Machine Learning Prediction of Radiofrequency Thermal Ablation Efficacy: A New Option to Optimize Thyroid Nodule Selection.
EUROPEAN THYROID JOURNAL(2019)
摘要
BACKGROUND:Radiofrequency (RF) is a therapeutic modality for reducing the volume of large benign thyroid nodules. If thermal therapies are interpreted as an alternative strategy to surgery, critical issues in their use are represented by the extent of nodule reduction and by the durability of nodule reduction over a long period of time.
OBJECTIVE:To assess the ability of machine learning to discriminate nodules with volume reduction rate (VRR) < or ≥50% at 12 months following RF treatment.
METHODS:A machine learning model was trained with a dataset of 402 cytologically benign thyroid nodules subjected to RF at six Italian Institutions. The model was trained with the following variables: baseline nodule volume, echostructure, macrocalcalcifications, vascularity, and 12-month VRR.
RESULTS:After training, the model could distinguish between nodules having VRR <50% from those having VRR ≥50% in 85% of cases (accuracy: 0.85; 95% confidence interval [CI]: 0.80-0.90; sensitivity: 0.70; 95% CI: 0.62-0.75; specificity: 0.99; 95% CI: 0.98-1.0; positive predictive value: 0.95; 95% CI: 0.92-0.98; negative predictive value: 0.95; 95% CI: 0.92-0.98).
CONCLUSIONS:This study demonstrates that a machine learning model can reliably identify those nodules that will have VRR < or ≥50% at 12 months after one RF treatment session. Predicting which nodules will be poor or good responders represents valuable data that may help physicians and patients decide on the best treatment option between thermal ablation and surgery or in predicting if more than one session might be necessary to obtain a significant volume reduction.
更多查看译文
关键词
Thyroid,Nodules,Radiofrequency,Laser,Thermal ablation,Machine learning,Artificial Intelligence
AI 理解论文
溯源树
样例
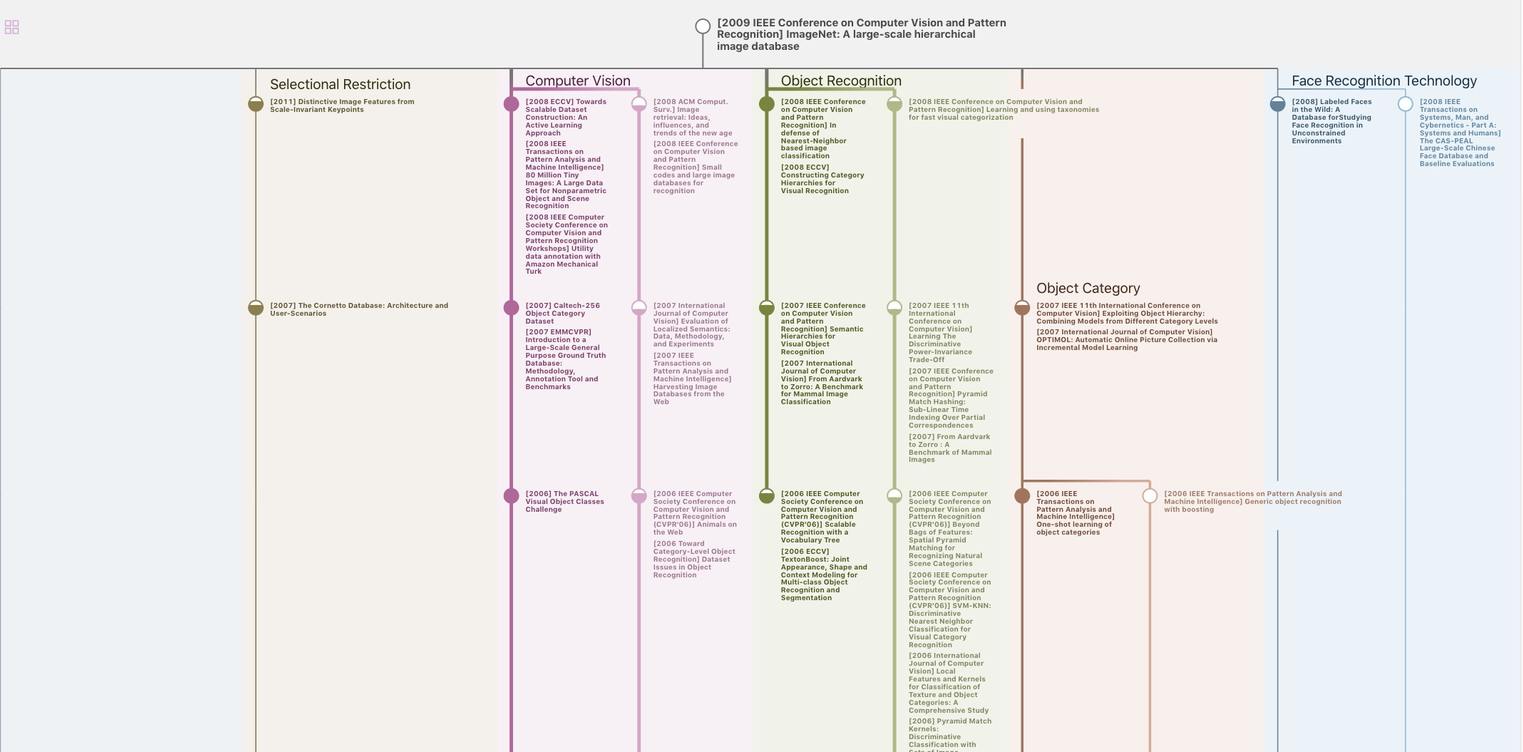
生成溯源树,研究论文发展脉络
Chat Paper
正在生成论文摘要