Early Gear Pitting Fault Diagnosis Based on Bi-directional LSTM
2019 PROGNOSTICS AND SYSTEM HEALTH MANAGEMENT CONFERENCE (PHM-QINGDAO)(2019)
摘要
The early gear pitting fault diagnosis has received much attention in the industry. In recent decades, with the popularity growth of artificial neural network, researchers have applied deep learning methods to figure out early gear pitting faults. However, the classical fault diagnosis methods usually use deep neural networks according to the time sequence of the collected signals. In this case, the feature extraction in the direction of the inverse time-domain signals is usually ignored. Aimed at overcoming this shortage, ground on a traditional Long Short Term Memory (LSTM) network, this paper proposes a Bidirectional LSTM (Bi-LSTM) to construct a fault diagnosis model of early gear pitting using raw vibration signals. Using the Bi-LSTM network, feature extraction of the vibrational signals in both directions is simultaneously carried out to evaluate the degree of the early gear pitting faults to better extract the gear pitting characteristics from the raw vibration signals of the gear. Through the analysis of the experimental data, compared with the traditional LSTM model, the Bi-directional LSTM has a classification accuracy of over 96% for early gear pitting fault diagnosis, which is an increase of 4.1%.
更多查看译文
关键词
artificial neural network, gear, vibration signal, long short term memory, gear pitting diagnosis
AI 理解论文
溯源树
样例
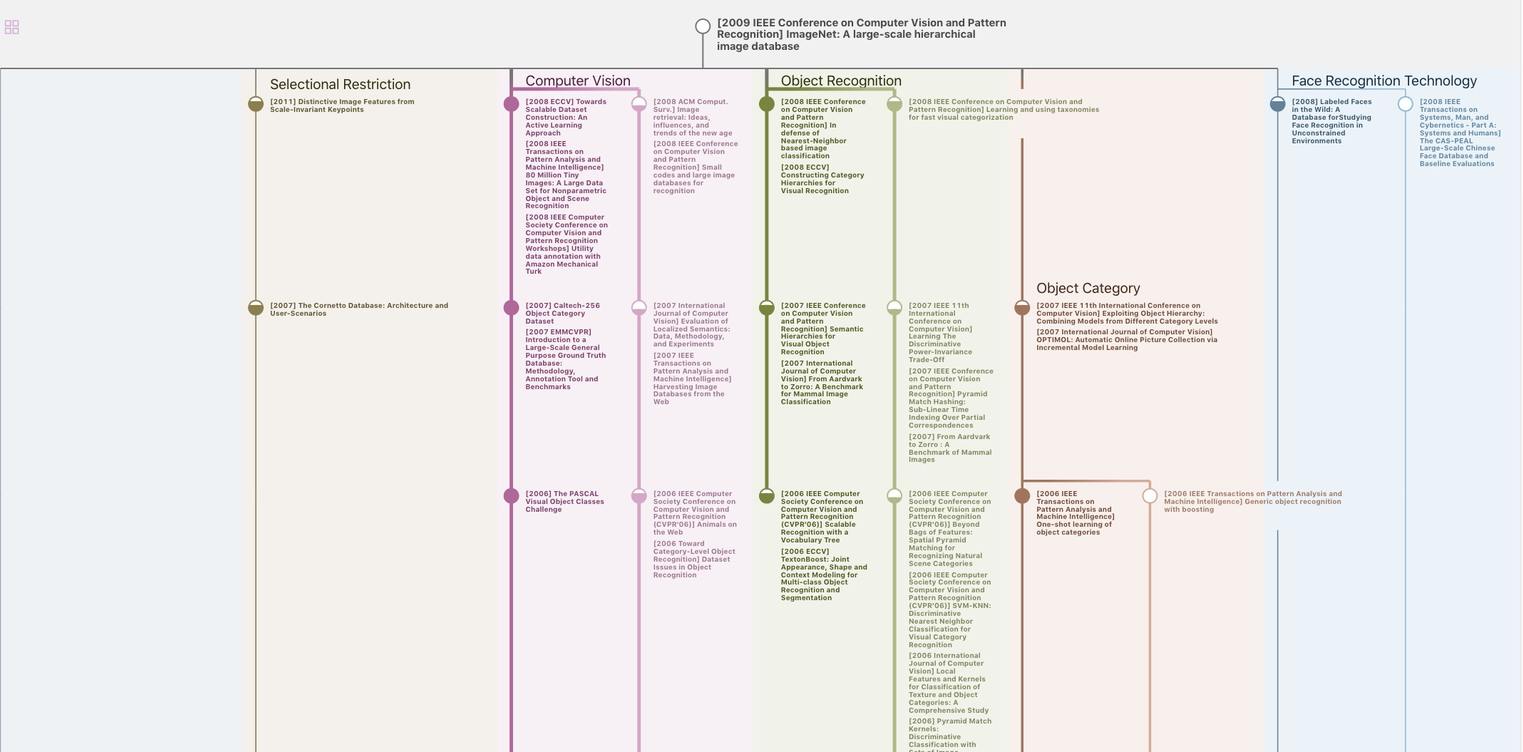
生成溯源树,研究论文发展脉络
Chat Paper
正在生成论文摘要