Meta Transfer Learning For Adaptive Vehicle Tracking In Uav Videos
MULTIMEDIA MODELING (MMM 2020), PT I(2020)
摘要
The vehicle tracking in UAV videos is still under-explored with the deep learning methods due to the lack of well labeled datasets. The challenges mainly come from the fact that the UAV view has much wider and changeable landscapes, which hinders the labeling task. In this paper, we propose a meta transfer learning method for adaptive vehicle tracking in UAV videos (MTAVT), which transfers the common features across landscapes, so that it can avoid over-fitting with the limited scale of dataset. Our MTAVT consists of two critical components: a meta learner and a transfer learner. Specifically, meta-learner is employed to adaptively learn the models to extract the sharing features between ground and drone views. The transfer learner is used to learn the domain-shifted features from ground-view to drone-view datasets by optimizing the ground-view models. We further seamlessly incorporate an exemplar-memory curriculum into meta learning by leveraging the memorized models, which serves as the training guidance for sequential sampling. Hence, this curriculum can enforce the meta learner to adapt to the new sequences in the drone-view datasets without losing the previous learned knowledge. Meanwhile, we simplify and stabilize the higher-order gradient training criteria for meta learning by exploring curriculum learning in multiple stages with various domains. We conduct extensive experiments and ablation studies on four public benchmarks and an evaluation dataset from YouTube (to release soon). All the experiments demonstrate that, our MTAVT has superior advantages over state-of-the-art methods in terms of accuracy, robustness, and versatility.
更多查看译文
关键词
Meta learner, Transfer learner, Exemplar-memory curriculum, UAV tracking
AI 理解论文
溯源树
样例
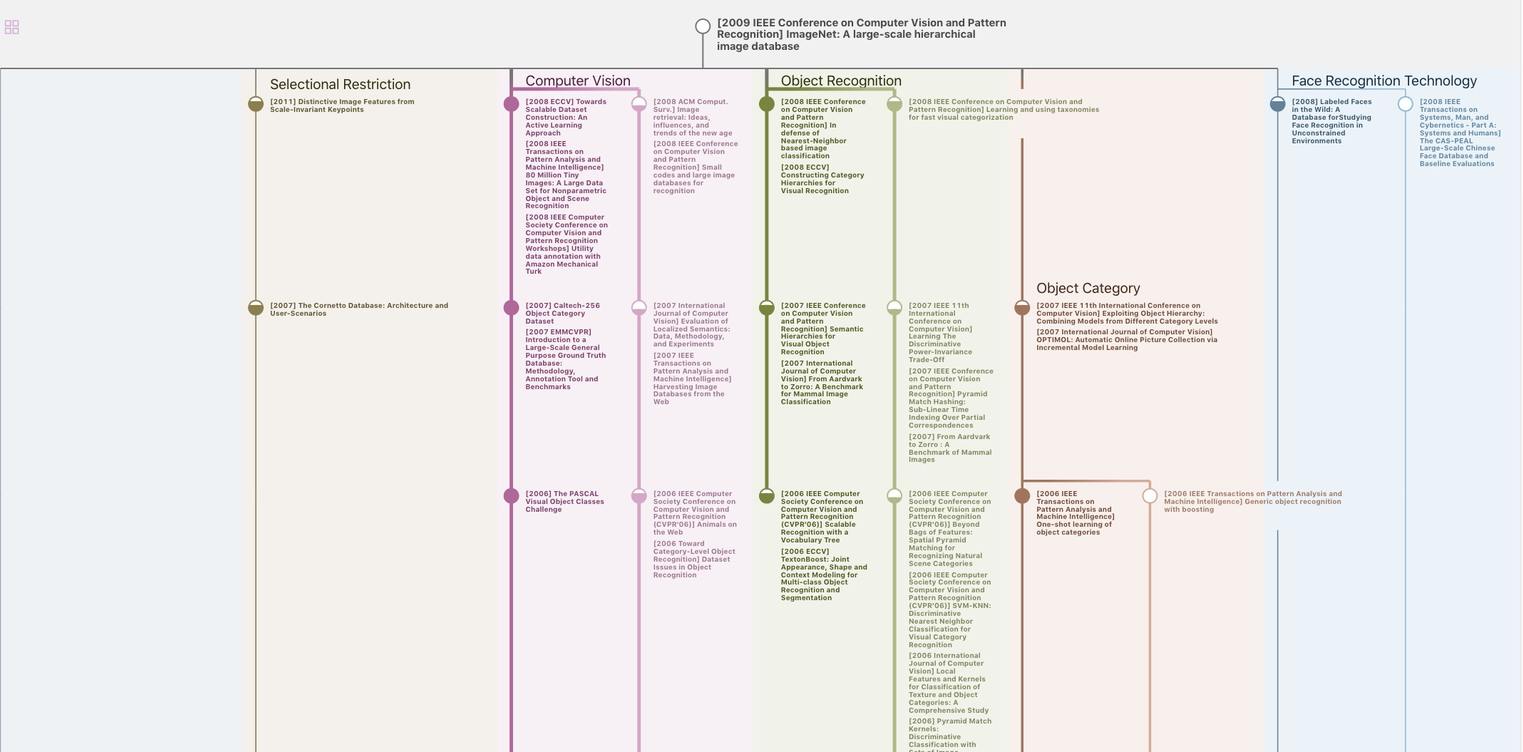
生成溯源树,研究论文发展脉络
Chat Paper
正在生成论文摘要