Adaptive Regularized Semi-Supervised Clustering Ensemble
IEEE ACCESS(2020)
摘要
Although semi-supervised clustering ensemble methods have achieved satisfactory performance, they fail to effectively utilize the constrained knowledge such as cannot-link and must-link when generating diverse ensemble members. In addition, they ignore negative effects brought about by redundancies and noisy data. To address the above shortcomings, in this paper we propose an approach to combine multiple semi-supervised clustering solutions via adaptively regularizing the weights of clustering ensemble members, which is referred to as ARSCE. First, we generate a series of feature subspaces by randomly selecting feature without replacement to avoid the scenario where there are two identical feature subspaces. Second, we conduct feature transformation on the above obtained feature subspaces while considering the pairwise constraints to find new clustering-friendly spaces, where clustering methods are exploited to generate various clustering solutions. Finally, we design a novel fusion strategy to integrate multiple clustering solutions into a unified clustering partition, where weights are designated for each clustering ensemble member. Extensive experiments are conducted on multiple real-world benchmarks, and experimental results demonstrate the effectiveness and superiority of our proposed method ARSCE over other counterparts.
更多查看译文
关键词
Clustering ensemble, semi-supervised clustering, constraint selection, clustering fusion
AI 理解论文
溯源树
样例
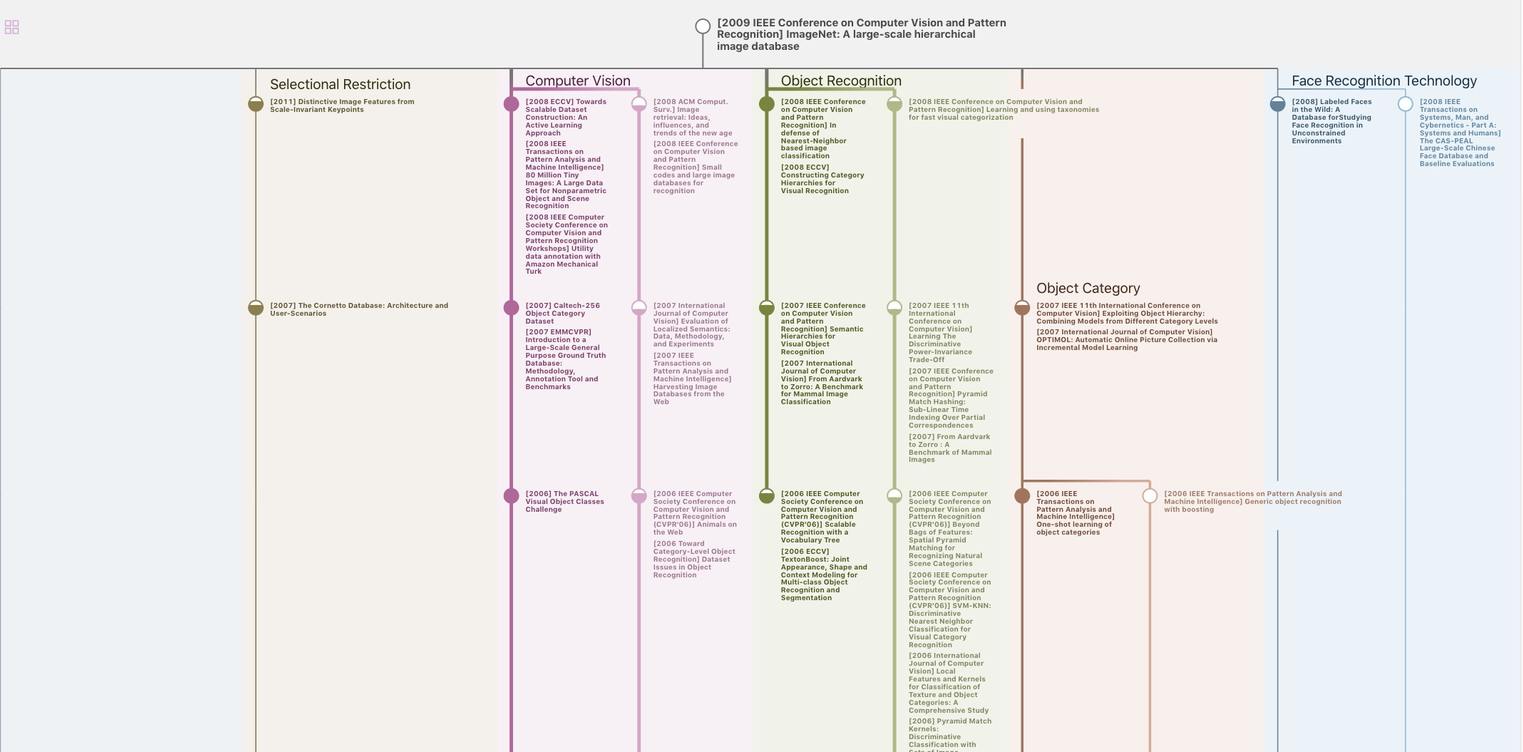
生成溯源树,研究论文发展脉络
Chat Paper
正在生成论文摘要