One-Step Deep Learning Approach To Ultrasound Image Formation And Image Segmentation With A Fully Convolutional Neural Network
2019 IEEE INTERNATIONAL ULTRASONICS SYMPOSIUM (IUS)(2019)
摘要
Single plane wave imaging is well-suited to high frame rate imaging tasks (e.g., ultrasound based robotic tracking). However, suboptimal image quality is obtained when limited to a single plane wave transmission. To address this challenge, we propose to train deep neural networks (DNNs) as an alternative to delay-and-sum beamforming followed by segmentation. Our overall goal is to extract information directly from raw channel data prior to the application of time delays and to simultaneously generate both a segmentation map and an ultrasound B-mode image of anechoic cysts surrounded by tissue. A network trained with 17,676 Field II simulations was tested with both simulated and experimental phantom data sets that were not included during training (9,108 and 320 images, respectively). DNN results from simulated and phantom test sets produced similar dice similarity coefficients (DSC), contrast, tissue signal-to-noise ratios (SNR), and generalized contrast-to-noise ratios (GCNR). Similarity is reported as the mean +/- standard deviation of these metrics for simulated and experimental test set results as follows: 0.92 +/- 0:13 and 0.92 +/- 0.03 DSC, respectively; +/- 6:41 dB and +/- 3:81 dB contrast, respectively; 3.78 +/- 1.08 and 4.53 +/- 1.23 SNR, respectively; and 1.00 +/- 0.01 and 1.00 +/- 0.01 GCNR, respectively. Thus, the DNNs successfully transferred feature representations learned from simulated data to experimental phantom data, highlighting the promise of this novel alternative to traditional ultrasound information extraction.
更多查看译文
关键词
Deep Learning, Neural Network, Fully Convolutional Neural Network, Beamforming, Image Segmentation
AI 理解论文
溯源树
样例
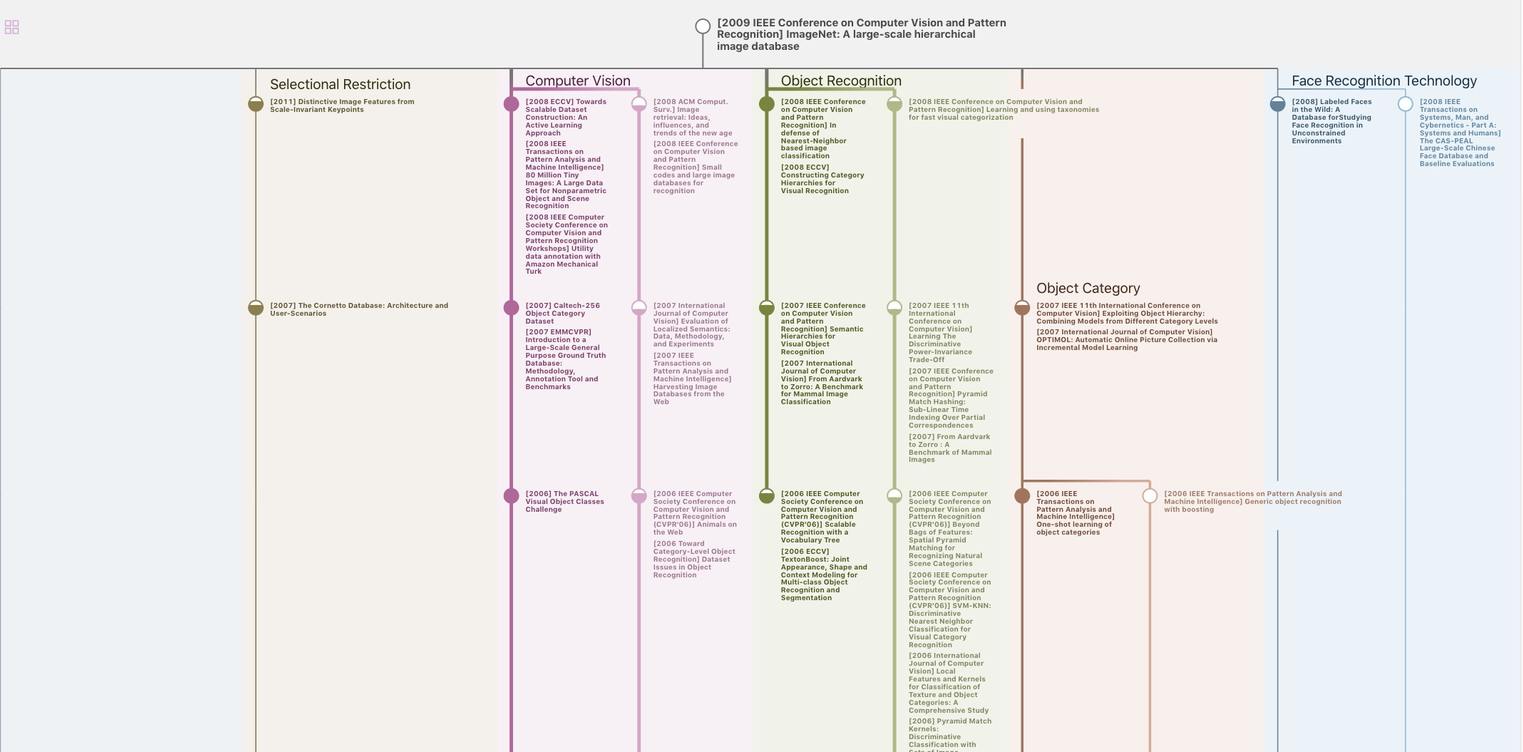
生成溯源树,研究论文发展脉络
Chat Paper
正在生成论文摘要