Earthquake-Induced Building Damage Mapping Based On Multi-Task Deep Learning Framework
IEEE ACCESS(2019)
摘要
Earthquake-induced building damage can be directly devastating, leading to a large population loss and massive property damage with the high rate of globalization and urbanization. In case an earthquake takes place, the location and the scale of collapsed buildings are vital for rescuers to take effective aid measures immediately to reduce casualties and economic loss. The development in earth observation using high spatial resolution images makes it possible to recognize damaged and intact buildings. However, the methods proposed are most limited to specific cases with a limited number of buildings in pure background objects. In this paper, a multi-task deep learning framework is proposed to map damaged and intact buildings from large-scale very high spatial resolution images. The images used in our study have complicated background objects, which share similar spectral and textual characteristics with buildings. Therefore, we built a main task model to detect buildings in good shape and damaged conditions, and an extra task model to supplement the main task by semantically segmenting the input image into multiple ground objects in the multi-task framework. It is an end-to-end framework, comprising residual network and pyramid pooling module, aiming to extract multi-scale contextual features. The proposed framework is trained and evaluated in different parts of western Haiti, which gets affected by the earthquake in 2010. Besides, the results demonstrate that multi-task deep learning framework is encouraging to map earthquake-induced building damage in complicated background.
更多查看译文
关键词
Building damage mapping, multi-task learning, deep learning framework
AI 理解论文
溯源树
样例
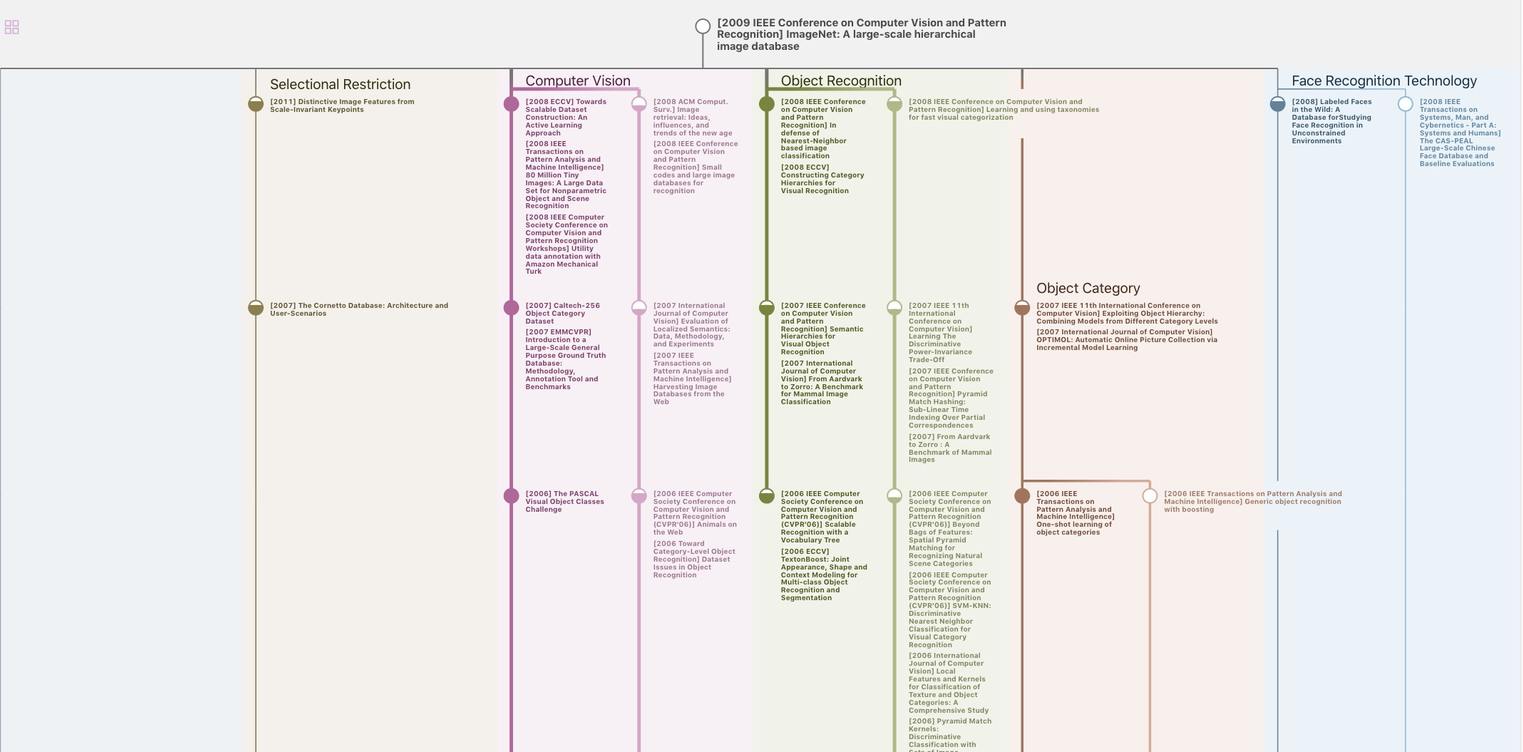
生成溯源树,研究论文发展脉络
Chat Paper
正在生成论文摘要