Heterogeneous Defect Prediction Based on Transfer Learning to Handle Extreme Imbalance
APPLIED SCIENCES-BASEL(2020)
摘要
Software systems are now ubiquitous and are used every day for automation purposes in personal and enterprise applications; they are also essential to many safety-critical and mission-critical systems, e.g., air traffic control systems, autonomous cars, and Supervisory Control And Data Acquisition (SCADA) systems. With the availability of massive storage capabilities, high speed Internet, and the advent of Internet of Things devices, modern software systems are growing in both size and complexity. Maintaining a high quality of such complex systems while manually keeping the error rate at a minimum is a challenge. This paper proposed a heterogeneous defect prediction method considering class extreme imbalance problem in real software datasets. In the first stage, Sampling with the Majority method (SWIM) based on Mahalanobis Distance is used to balance the dataset to reduce the influence of minority samples in defect data. Due to the negative impact of uncorrelated features on the classification algorithm, the second stage uses ensemble learning and joint similarity measurement to select the most relevant and representative features between the source project and the target project. The third phase realizes the transfer learning from the source project to the target project in the Grassmann manifold space. Our experiments, conducted using nine projects of three public domain software defect libraries and compared with four existing advanced methods to verify the effectiveness of the proposed method in this paper. The experimental results indicate that the proposed method is more accurate in terms of Area under curve (AUC).
更多查看译文
关键词
heterogeneous defect prediction,class extreme imbalance,transfer learning,Grassmann manifold
AI 理解论文
溯源树
样例
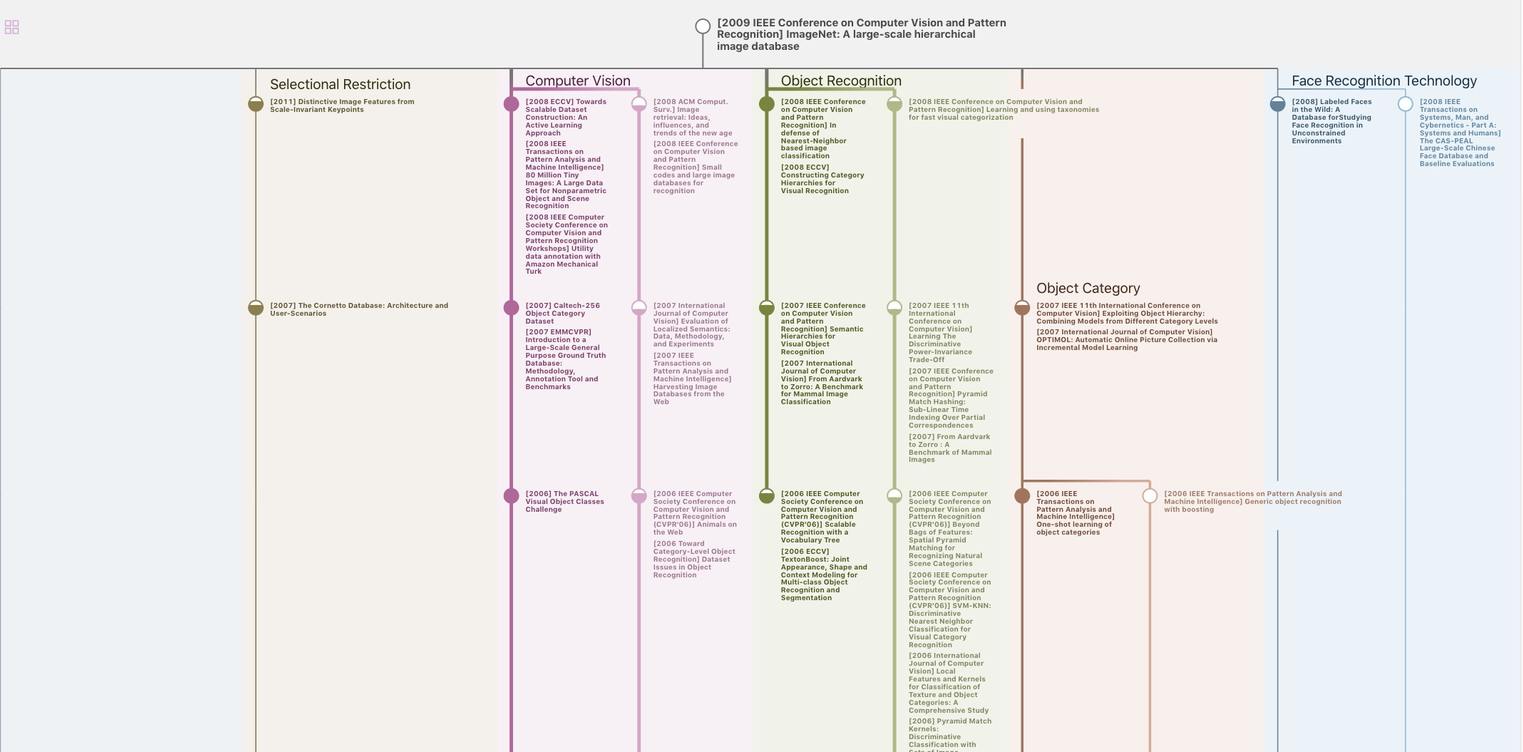
生成溯源树,研究论文发展脉络
Chat Paper
正在生成论文摘要