UnLearnerMC: Unsupervised learning of dense depth and camera pose using mask and multiple consistency losses
Knowledge-Based Systems(2020)
摘要
In this paper, we propose an unsupervised learning framework, named UnLearnerMC, for jointly training monocular depth, camera pose, and segmentation from videos. Existing unsupervised methods typically exploit optical flow consistency to train the segmentation network and eliminate interference from moving objects. Our key point is to create SegMaskNet and corresponding training losses for distinguishing between moving objects and static scenes. Specifically, we eliminate misleading by exploiting boundary masks when moving objects are beyond the view boundary. The SegMaskNet constantly adjusts the allocation of static and moving object pixels via a cooperative loss to minimize the total loss during training. For the moving areas, we can use the re-estimated static depth and pose to eliminate interference from moving areas by using the proposed photometric loop consistency loss. Experiments on KITTI datasets show that UnLearnerMC achieves state-of-the-art results in single-view depth and camera ego-motion, which illustrate the benefits of our approach.
更多查看译文
关键词
Deep learning,Depth estimation,Camera pose,Photometric loop consistency loss,Cooperative loss
AI 理解论文
溯源树
样例
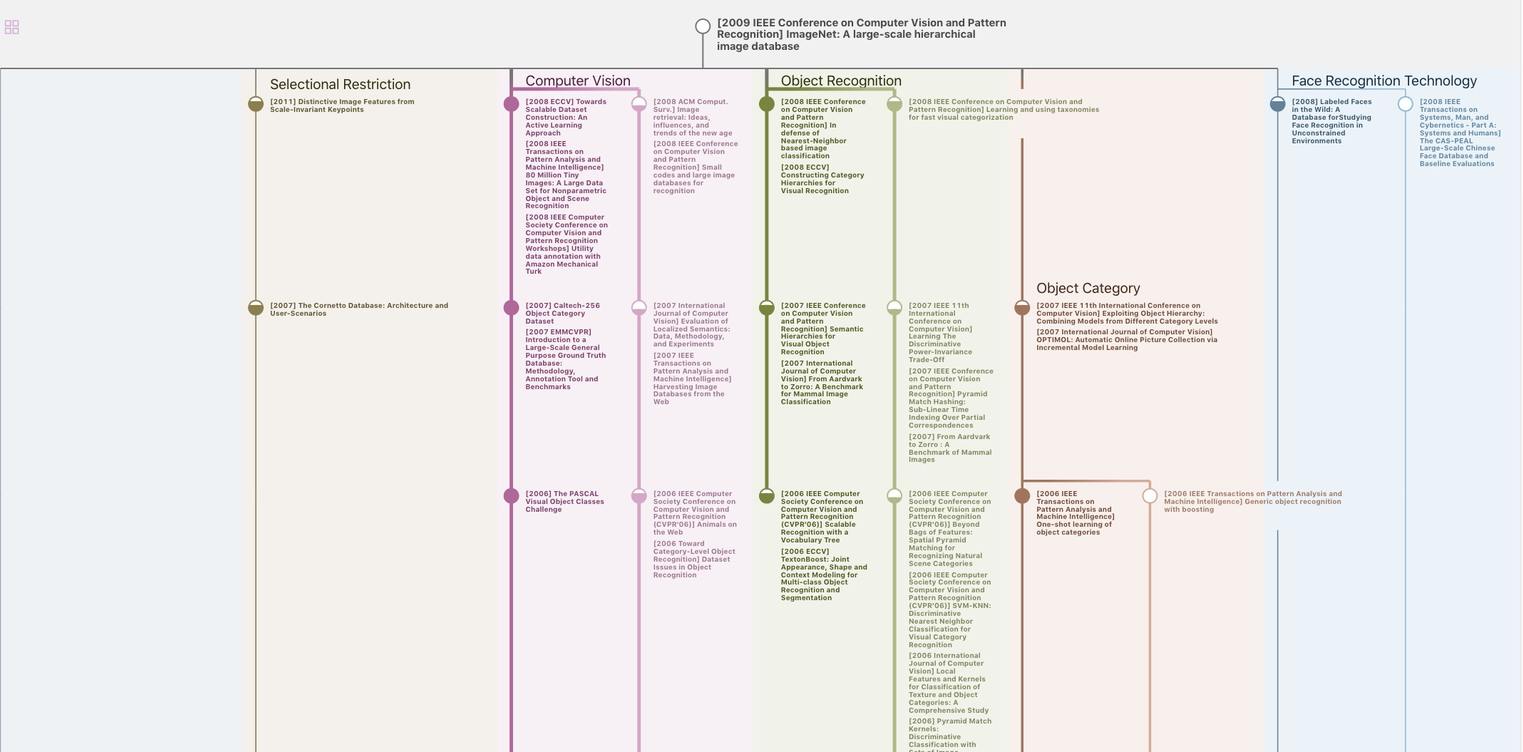
生成溯源树,研究论文发展脉络
Chat Paper
正在生成论文摘要