mahalanobis distance detection and spectral angular distance detection of subspace projection by local sliding change dual windows. With this local joint subspace process, the spatial-spectral information is jointly utilized and the local subspace score is also obtained. Then, to further improve the detection probability, we propose the support vector score by classifying the dimensionally reduced hyperspectral image data through a trained SVM model. Finally, we obtain the final detection result from the linear combination of above scores. Compared with alternative methods, the proposed method has shown a superior performance on both synthetic and real-world datasets.
更多查看译文
关键词
Support Vector Machines,Hyperspectral Imaging,Hyperspectral,Spectral Unmixing,Change Detection
AI 理解论文
溯源树
样例
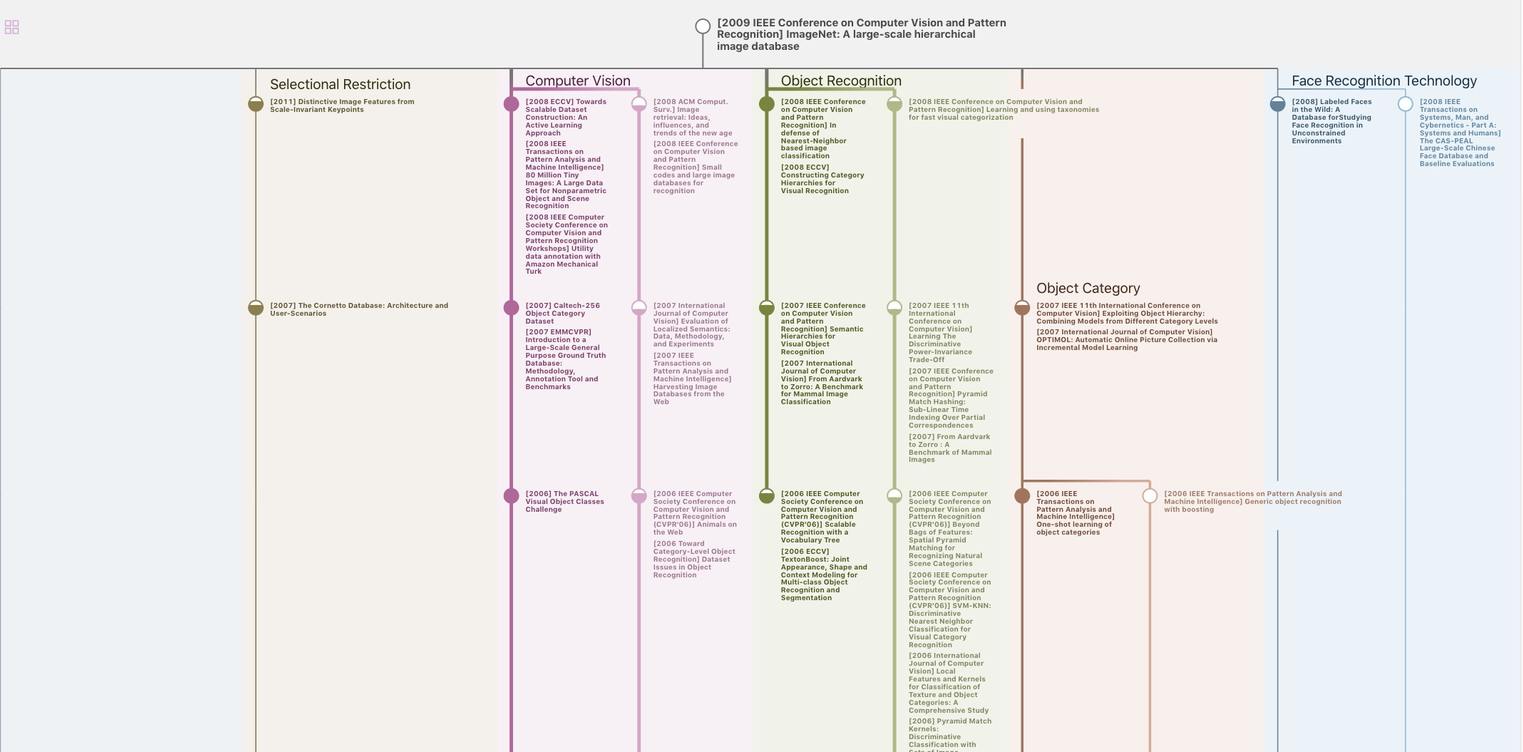
生成溯源树,研究论文发展脉络
Chat Paper
正在生成论文摘要