Smart Machine Maintenance Enabled by a Condition Monitoring Living Lab
IFAC-PapersOnLine(2019)
摘要
A key barrier in the industrial adoption of condition monitoring is the lack of large and reliable data sets about the full lifetime of bearings in machines. This data is useful for model training as well as for validation purposes. This paper demonstrates how a living lab, consisting of 7 identical drive train sub-systems, can enable smart machine maintenance and support the adoption of condition monitoring technologies in the industry. The living lab allows to perform accelerated lifetime tests of bearings and to speed up the process of collecting large amounts of data about degrading bearings. It is shown that the data can be used to benchmark diagnostic algorithms. Three methods are compared: a data driven approach developed by the Linz Center of Mechatronics (LCM), a diagnostic method of Flanders Make (FM) and an approach developed by the Center for Intelligent Maintenance Systems (IMS). It is concluded that the method of IMS and FM, employing bearing specific features, tend to be slightly more sensitive to early detect bearing faults than the data driven approach employed by LCM. On the contrary, the method of LCM does not require specific system knowledge and is not limited to bearing monitoring only. The method is more widely applicable to fault monitoring problems. Besides a benchmark study, the living lab can also be used to develop, test and validate new diagnostic and prognostic methods. In this way, the living lab provides opportunities to enable a wider adoption of condition monitoring technologies in industry.
更多查看译文
关键词
predictive maintenance,condition monitoring,benchmarking,fleet monitoring,bearings,accelerated lifetime
AI 理解论文
溯源树
样例
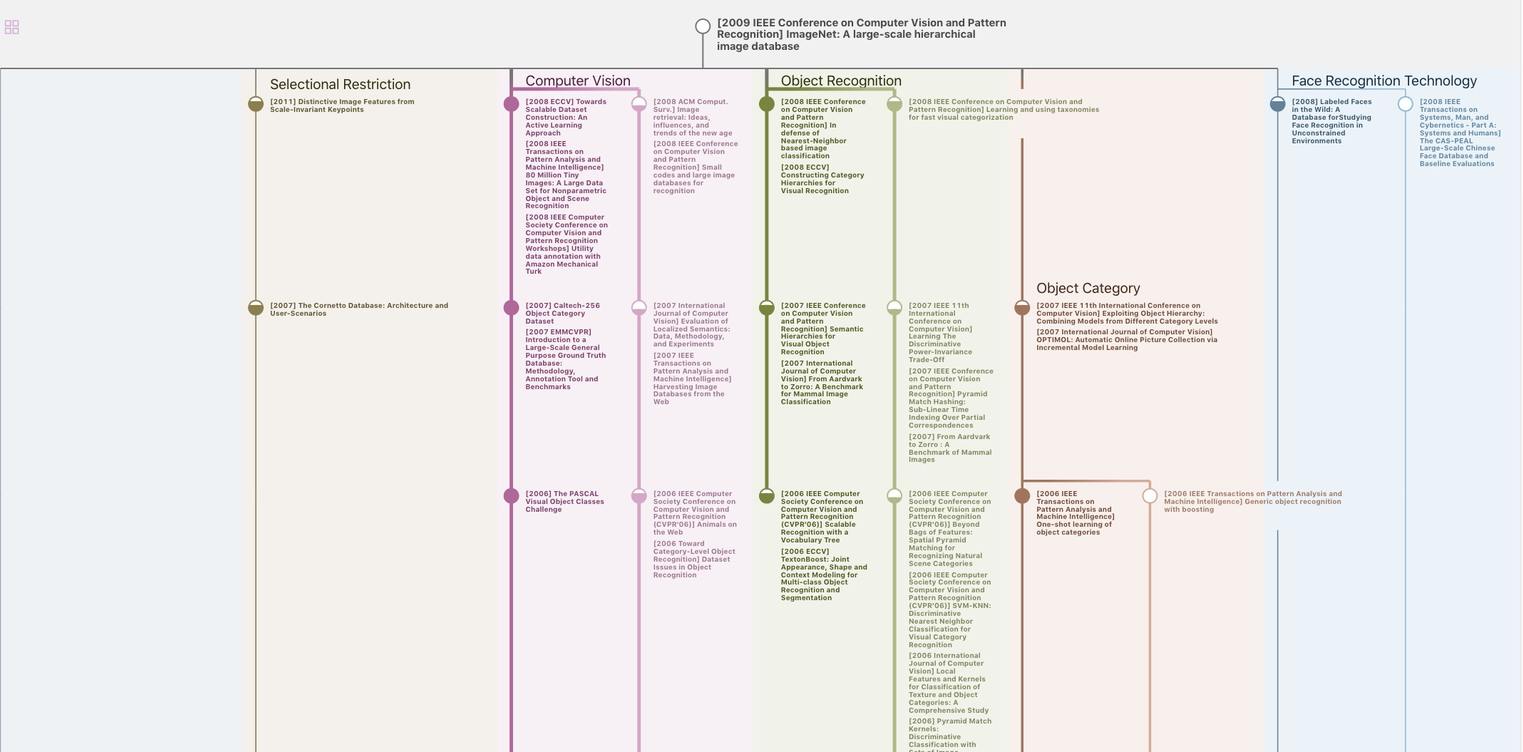
生成溯源树,研究论文发展脉络
Chat Paper
正在生成论文摘要