Q-Learning-Based Fuzzy Logic for Multi-objective Routing Algorithm in Flying Ad Hoc Networks
Wireless Personal Communications(2020)
摘要
Flying ad hoc networks (FANETs) that consist of multiple unmanned aerial vehicles (UAVs) have developed owing to the rapid technological evolution of electronics, sensors, and communication technologies. In this paper, we propose a multi-objective routing algorithm for FANETs. In addition to the basic transmission performance in the construction of the routing path, the network impact according to the mobility of the UAV nodes and the energy state of each node should be considered because of the characteristics of the FANET, and the overall efficiency and safety of the network should be satisfied. We therefore propose the use of Q-learning-based fuzzy logic for the FANET routing protocol. The proposed algorithm facilitates the selection of the routing paths to be processed in terms of link and overall path performances. The optimal routing path to the destination is determined by each UAV using a fuzzy system with link- and path-level parameters. The link-level parameters include the transmission rate, energy state, and flight status between neighbor UAVs, while the path-level parameters include the hop count and successful packet delivery time. The path-level parameters are dynamically updated by the reinforcement learning method. In the simulation results, we compared the proposal with the conventional fuzzy logic and Q-value-based ad hoc on-demand distance vector. The results show that the proposed method can maintain low hop count and energy consumption and prolong the network lifetime.
更多查看译文
关键词
Routing algorithm,Flying ad hoc networks,Multi-objective,Fuzzy logic,Q-learning
AI 理解论文
溯源树
样例
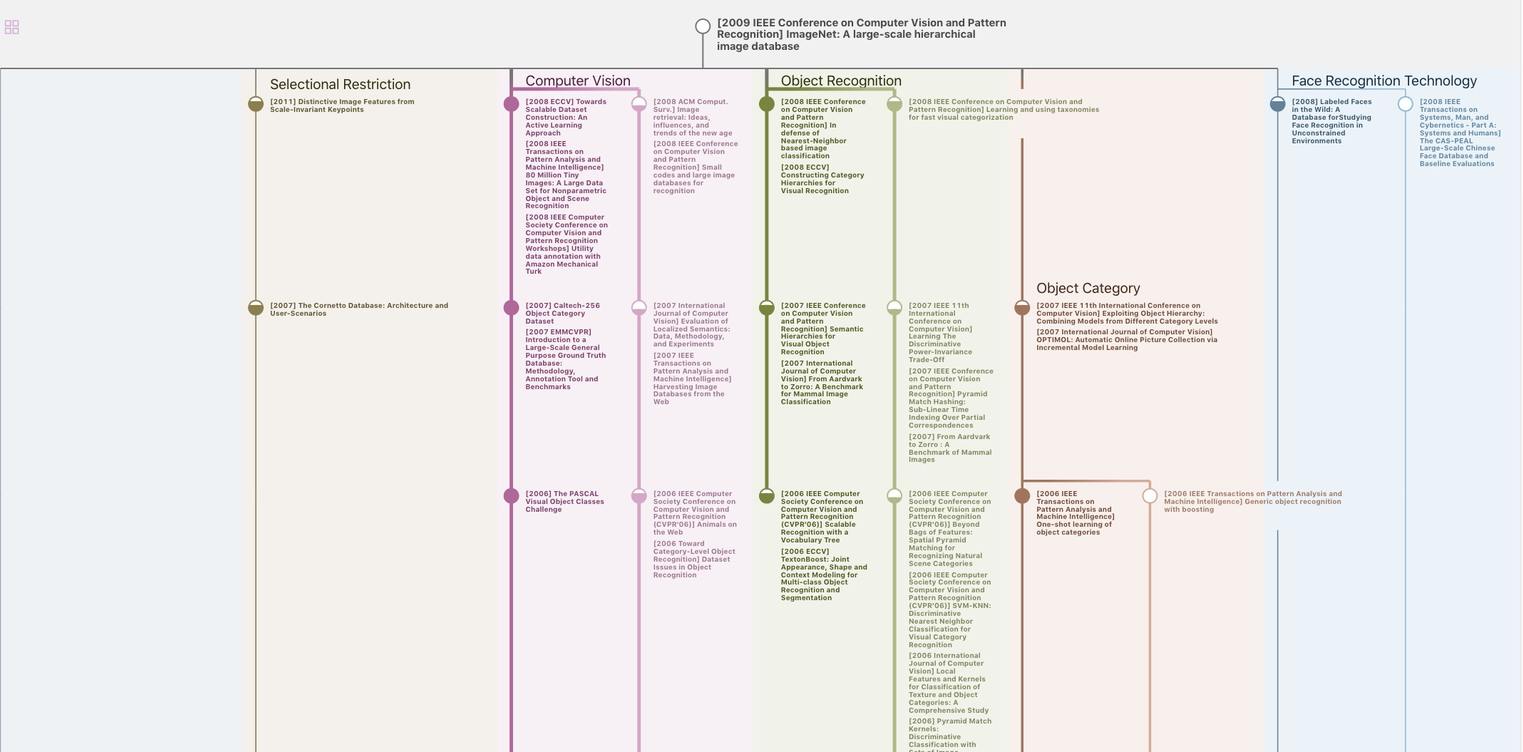
生成溯源树,研究论文发展脉络
Chat Paper
正在生成论文摘要