The Shape of Data: Intrinsic Distance for Data Distributions
ICLR(2020)
摘要
The ability to represent and compare machine learning models is crucial in order to quantify subtle model changes, evaluate generative models, and gather insights on neural network architectures. Existing techniques for comparing data distributions focus on global data properties such as mean and covariance; in that sense, they are extrinsic and uni-scale. We develop a first-of-its-kind intrinsic and multi-scale method for characterizing and comparing data manifolds, using a lower-bound of the spectral variant of the Gromov-Wasserstein inter-manifold distance, which compares all data moments. In a thorough experimental study, we demonstrate that our method effectively discerns the structure of data manifolds even on unaligned data of different dimensionalities; moreover, we showcase its efficacy in evaluating the quality of generative models.
更多查看译文
关键词
Deep Learning, Generative Models, Nonlinear Dimensionality Reduction, Manifold Learning, Similarity and Distance Learning, Spectral Methods
AI 理解论文
溯源树
样例
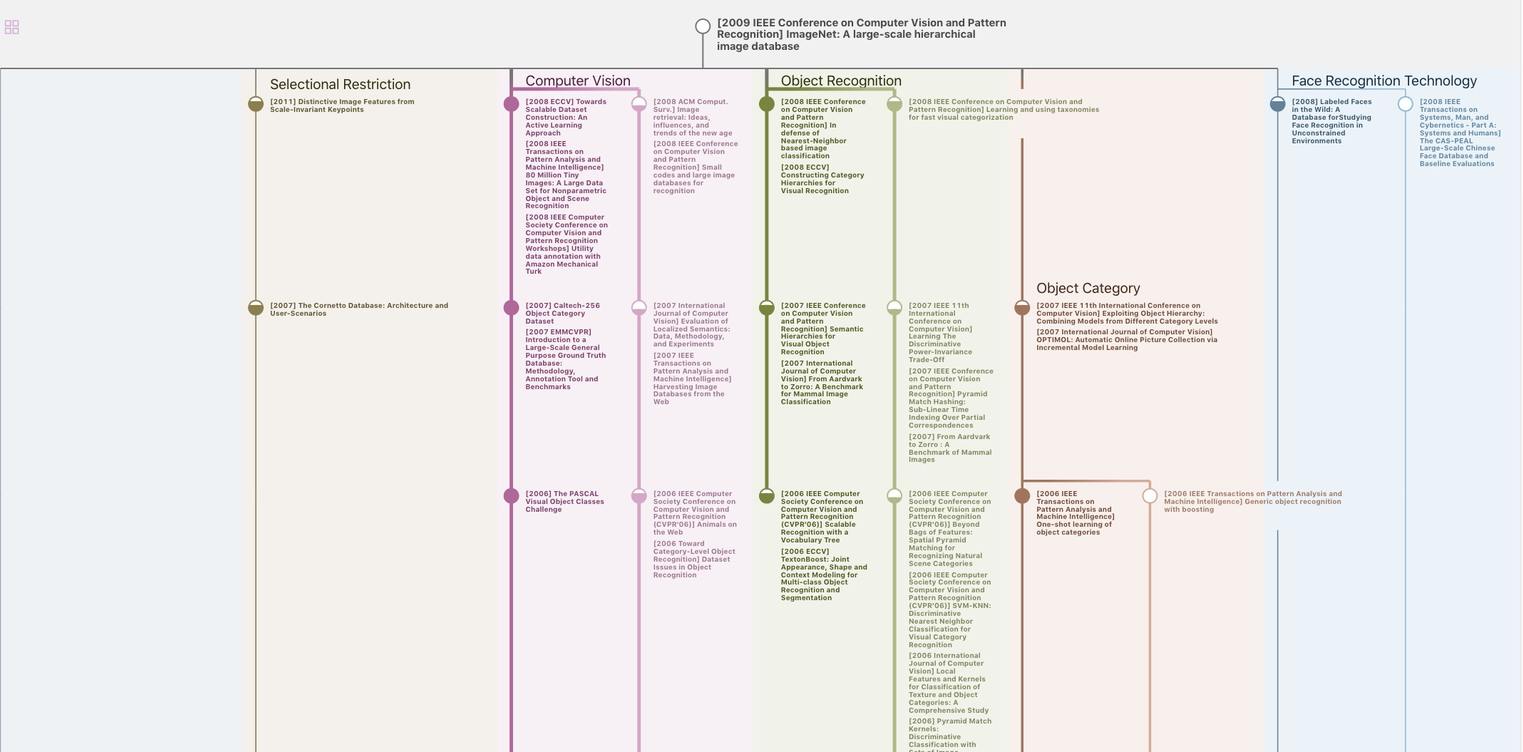
生成溯源树,研究论文发展脉络
Chat Paper
正在生成论文摘要