Scalable Person Re-Identification by Harmonious Attention
International Journal of Computer Vision(2019)
摘要
Existing person re-identification (re-id) deep learning methods rely heavily on the utilisation of large and computationally expensive convolutional neural networks. They are therefore not scalable to large scale re-id deployment scenarios with the need of processing a large amount of surveillance video data, due to the lengthy inference process with high computing costs. In this work, we address this limitation via jointly learning re-id attention selection. Specifically, we formulate a novel harmonious attention network (HAN) framework to jointly learn soft pixel attention and hard region attention alongside simultaneous deep feature representation learning, particularly enabling more discriminative re-id matching by efficient networks with more scalable model inference and feature matching. Extensive evaluations validate the cost-effectiveness superiority of the proposed HAN approach for person re-id against a wide variety of state-of-the-art methods on four large benchmark datasets: CUHK03, Market-1501, DukeMTMC, and MSMT17.
更多查看译文
关键词
Person re-identification, Scalable search, Compact model, Attention learning, Local and global representation learning
AI 理解论文
溯源树
样例
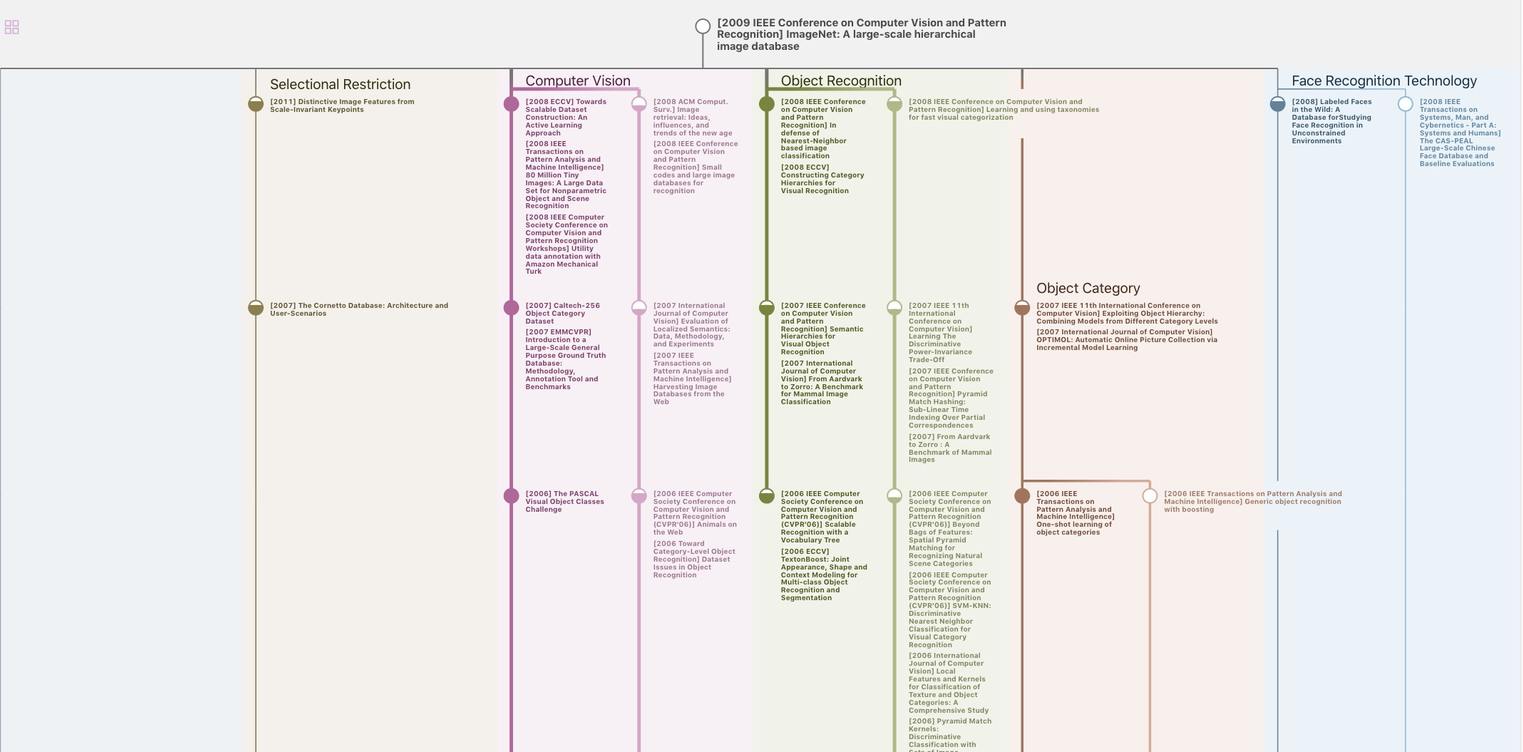
生成溯源树,研究论文发展脉络
Chat Paper
正在生成论文摘要