Models as Approximations I: Consequences Illustrated with Linear Regression
STATISTICAL SCIENCE(2019)
摘要
In the early 1980s, Halbert White inaugurated a "model-robust" form of statistical inference based on the "sandwich estimator" of standard error. This estimator is known to be "heteroskedasticity-consistent," but it is less well known to be "nonlinearity-consistent" as well. Nonlinearity, however, raises fundamental issues because in its presence regressors are not ancillary, hence cannot be treated as fixed. The consequences are deep: (1) population slopes need to be reinterpreted as statistical functionals obtained from OLS fits to largely arbitrary joint x-y distributions; (2) the meaning of slope parameters needs to be rethought; (3) the regressor distribution affects the slope parameters; (4) randomness of the regressors becomes a source of sampling variability in slope estimates of order 1/v N; (5) inference needs to be based on model-robust standard errors, including sandwich estimators or the x-y bootstrap. In theory, model-robust and model-trusting standard errors can deviate by arbitrary magnitudes either way. In practice, significant deviations between them can be detected with a diagnostic test.
更多查看译文
关键词
Ancillarity of regressors,misspecification,econometrics,sandwich estimator,bootstrap
AI 理解论文
溯源树
样例
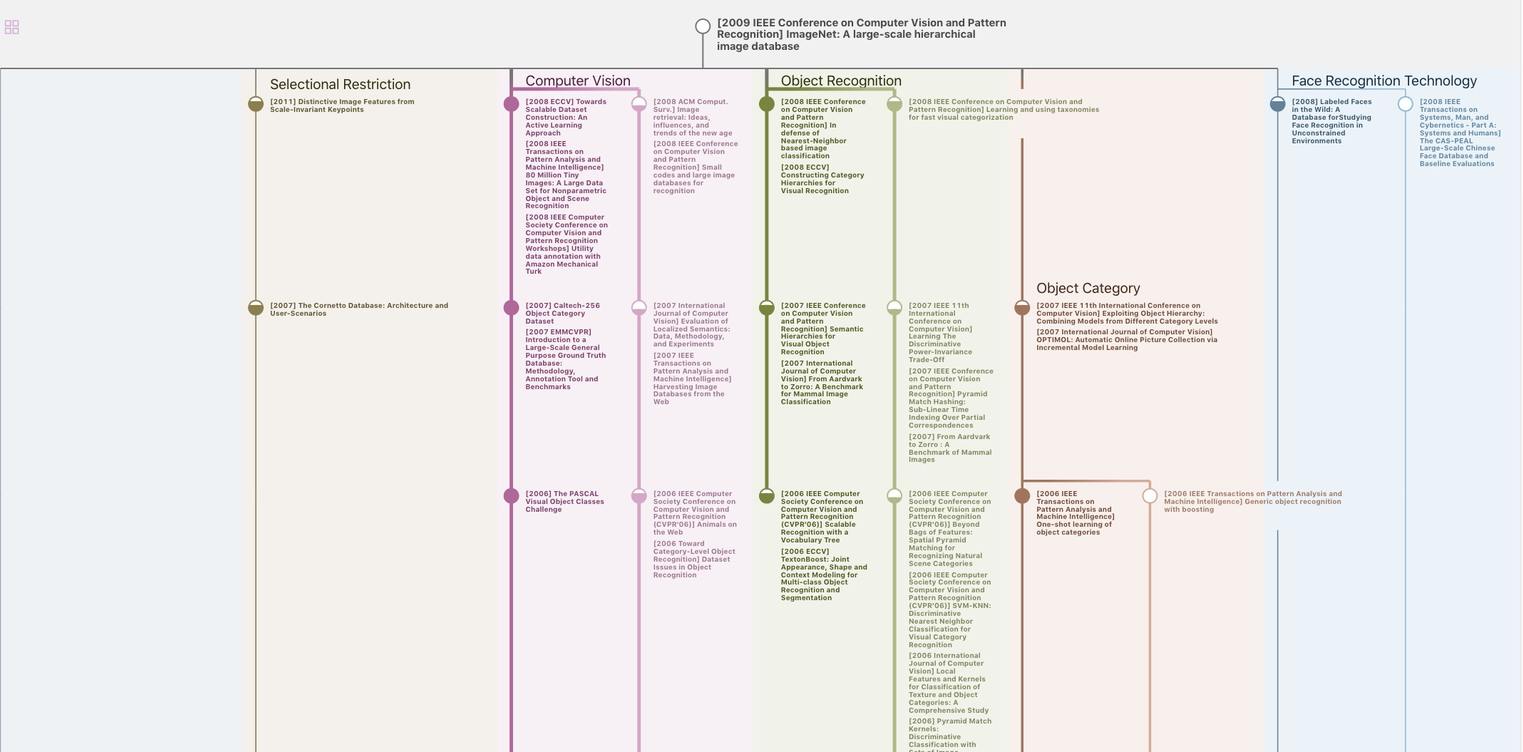
生成溯源树,研究论文发展脉络
Chat Paper
正在生成论文摘要