Robust Motion Blur Kernel Estimation by Kernel Continuity Prior
IEEE ACCESS(2020)
摘要
The accurate kernel estimation is key to the blind motion deblurring. Many previous methods depend on the image regularization to recover strong edges in the observed image for kernel estimation. However, the estimated kernel will be degraded when recovered strong edges are less accurate, especially in images full of small-scale edges. Different from previous methods, we focus on the kernel regularization. Inspired by the fact that the blur kernel is highly related to the continuous camera motion trajectory during the image capturing, we propose to encourage the continuity of the kernel through a kernel prior. The proposed prior measures the continuity of each element in the kernel and generates a continuity map. By encouraging the sparsity of the map using L-0 norm, discontinuous kernel elements are suppressed. Since the model with the proposed prior is non-convex and non-linear, an approximation method is proposed to minimize the cost function efficiently. Numerous experimental results show that our method outperforms state-of-the-art methods on both the normal and challenging cases. Moreover, the proposed prior is able to further improve the performance of existing MAP-based methods.
更多查看译文
关键词
Image deblurring,robust blur Kernel estimation,small-scale edges,Kernel continuity
AI 理解论文
溯源树
样例
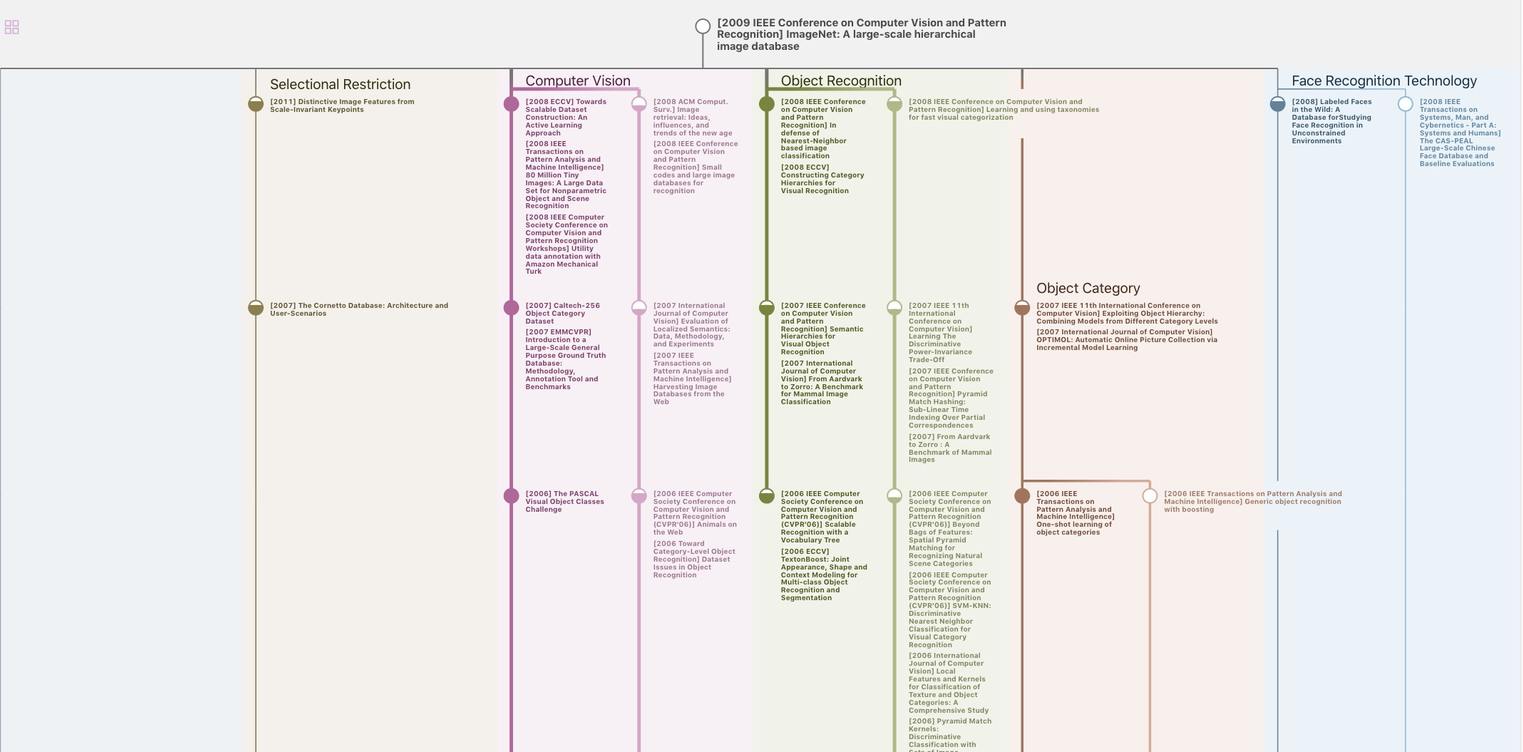
生成溯源树,研究论文发展脉络
Chat Paper
正在生成论文摘要