Joint Sketch-Attribute Learning For Fine-Grained Face Synthesis
MULTIMEDIA MODELING (MMM 2020), PT I(2020)
摘要
The photorealism of synthetic face images has been significantly improved by generative adversarial networks (GANs). Besides of the realism, more accurate control on the properties of face images. While sketches convey the desired shapes, attributes describe appearance. However, it remains challenging to jointly exploit sketches and attributes, which are in different modalities, to generate high-resolution photorealistic face images. In this paper, we propose a novel joint sketch-attribute learning approach to synthesize photo-realistic face images with conditional GANs. A hybrid generator is proposed to learn a unified embedding of shape from sketches and appearance from attributes for synthesizing images. We propose an attribute modulation module, which transfers user-preferred attributes to reinforce sketch representation with appearance details. Using the proposed approach, users could flexibly manipulate the desired shape and appearance of synthesized face images with fine-grained control. We conducted extensive experiments on the CelebA-HQ dataset [16]. The experimental results have demonstrated the effectiveness of the proposed approach.
更多查看译文
关键词
Conditional GANs, Sketch, Face attributes, Image synthesis, Joint learning
AI 理解论文
溯源树
样例
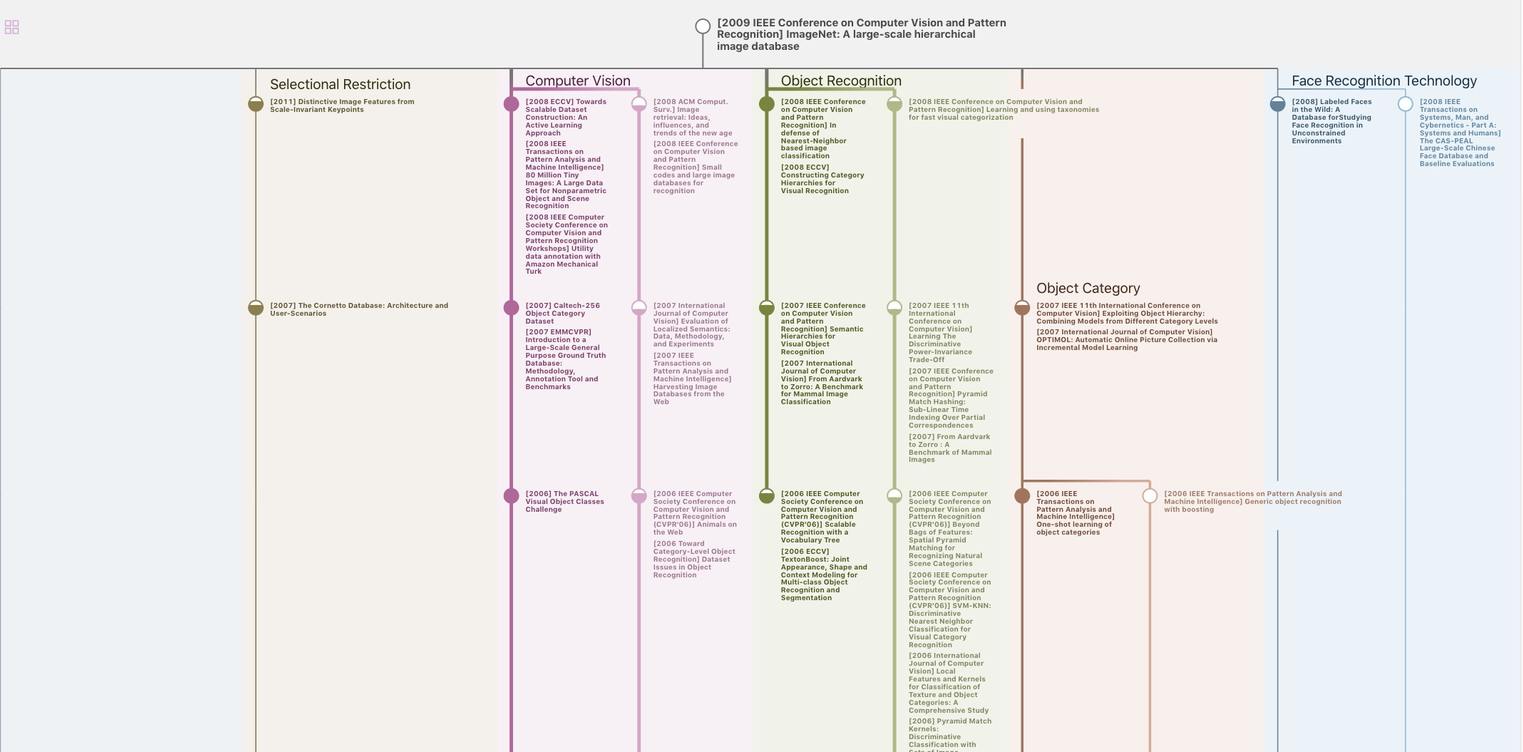
生成溯源树,研究论文发展脉络
Chat Paper
正在生成论文摘要