Conditional restricted Boltzmann machine for item recommendation
Neurocomputing(2020)
摘要
Recommender systems provide an excellent solution to the issue of information overload by generating item recommendation from a huge collection of items based on users’ preferences. In terms of modeling users’ rating data, existing methods are mainly neighborhood- and factorization-based methods, most of which are rating oriented. Among network-based methods, the restricted Boltzmann machine (RBM) model is also applied to rating prediction tasks. However, item recommendation tasks play a more important role in the real world, due to the large item space as well as users’ limited attention. In this paper, we treat users’ rating behaviors from a new perspective and study the effectiveness of conditional RBM (CRBM) in modeling users’ rating preferences for top-k recommendation. We conduct extensive empirical studies on four real-world datasets and find that our proposed CRBM-IR is very competitive in exploiting users’ explicit rating feedback in comparison with the closely related works.
更多查看译文
关键词
Conditional restricted Boltzmann machine,Item recommendation,Explicit feedback
AI 理解论文
溯源树
样例
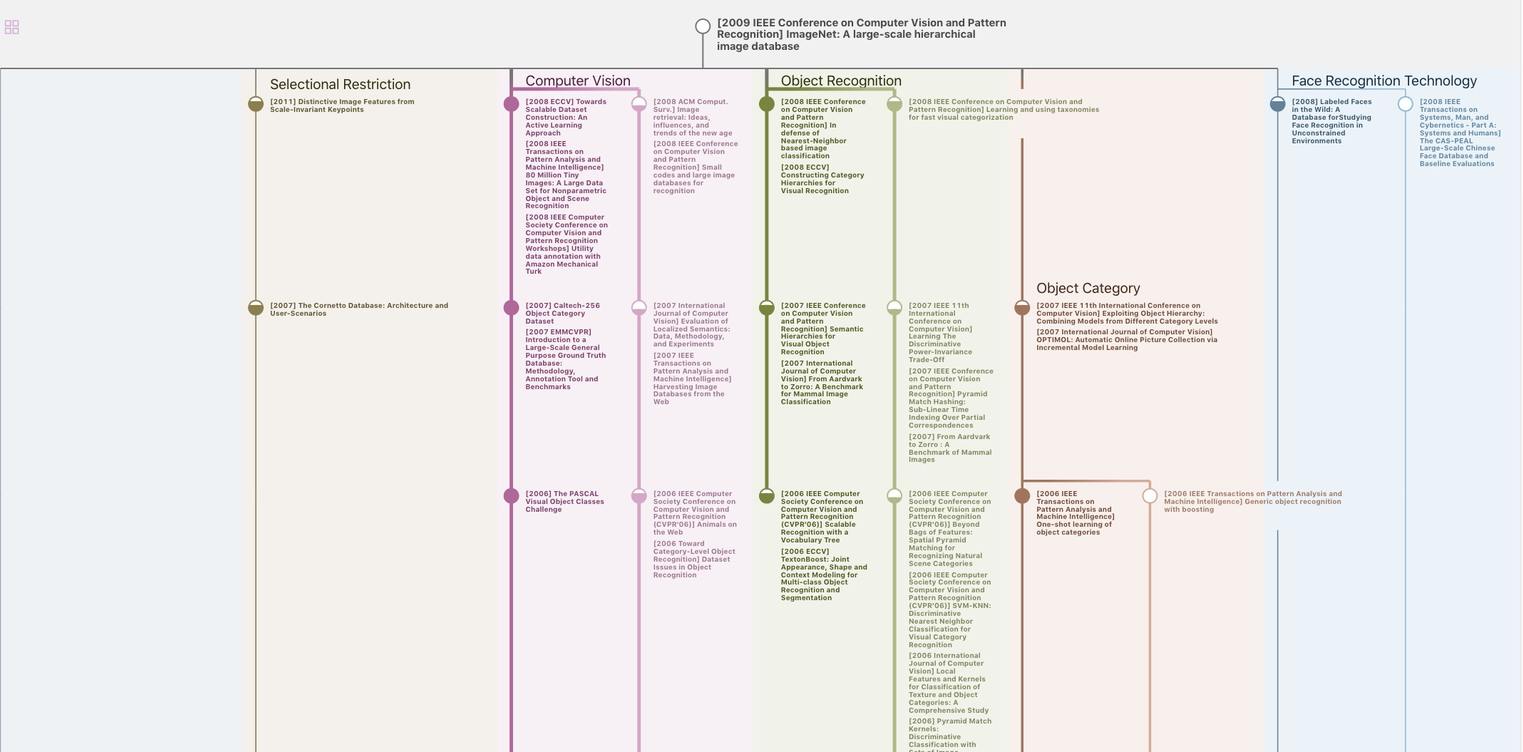
生成溯源树,研究论文发展脉络
Chat Paper
正在生成论文摘要