Blind image deblurring via hybrid deep priors modeling
Neurocomputing(2020)
摘要
•We propose a novel MAP inference scheme HDPM to integrate the explicit hand-crafted sparse prior and implicit trainable deep network architectures into underline image propagation for latent image estimation. Our HDPM indeed gains the advantages of both pre-designed task cues and data-dependent implicit deep priors. Such strategy can not only investigate the intrinsic image structure, but also provide textured guidance from date distribution for our sharp latent image propagation.•Our implicit deep prior learned from a network can be trained by paired noisy nature image and clear gradient image, which is easier to achieve than training end-to-end deblurring network architecture directly. Moreover, merging the implicit network and explicit experience into traditional MAP framework makes the propagation of latent image more robust than a pure network. It is thanks to the mutual promotion of the two components in our hybrid deep priors.•We extend our HDPM to non-uniform deblurring based on the geometrically consistent model from the discretization of motion blur. Our learnable implicit priors (network) can directly apply to this model without retraining. The experimental results demonstrate the better restoration in our extension.
更多查看译文
关键词
Image processing,Blind image deblurring,Kernel estimation,Hybrid deep priors,Residual network
AI 理解论文
溯源树
样例
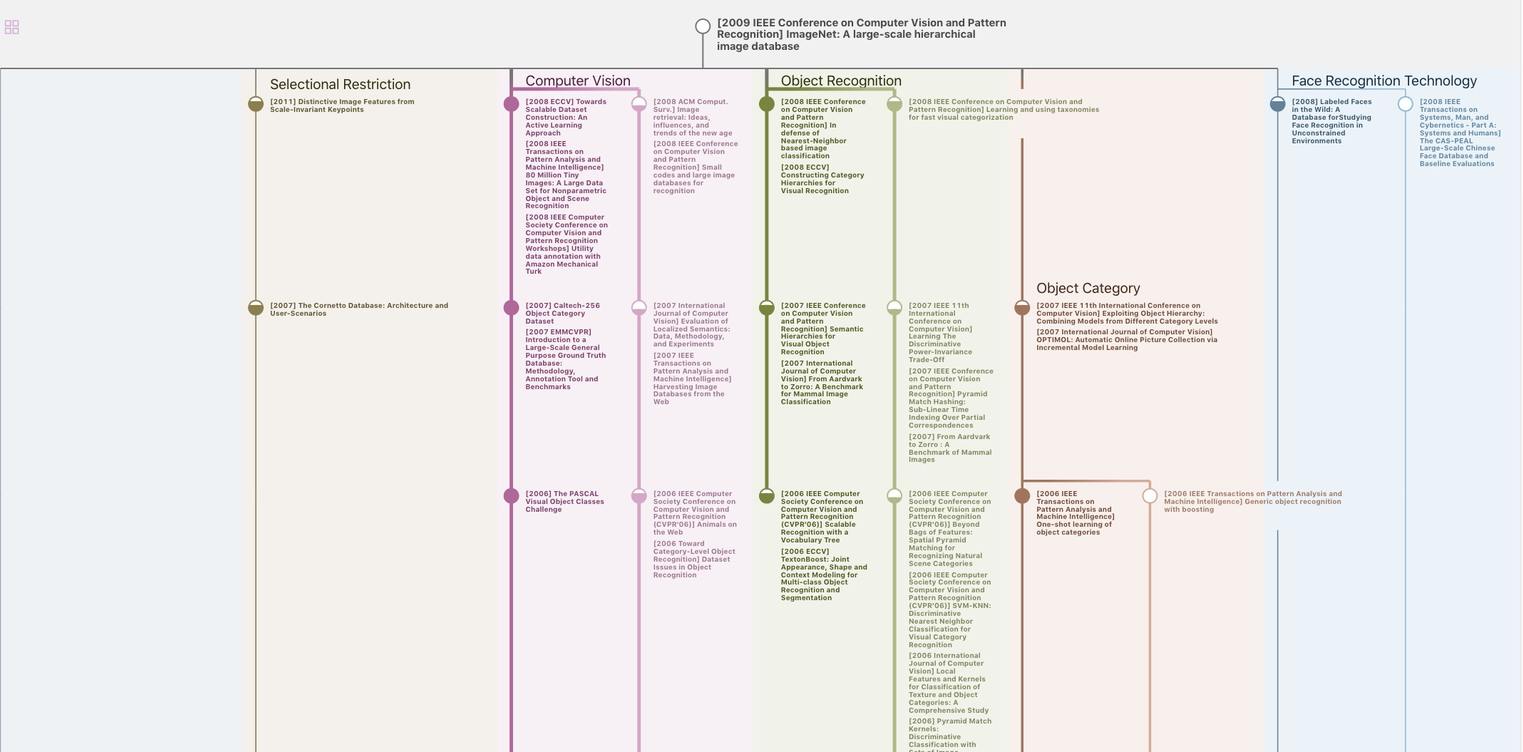
生成溯源树,研究论文发展脉络
Chat Paper
正在生成论文摘要