Factor graph fragmentization of expectation propagation
JOURNAL OF THE KOREAN STATISTICAL SOCIETY(2020)
摘要
Expectation propagation is a general approach to fast approximate inference for graphical models. The existing literature treats models separately when it comes to deriving and coding expectation propagation inference algorithms. This comes at the cost of similar, long-winded algebraic steps being repeated and slowing down algorithmic development. We demonstrate how factor graph fragmentization can overcome this impediment. This involves adoption of the message passing on a factor graph approach to expectation propagation and identification of factor graph sub-graphs, which we call fragments , that are common to wide classes of models. Key fragments and their corresponding messages are catalogued which means that their algebra does not need to be repeated. This allows compartmentalization of coding and efficient software development.
更多查看译文
关键词
Approximate Bayesian inference,Generalized linear mixed models,Graphical models,Kullback-Leibler projection,Message passing
AI 理解论文
溯源树
样例
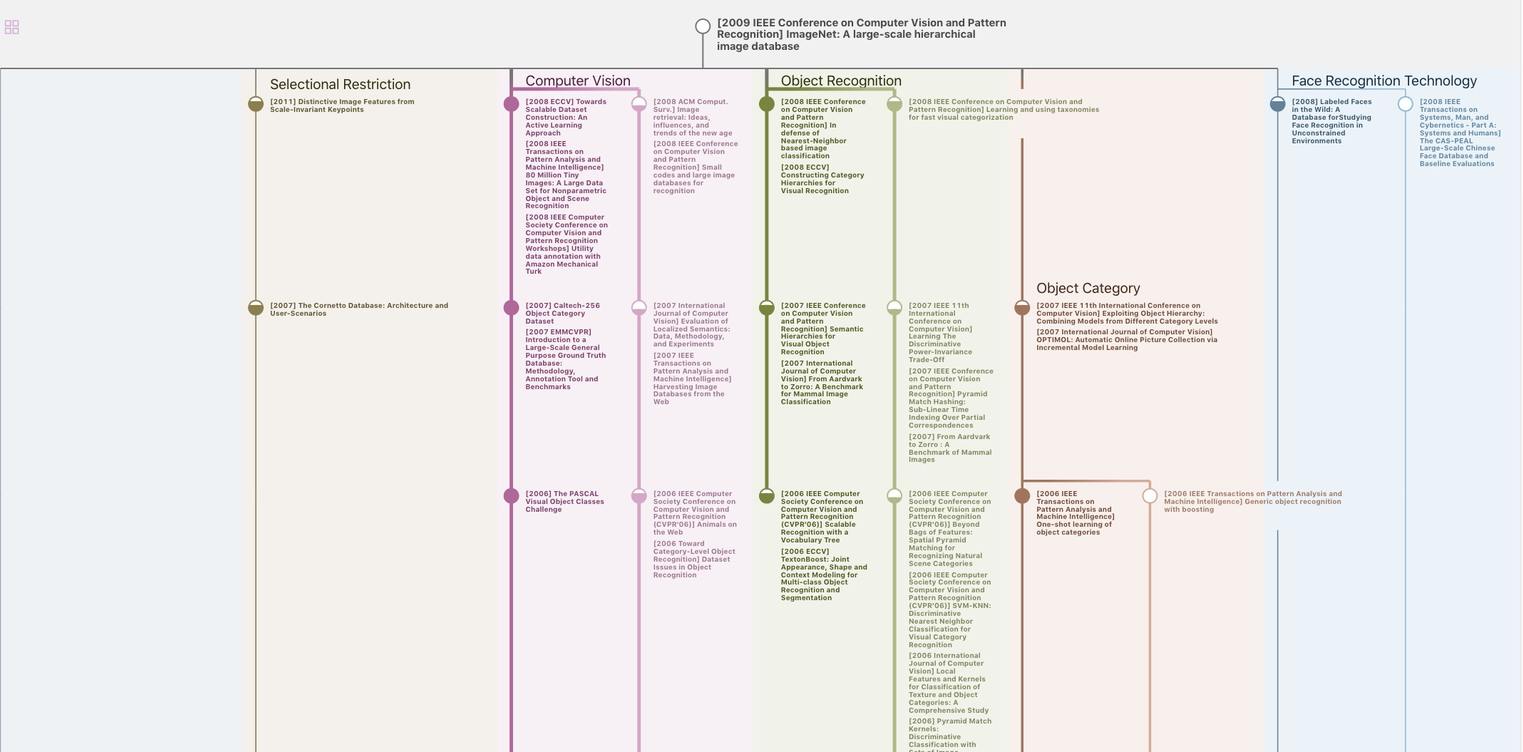
生成溯源树,研究论文发展脉络
Chat Paper
正在生成论文摘要