A Multi-Task Imputation and Classification Neural Architecture for Early Prediction of Sepsis from Multivariate Clinical Time Series
CinC(2019)
摘要
Early prediction of sepsis onset can notify clinicians to provide timely interventions to patients to improve their clinical outcomes. The key question motivating this work is: given a retrospective patient cohort consisting of multivariate clinical time series (e.g., vital signs and lab measurement) and patients' demographics, how to build a model to predict the onset of sepsis six hours earlier? To tackle this challenge, we first used a recurrent imputation for time series (RITS) approach to impute missing values in multivariate clinical time series. Second, we applied temporal convolutional networks (TCN) to the RITS-imputed data. Compared to other sequence prediction models, TCN can effectively control the size of sequence history. Third, when defining the loss function, we assigned custom time- dependent weights to different types of errors. We achieved 9th place (team name = prna, utility score = 0.328) at the 2019 PhysioNet Computing in Cardiology Challenge, which evaluated our proposed model on a real-world sepsis patient cohort. At a follow-up ‘hackathon’ event, held by the challenge organizers, an improved version of our algorithm achieved 2nd place (utility score = 0.342).
更多查看译文
关键词
classification neural architecture,multivariate clinical time series,clinical outcomes,retrospective patient cohort,RITS-imputed data,sequence prediction models,multitask imputation,early prediction,recurrent imputation for time series approach,temporal convolutional networks,TCN,real-world sepsis patient cohort
AI 理解论文
溯源树
样例
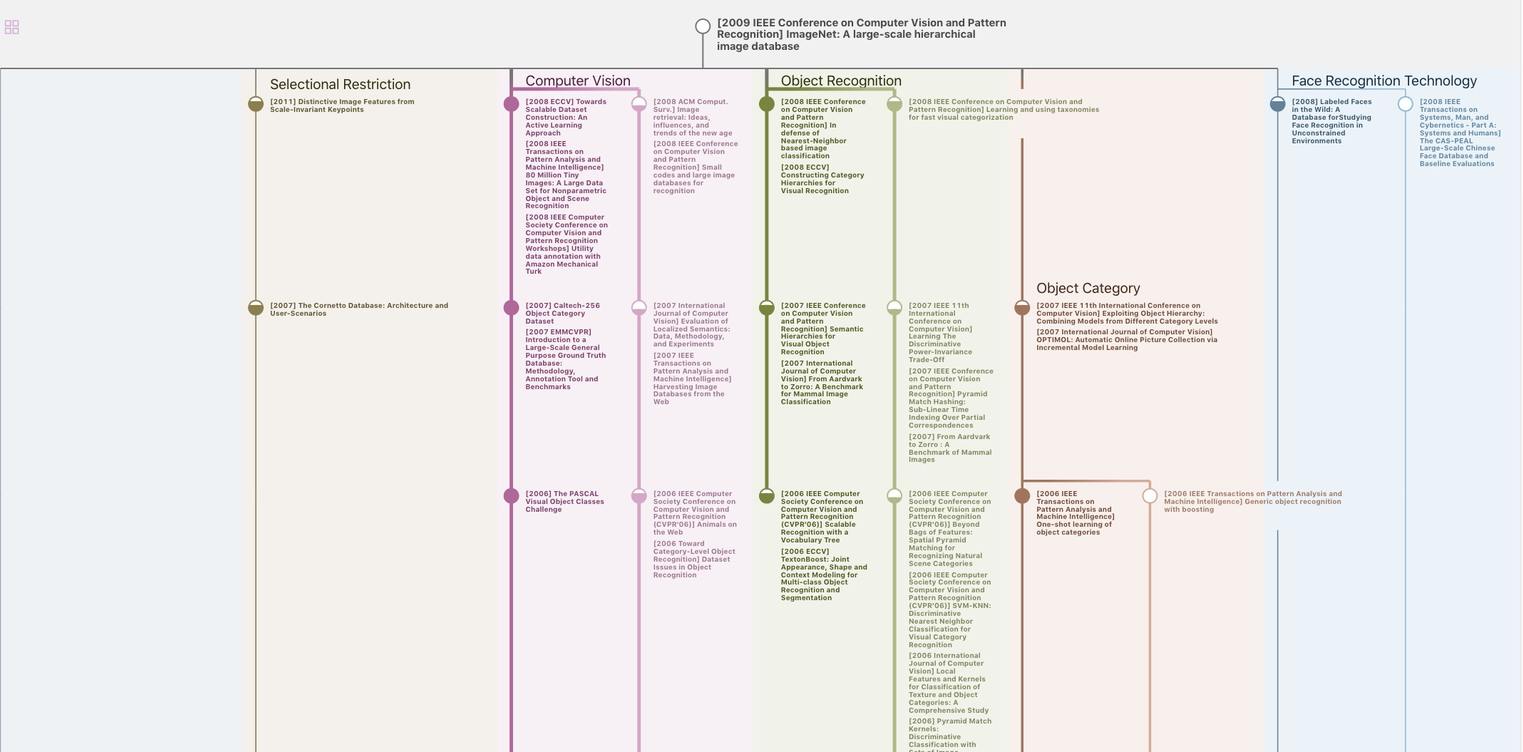
生成溯源树,研究论文发展脉络
Chat Paper
正在生成论文摘要