Novel Contradiction Resolution in Fixed Point Transformation-based Adaptive Control
2018 IEEE 18th International Symposium on Computational Intelligence and Informatics (CINTI)(2018)
摘要
In the control practice the problem of “underactu-ation” often arises: for controlling the state of a multivariable system the number of the independently applicable control signals is smaller than that of the components of the state variables. Then either certain state components can propagate in time without direct control while the others are precisely controlled, or some compromise has to be elaborated on the basis of which the control forces are “distributed” to keep each variable under partial and imprecise control. Normally, only imprecise and/or incomplete system models are available. Traditionally a compromise is achieved by the use of optimal controllers in which the often contradictory requirements are represented as nonnegative contributions to a cost function that is minimized under the constraints representing the available approximate dynamic model. The heuristic “Receding Horizon Controller” (RHC) uses “Nonlinear Programming” (NP): the optimum is found over a discrete grid by the use of Lagrange's Reduced Gradient Method. For dealing with imprecise modeling and unknown external disturbances the control forces are calculated for and are exerted only over a “short” horizon, then, after observing the actual state by measurements, a new horizon is designed, etc. The adaptive controllers, that normally are designed on the basis of Lyapunov's 2nd method, do not allow simple integration with the optimal controllers. The design of optimal and adaptive controllers is a challenge. To take up this challenge in Adaptive Control Lyapunov's 2nd method was replaced by a “Fixed Point Transformation” (FPT)-based approach. At first the control problem is transformed into finding the fixed point of a contractive map over a Banach Space, then it is solved via iteration. With this approach the contradiction resolution was tackled by time-sharing instead of minimizing a cost function. Alternatively, the minimization of a cost function was tackled by FPT simply by driving the gradient of the Auxiliary Function directly to zero. In the present paper, to our best knowledge in the first time, a novel cost function-free adaptive solution is suggested for underactuated systems in which the compromise between the various requirements is “built in” into the FPT by interpretation. This approach is as heuristic as the RHC, and in general no good convergence can be guaranteed. As an application example the control of a 2 degree of freedom system, the FiztHugh Neuron Model is considered via computer simulations. The possibilities and the limitations of the suggested method are discussed.
更多查看译文
关键词
Adaptive Control,Optimal Control,Fixed Point Transformations,Underactuated Systems,FiztHugh Neuron
AI 理解论文
溯源树
样例
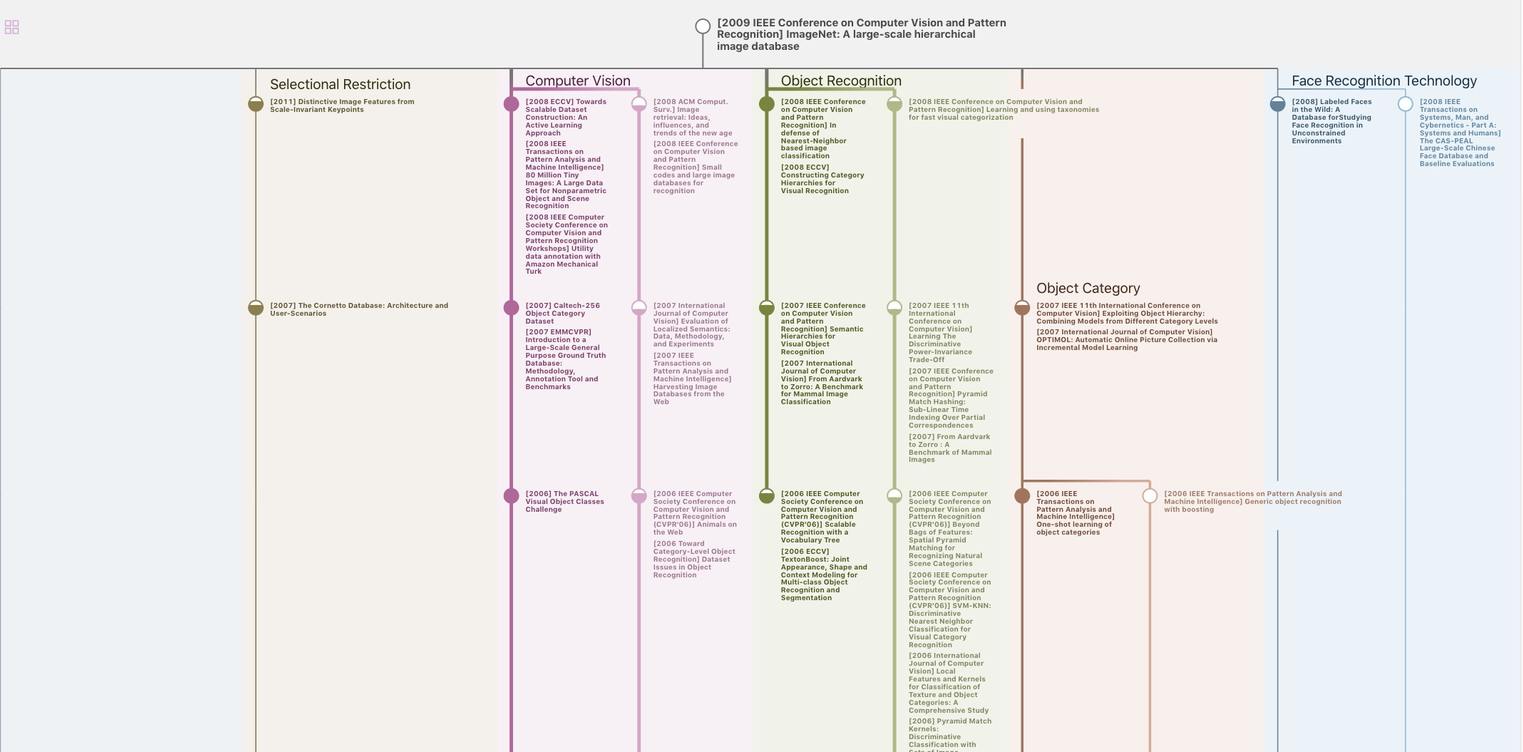
生成溯源树,研究论文发展脉络
Chat Paper
正在生成论文摘要